Convergence in Distribution implies Convergence in Expectation uniformly for an equicontinuous family
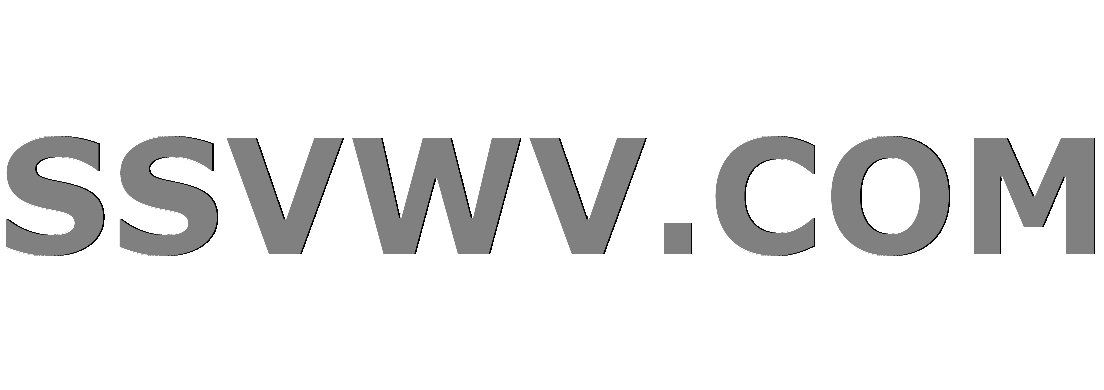
Multi tool use
up vote
1
down vote
favorite
If $X_n xrightarrow{text{d}} X$, then for any equicontinuous family ${f_theta:thetain Theta}$, satisfying sup${|f_theta(x)|:xin mathbf{R},thetain Theta}}<infty$, prove that $E[f_theta(X_n)]rightarrow E[f_theta(X)]$ uniformly in $thetain Theta$.
Now I know if $X_n xrightarrow{text{d}} X$, $E[f_theta(X_n)]rightarrow E[f_theta(X)]hspace{1ex}forall thetainTheta$. And I sense I have to use Arzela-Ascoli Theorem, but I don't have compact set as domain. Any help?
functional-analysis probability-theory measure-theory convergence equicontinuity
add a comment |
up vote
1
down vote
favorite
If $X_n xrightarrow{text{d}} X$, then for any equicontinuous family ${f_theta:thetain Theta}$, satisfying sup${|f_theta(x)|:xin mathbf{R},thetain Theta}}<infty$, prove that $E[f_theta(X_n)]rightarrow E[f_theta(X)]$ uniformly in $thetain Theta$.
Now I know if $X_n xrightarrow{text{d}} X$, $E[f_theta(X_n)]rightarrow E[f_theta(X)]hspace{1ex}forall thetainTheta$. And I sense I have to use Arzela-Ascoli Theorem, but I don't have compact set as domain. Any help?
functional-analysis probability-theory measure-theory convergence equicontinuity
add a comment |
up vote
1
down vote
favorite
up vote
1
down vote
favorite
If $X_n xrightarrow{text{d}} X$, then for any equicontinuous family ${f_theta:thetain Theta}$, satisfying sup${|f_theta(x)|:xin mathbf{R},thetain Theta}}<infty$, prove that $E[f_theta(X_n)]rightarrow E[f_theta(X)]$ uniformly in $thetain Theta$.
Now I know if $X_n xrightarrow{text{d}} X$, $E[f_theta(X_n)]rightarrow E[f_theta(X)]hspace{1ex}forall thetainTheta$. And I sense I have to use Arzela-Ascoli Theorem, but I don't have compact set as domain. Any help?
functional-analysis probability-theory measure-theory convergence equicontinuity
If $X_n xrightarrow{text{d}} X$, then for any equicontinuous family ${f_theta:thetain Theta}$, satisfying sup${|f_theta(x)|:xin mathbf{R},thetain Theta}}<infty$, prove that $E[f_theta(X_n)]rightarrow E[f_theta(X)]$ uniformly in $thetain Theta$.
Now I know if $X_n xrightarrow{text{d}} X$, $E[f_theta(X_n)]rightarrow E[f_theta(X)]hspace{1ex}forall thetainTheta$. And I sense I have to use Arzela-Ascoli Theorem, but I don't have compact set as domain. Any help?
functional-analysis probability-theory measure-theory convergence equicontinuity
functional-analysis probability-theory measure-theory convergence equicontinuity
edited Nov 12 at 6:29
saz
76.3k755117
76.3k755117
asked Nov 11 at 14:09
PSG
1999
1999
add a comment |
add a comment |
1 Answer
1
active
oldest
votes
up vote
1
down vote
accepted
Arzelà-Ascoli is a good idea; in order to apply it we need to truncate the functions $f_{theta}$ in a suitable way.
Step 1: Find suitable level of truncation Fix $epsilon>0$. By the monotone convergence theorem, there exists $R>0$ such that
$$mathbb{P}(|X| geq R) leq epsilon; tag{1}$$
without loss of generality, we may assume that $mathbb{P}(|X|=R)=0$. Since $X_n to X$ weakly we have
$$mathbb{P}(|X_n| geq R) to mathbb{P}(|X| geq R) leq epsilon,$$
and therefore we can choose $N in mathbb{N}$ such that
$$mathbb{P}(|X_n| geq R) leq 2 epsilon quad text{for all $n geq N$.}$$
We can enlarge $R>0$ in such a way that
$$mathbb{P}(|X_j| geq R) leq 2 epsilon quad text{or all $j=1,ldots,N-1$}$$
(note that we only have finitely many $j$'s here!), and therefore we get
$$mathbb{P}(|X_n| geq R) leq 2 epsilon quad text{for all $n geq 1$.} tag{2}$$
Step 2: Apply Arzelà-Ascoli For fixed $epsilon>0$ let $R>0$ be as in Step 1 (i.e. such that $(1)$ and $(2)$ hold). Choose a function $chi in C_b(mathbb{R})$, $0 leq chi leq 1$ such that $chi(x)=1$ for $|x| leq R$ and $chi(x)=$ for $|x| geq R+1$. The family $${chi cdot f_{theta}; theta in Theta}$$ is uniformly bounded and equicontinuous. Moreover, the support of each function $g_{theta} := chi cdot f_{theta}$ is contained in the closed ball of radius $R+1$. By the Arzelà-Ascoli theorem, this implies that the above family is compact in $C_b(mathbb{R})$. In particular, we can choose $theta_1,ldots,theta_m in Theta$ such that $${g_{theta}; theta in Theta} subseteq bigcup_{j=1}^m B(g_{theta_j},epsilon). tag{3}$$ For each $j=1,ldots,m$ there exists $N_j$ such that $$|mathbb{E}g_{theta_j}(X_n)-mathbb{E}g_{theta_j}(X)| leq epsilon quad text{for all $n geq N_j$};$$ set $N := max{N_1,ldots,N_m}$.
Step 3: Prove uniform convergence Fix $theta in Theta$. Clearly, we have
$$|mathbb{E}f_{theta}(X_n)-mathbb{E}f_{theta}(X)| leq I_1+I_2$$
where
$$begin{align*} I_1 &:= |mathbb{E}(f_{theta}(X_n) chi(X_n))- mathbb{E}(f_{theta}(X) chi(X))| \ I_2 &:=|mathbb{E}(f_{theta}(X_n) (1-chi(X_n)))- mathbb{E}(f_{theta}(X) (1-chi(X)))| end{align*}$$
We estimate the terms separately.
Estimate of $I_1$: According to $(3)$ there exists $j in {1,ldots,m}$ such that $|g_{theta}-g_{theta_j}|_{infty} leq epsilon$. Hence, $$I_1 = |mathbb{E}g_{theta}(X_n)-mathbb{E}g_{theta}(X)| leq 2 |g_{theta}-g_{theta_j}|_{infty} + |mathbb{E}g_{theta_j}(X_n)-mathbb{E}g_{theta_j}(X)| leq 3 epsilon tag{4}$$ for all $n geq N$.
Estimate of $I_2$: Since $chi(x)=0$ for all $|x| leq R$ and $0 leq chi leq 1$, we clearly have
$$I_2 leq |f_{theta}|_{infty} left( mathbb{P}(|X_n| geq R) + mathbb{P}(|X| geq R) right).$$
It follows from $(1)$ and $(2)$ that
$$I_2 leq3 |f_{theta}|_{infty} epsilon.$$
If we set $M:= sup_{theta in Theta} |f_{theta}|_{infty}$ (which is finite by assumption) then $$I_2 leq 3 M epsilon.$$
Combining our estimates, we find that
$$|mathbb{E}f_{theta}(X_n)-mathbb{E}f_{theta}(X)| leq 3 (M+1) epsilon$$
for all $n geq N$. Since $epsilon>0$ is arbitrary and $N$ does not depend on $theta$, this proves the uniform convergence with respect to $theta in Theta$: $$sup_{theta in Theta} |mathbb{E}f_{theta}(X_n)-mathbb{E}f_{theta}(X)| xrightarrow{n to infty} 0.$$
Remark: Note that the result implies in particular the following statement.
Theorem If $X_n to X$ in distribution then $$sup_{xi in K} |mathbb{E}exp(i xi X_n)-mathbb{E}exp(i xi X)| xrightarrow{n to infty} 0$$ for any compact set $K$, i.e. the characteristic functions converge uniformly on compact sets.
The family $(exp(itx theta))_{theta in mathbb{R}}$ isn't equicontinuous - only if we restrict $theta$ on compact sets. In particular, the characteristic functions only convergence on compact sets uniformly! For example $delta_{1/n} rightarrow delta_0$, but $|exp(it_n/n) -1| = 2$ for all $t_n = n pi$. Thus the convergence cannot be uniformly!
– p4sch
Nov 12 at 8:43
@p4sch You are right of course, I corrected it; thank you.
– saz
Nov 12 at 9:25
add a comment |
1 Answer
1
active
oldest
votes
1 Answer
1
active
oldest
votes
active
oldest
votes
active
oldest
votes
up vote
1
down vote
accepted
Arzelà-Ascoli is a good idea; in order to apply it we need to truncate the functions $f_{theta}$ in a suitable way.
Step 1: Find suitable level of truncation Fix $epsilon>0$. By the monotone convergence theorem, there exists $R>0$ such that
$$mathbb{P}(|X| geq R) leq epsilon; tag{1}$$
without loss of generality, we may assume that $mathbb{P}(|X|=R)=0$. Since $X_n to X$ weakly we have
$$mathbb{P}(|X_n| geq R) to mathbb{P}(|X| geq R) leq epsilon,$$
and therefore we can choose $N in mathbb{N}$ such that
$$mathbb{P}(|X_n| geq R) leq 2 epsilon quad text{for all $n geq N$.}$$
We can enlarge $R>0$ in such a way that
$$mathbb{P}(|X_j| geq R) leq 2 epsilon quad text{or all $j=1,ldots,N-1$}$$
(note that we only have finitely many $j$'s here!), and therefore we get
$$mathbb{P}(|X_n| geq R) leq 2 epsilon quad text{for all $n geq 1$.} tag{2}$$
Step 2: Apply Arzelà-Ascoli For fixed $epsilon>0$ let $R>0$ be as in Step 1 (i.e. such that $(1)$ and $(2)$ hold). Choose a function $chi in C_b(mathbb{R})$, $0 leq chi leq 1$ such that $chi(x)=1$ for $|x| leq R$ and $chi(x)=$ for $|x| geq R+1$. The family $${chi cdot f_{theta}; theta in Theta}$$ is uniformly bounded and equicontinuous. Moreover, the support of each function $g_{theta} := chi cdot f_{theta}$ is contained in the closed ball of radius $R+1$. By the Arzelà-Ascoli theorem, this implies that the above family is compact in $C_b(mathbb{R})$. In particular, we can choose $theta_1,ldots,theta_m in Theta$ such that $${g_{theta}; theta in Theta} subseteq bigcup_{j=1}^m B(g_{theta_j},epsilon). tag{3}$$ For each $j=1,ldots,m$ there exists $N_j$ such that $$|mathbb{E}g_{theta_j}(X_n)-mathbb{E}g_{theta_j}(X)| leq epsilon quad text{for all $n geq N_j$};$$ set $N := max{N_1,ldots,N_m}$.
Step 3: Prove uniform convergence Fix $theta in Theta$. Clearly, we have
$$|mathbb{E}f_{theta}(X_n)-mathbb{E}f_{theta}(X)| leq I_1+I_2$$
where
$$begin{align*} I_1 &:= |mathbb{E}(f_{theta}(X_n) chi(X_n))- mathbb{E}(f_{theta}(X) chi(X))| \ I_2 &:=|mathbb{E}(f_{theta}(X_n) (1-chi(X_n)))- mathbb{E}(f_{theta}(X) (1-chi(X)))| end{align*}$$
We estimate the terms separately.
Estimate of $I_1$: According to $(3)$ there exists $j in {1,ldots,m}$ such that $|g_{theta}-g_{theta_j}|_{infty} leq epsilon$. Hence, $$I_1 = |mathbb{E}g_{theta}(X_n)-mathbb{E}g_{theta}(X)| leq 2 |g_{theta}-g_{theta_j}|_{infty} + |mathbb{E}g_{theta_j}(X_n)-mathbb{E}g_{theta_j}(X)| leq 3 epsilon tag{4}$$ for all $n geq N$.
Estimate of $I_2$: Since $chi(x)=0$ for all $|x| leq R$ and $0 leq chi leq 1$, we clearly have
$$I_2 leq |f_{theta}|_{infty} left( mathbb{P}(|X_n| geq R) + mathbb{P}(|X| geq R) right).$$
It follows from $(1)$ and $(2)$ that
$$I_2 leq3 |f_{theta}|_{infty} epsilon.$$
If we set $M:= sup_{theta in Theta} |f_{theta}|_{infty}$ (which is finite by assumption) then $$I_2 leq 3 M epsilon.$$
Combining our estimates, we find that
$$|mathbb{E}f_{theta}(X_n)-mathbb{E}f_{theta}(X)| leq 3 (M+1) epsilon$$
for all $n geq N$. Since $epsilon>0$ is arbitrary and $N$ does not depend on $theta$, this proves the uniform convergence with respect to $theta in Theta$: $$sup_{theta in Theta} |mathbb{E}f_{theta}(X_n)-mathbb{E}f_{theta}(X)| xrightarrow{n to infty} 0.$$
Remark: Note that the result implies in particular the following statement.
Theorem If $X_n to X$ in distribution then $$sup_{xi in K} |mathbb{E}exp(i xi X_n)-mathbb{E}exp(i xi X)| xrightarrow{n to infty} 0$$ for any compact set $K$, i.e. the characteristic functions converge uniformly on compact sets.
The family $(exp(itx theta))_{theta in mathbb{R}}$ isn't equicontinuous - only if we restrict $theta$ on compact sets. In particular, the characteristic functions only convergence on compact sets uniformly! For example $delta_{1/n} rightarrow delta_0$, but $|exp(it_n/n) -1| = 2$ for all $t_n = n pi$. Thus the convergence cannot be uniformly!
– p4sch
Nov 12 at 8:43
@p4sch You are right of course, I corrected it; thank you.
– saz
Nov 12 at 9:25
add a comment |
up vote
1
down vote
accepted
Arzelà-Ascoli is a good idea; in order to apply it we need to truncate the functions $f_{theta}$ in a suitable way.
Step 1: Find suitable level of truncation Fix $epsilon>0$. By the monotone convergence theorem, there exists $R>0$ such that
$$mathbb{P}(|X| geq R) leq epsilon; tag{1}$$
without loss of generality, we may assume that $mathbb{P}(|X|=R)=0$. Since $X_n to X$ weakly we have
$$mathbb{P}(|X_n| geq R) to mathbb{P}(|X| geq R) leq epsilon,$$
and therefore we can choose $N in mathbb{N}$ such that
$$mathbb{P}(|X_n| geq R) leq 2 epsilon quad text{for all $n geq N$.}$$
We can enlarge $R>0$ in such a way that
$$mathbb{P}(|X_j| geq R) leq 2 epsilon quad text{or all $j=1,ldots,N-1$}$$
(note that we only have finitely many $j$'s here!), and therefore we get
$$mathbb{P}(|X_n| geq R) leq 2 epsilon quad text{for all $n geq 1$.} tag{2}$$
Step 2: Apply Arzelà-Ascoli For fixed $epsilon>0$ let $R>0$ be as in Step 1 (i.e. such that $(1)$ and $(2)$ hold). Choose a function $chi in C_b(mathbb{R})$, $0 leq chi leq 1$ such that $chi(x)=1$ for $|x| leq R$ and $chi(x)=$ for $|x| geq R+1$. The family $${chi cdot f_{theta}; theta in Theta}$$ is uniformly bounded and equicontinuous. Moreover, the support of each function $g_{theta} := chi cdot f_{theta}$ is contained in the closed ball of radius $R+1$. By the Arzelà-Ascoli theorem, this implies that the above family is compact in $C_b(mathbb{R})$. In particular, we can choose $theta_1,ldots,theta_m in Theta$ such that $${g_{theta}; theta in Theta} subseteq bigcup_{j=1}^m B(g_{theta_j},epsilon). tag{3}$$ For each $j=1,ldots,m$ there exists $N_j$ such that $$|mathbb{E}g_{theta_j}(X_n)-mathbb{E}g_{theta_j}(X)| leq epsilon quad text{for all $n geq N_j$};$$ set $N := max{N_1,ldots,N_m}$.
Step 3: Prove uniform convergence Fix $theta in Theta$. Clearly, we have
$$|mathbb{E}f_{theta}(X_n)-mathbb{E}f_{theta}(X)| leq I_1+I_2$$
where
$$begin{align*} I_1 &:= |mathbb{E}(f_{theta}(X_n) chi(X_n))- mathbb{E}(f_{theta}(X) chi(X))| \ I_2 &:=|mathbb{E}(f_{theta}(X_n) (1-chi(X_n)))- mathbb{E}(f_{theta}(X) (1-chi(X)))| end{align*}$$
We estimate the terms separately.
Estimate of $I_1$: According to $(3)$ there exists $j in {1,ldots,m}$ such that $|g_{theta}-g_{theta_j}|_{infty} leq epsilon$. Hence, $$I_1 = |mathbb{E}g_{theta}(X_n)-mathbb{E}g_{theta}(X)| leq 2 |g_{theta}-g_{theta_j}|_{infty} + |mathbb{E}g_{theta_j}(X_n)-mathbb{E}g_{theta_j}(X)| leq 3 epsilon tag{4}$$ for all $n geq N$.
Estimate of $I_2$: Since $chi(x)=0$ for all $|x| leq R$ and $0 leq chi leq 1$, we clearly have
$$I_2 leq |f_{theta}|_{infty} left( mathbb{P}(|X_n| geq R) + mathbb{P}(|X| geq R) right).$$
It follows from $(1)$ and $(2)$ that
$$I_2 leq3 |f_{theta}|_{infty} epsilon.$$
If we set $M:= sup_{theta in Theta} |f_{theta}|_{infty}$ (which is finite by assumption) then $$I_2 leq 3 M epsilon.$$
Combining our estimates, we find that
$$|mathbb{E}f_{theta}(X_n)-mathbb{E}f_{theta}(X)| leq 3 (M+1) epsilon$$
for all $n geq N$. Since $epsilon>0$ is arbitrary and $N$ does not depend on $theta$, this proves the uniform convergence with respect to $theta in Theta$: $$sup_{theta in Theta} |mathbb{E}f_{theta}(X_n)-mathbb{E}f_{theta}(X)| xrightarrow{n to infty} 0.$$
Remark: Note that the result implies in particular the following statement.
Theorem If $X_n to X$ in distribution then $$sup_{xi in K} |mathbb{E}exp(i xi X_n)-mathbb{E}exp(i xi X)| xrightarrow{n to infty} 0$$ for any compact set $K$, i.e. the characteristic functions converge uniformly on compact sets.
The family $(exp(itx theta))_{theta in mathbb{R}}$ isn't equicontinuous - only if we restrict $theta$ on compact sets. In particular, the characteristic functions only convergence on compact sets uniformly! For example $delta_{1/n} rightarrow delta_0$, but $|exp(it_n/n) -1| = 2$ for all $t_n = n pi$. Thus the convergence cannot be uniformly!
– p4sch
Nov 12 at 8:43
@p4sch You are right of course, I corrected it; thank you.
– saz
Nov 12 at 9:25
add a comment |
up vote
1
down vote
accepted
up vote
1
down vote
accepted
Arzelà-Ascoli is a good idea; in order to apply it we need to truncate the functions $f_{theta}$ in a suitable way.
Step 1: Find suitable level of truncation Fix $epsilon>0$. By the monotone convergence theorem, there exists $R>0$ such that
$$mathbb{P}(|X| geq R) leq epsilon; tag{1}$$
without loss of generality, we may assume that $mathbb{P}(|X|=R)=0$. Since $X_n to X$ weakly we have
$$mathbb{P}(|X_n| geq R) to mathbb{P}(|X| geq R) leq epsilon,$$
and therefore we can choose $N in mathbb{N}$ such that
$$mathbb{P}(|X_n| geq R) leq 2 epsilon quad text{for all $n geq N$.}$$
We can enlarge $R>0$ in such a way that
$$mathbb{P}(|X_j| geq R) leq 2 epsilon quad text{or all $j=1,ldots,N-1$}$$
(note that we only have finitely many $j$'s here!), and therefore we get
$$mathbb{P}(|X_n| geq R) leq 2 epsilon quad text{for all $n geq 1$.} tag{2}$$
Step 2: Apply Arzelà-Ascoli For fixed $epsilon>0$ let $R>0$ be as in Step 1 (i.e. such that $(1)$ and $(2)$ hold). Choose a function $chi in C_b(mathbb{R})$, $0 leq chi leq 1$ such that $chi(x)=1$ for $|x| leq R$ and $chi(x)=$ for $|x| geq R+1$. The family $${chi cdot f_{theta}; theta in Theta}$$ is uniformly bounded and equicontinuous. Moreover, the support of each function $g_{theta} := chi cdot f_{theta}$ is contained in the closed ball of radius $R+1$. By the Arzelà-Ascoli theorem, this implies that the above family is compact in $C_b(mathbb{R})$. In particular, we can choose $theta_1,ldots,theta_m in Theta$ such that $${g_{theta}; theta in Theta} subseteq bigcup_{j=1}^m B(g_{theta_j},epsilon). tag{3}$$ For each $j=1,ldots,m$ there exists $N_j$ such that $$|mathbb{E}g_{theta_j}(X_n)-mathbb{E}g_{theta_j}(X)| leq epsilon quad text{for all $n geq N_j$};$$ set $N := max{N_1,ldots,N_m}$.
Step 3: Prove uniform convergence Fix $theta in Theta$. Clearly, we have
$$|mathbb{E}f_{theta}(X_n)-mathbb{E}f_{theta}(X)| leq I_1+I_2$$
where
$$begin{align*} I_1 &:= |mathbb{E}(f_{theta}(X_n) chi(X_n))- mathbb{E}(f_{theta}(X) chi(X))| \ I_2 &:=|mathbb{E}(f_{theta}(X_n) (1-chi(X_n)))- mathbb{E}(f_{theta}(X) (1-chi(X)))| end{align*}$$
We estimate the terms separately.
Estimate of $I_1$: According to $(3)$ there exists $j in {1,ldots,m}$ such that $|g_{theta}-g_{theta_j}|_{infty} leq epsilon$. Hence, $$I_1 = |mathbb{E}g_{theta}(X_n)-mathbb{E}g_{theta}(X)| leq 2 |g_{theta}-g_{theta_j}|_{infty} + |mathbb{E}g_{theta_j}(X_n)-mathbb{E}g_{theta_j}(X)| leq 3 epsilon tag{4}$$ for all $n geq N$.
Estimate of $I_2$: Since $chi(x)=0$ for all $|x| leq R$ and $0 leq chi leq 1$, we clearly have
$$I_2 leq |f_{theta}|_{infty} left( mathbb{P}(|X_n| geq R) + mathbb{P}(|X| geq R) right).$$
It follows from $(1)$ and $(2)$ that
$$I_2 leq3 |f_{theta}|_{infty} epsilon.$$
If we set $M:= sup_{theta in Theta} |f_{theta}|_{infty}$ (which is finite by assumption) then $$I_2 leq 3 M epsilon.$$
Combining our estimates, we find that
$$|mathbb{E}f_{theta}(X_n)-mathbb{E}f_{theta}(X)| leq 3 (M+1) epsilon$$
for all $n geq N$. Since $epsilon>0$ is arbitrary and $N$ does not depend on $theta$, this proves the uniform convergence with respect to $theta in Theta$: $$sup_{theta in Theta} |mathbb{E}f_{theta}(X_n)-mathbb{E}f_{theta}(X)| xrightarrow{n to infty} 0.$$
Remark: Note that the result implies in particular the following statement.
Theorem If $X_n to X$ in distribution then $$sup_{xi in K} |mathbb{E}exp(i xi X_n)-mathbb{E}exp(i xi X)| xrightarrow{n to infty} 0$$ for any compact set $K$, i.e. the characteristic functions converge uniformly on compact sets.
Arzelà-Ascoli is a good idea; in order to apply it we need to truncate the functions $f_{theta}$ in a suitable way.
Step 1: Find suitable level of truncation Fix $epsilon>0$. By the monotone convergence theorem, there exists $R>0$ such that
$$mathbb{P}(|X| geq R) leq epsilon; tag{1}$$
without loss of generality, we may assume that $mathbb{P}(|X|=R)=0$. Since $X_n to X$ weakly we have
$$mathbb{P}(|X_n| geq R) to mathbb{P}(|X| geq R) leq epsilon,$$
and therefore we can choose $N in mathbb{N}$ such that
$$mathbb{P}(|X_n| geq R) leq 2 epsilon quad text{for all $n geq N$.}$$
We can enlarge $R>0$ in such a way that
$$mathbb{P}(|X_j| geq R) leq 2 epsilon quad text{or all $j=1,ldots,N-1$}$$
(note that we only have finitely many $j$'s here!), and therefore we get
$$mathbb{P}(|X_n| geq R) leq 2 epsilon quad text{for all $n geq 1$.} tag{2}$$
Step 2: Apply Arzelà-Ascoli For fixed $epsilon>0$ let $R>0$ be as in Step 1 (i.e. such that $(1)$ and $(2)$ hold). Choose a function $chi in C_b(mathbb{R})$, $0 leq chi leq 1$ such that $chi(x)=1$ for $|x| leq R$ and $chi(x)=$ for $|x| geq R+1$. The family $${chi cdot f_{theta}; theta in Theta}$$ is uniformly bounded and equicontinuous. Moreover, the support of each function $g_{theta} := chi cdot f_{theta}$ is contained in the closed ball of radius $R+1$. By the Arzelà-Ascoli theorem, this implies that the above family is compact in $C_b(mathbb{R})$. In particular, we can choose $theta_1,ldots,theta_m in Theta$ such that $${g_{theta}; theta in Theta} subseteq bigcup_{j=1}^m B(g_{theta_j},epsilon). tag{3}$$ For each $j=1,ldots,m$ there exists $N_j$ such that $$|mathbb{E}g_{theta_j}(X_n)-mathbb{E}g_{theta_j}(X)| leq epsilon quad text{for all $n geq N_j$};$$ set $N := max{N_1,ldots,N_m}$.
Step 3: Prove uniform convergence Fix $theta in Theta$. Clearly, we have
$$|mathbb{E}f_{theta}(X_n)-mathbb{E}f_{theta}(X)| leq I_1+I_2$$
where
$$begin{align*} I_1 &:= |mathbb{E}(f_{theta}(X_n) chi(X_n))- mathbb{E}(f_{theta}(X) chi(X))| \ I_2 &:=|mathbb{E}(f_{theta}(X_n) (1-chi(X_n)))- mathbb{E}(f_{theta}(X) (1-chi(X)))| end{align*}$$
We estimate the terms separately.
Estimate of $I_1$: According to $(3)$ there exists $j in {1,ldots,m}$ such that $|g_{theta}-g_{theta_j}|_{infty} leq epsilon$. Hence, $$I_1 = |mathbb{E}g_{theta}(X_n)-mathbb{E}g_{theta}(X)| leq 2 |g_{theta}-g_{theta_j}|_{infty} + |mathbb{E}g_{theta_j}(X_n)-mathbb{E}g_{theta_j}(X)| leq 3 epsilon tag{4}$$ for all $n geq N$.
Estimate of $I_2$: Since $chi(x)=0$ for all $|x| leq R$ and $0 leq chi leq 1$, we clearly have
$$I_2 leq |f_{theta}|_{infty} left( mathbb{P}(|X_n| geq R) + mathbb{P}(|X| geq R) right).$$
It follows from $(1)$ and $(2)$ that
$$I_2 leq3 |f_{theta}|_{infty} epsilon.$$
If we set $M:= sup_{theta in Theta} |f_{theta}|_{infty}$ (which is finite by assumption) then $$I_2 leq 3 M epsilon.$$
Combining our estimates, we find that
$$|mathbb{E}f_{theta}(X_n)-mathbb{E}f_{theta}(X)| leq 3 (M+1) epsilon$$
for all $n geq N$. Since $epsilon>0$ is arbitrary and $N$ does not depend on $theta$, this proves the uniform convergence with respect to $theta in Theta$: $$sup_{theta in Theta} |mathbb{E}f_{theta}(X_n)-mathbb{E}f_{theta}(X)| xrightarrow{n to infty} 0.$$
Remark: Note that the result implies in particular the following statement.
Theorem If $X_n to X$ in distribution then $$sup_{xi in K} |mathbb{E}exp(i xi X_n)-mathbb{E}exp(i xi X)| xrightarrow{n to infty} 0$$ for any compact set $K$, i.e. the characteristic functions converge uniformly on compact sets.
edited Nov 14 at 16:06
answered Nov 11 at 18:38
saz
76.3k755117
76.3k755117
The family $(exp(itx theta))_{theta in mathbb{R}}$ isn't equicontinuous - only if we restrict $theta$ on compact sets. In particular, the characteristic functions only convergence on compact sets uniformly! For example $delta_{1/n} rightarrow delta_0$, but $|exp(it_n/n) -1| = 2$ for all $t_n = n pi$. Thus the convergence cannot be uniformly!
– p4sch
Nov 12 at 8:43
@p4sch You are right of course, I corrected it; thank you.
– saz
Nov 12 at 9:25
add a comment |
The family $(exp(itx theta))_{theta in mathbb{R}}$ isn't equicontinuous - only if we restrict $theta$ on compact sets. In particular, the characteristic functions only convergence on compact sets uniformly! For example $delta_{1/n} rightarrow delta_0$, but $|exp(it_n/n) -1| = 2$ for all $t_n = n pi$. Thus the convergence cannot be uniformly!
– p4sch
Nov 12 at 8:43
@p4sch You are right of course, I corrected it; thank you.
– saz
Nov 12 at 9:25
The family $(exp(itx theta))_{theta in mathbb{R}}$ isn't equicontinuous - only if we restrict $theta$ on compact sets. In particular, the characteristic functions only convergence on compact sets uniformly! For example $delta_{1/n} rightarrow delta_0$, but $|exp(it_n/n) -1| = 2$ for all $t_n = n pi$. Thus the convergence cannot be uniformly!
– p4sch
Nov 12 at 8:43
The family $(exp(itx theta))_{theta in mathbb{R}}$ isn't equicontinuous - only if we restrict $theta$ on compact sets. In particular, the characteristic functions only convergence on compact sets uniformly! For example $delta_{1/n} rightarrow delta_0$, but $|exp(it_n/n) -1| = 2$ for all $t_n = n pi$. Thus the convergence cannot be uniformly!
– p4sch
Nov 12 at 8:43
@p4sch You are right of course, I corrected it; thank you.
– saz
Nov 12 at 9:25
@p4sch You are right of course, I corrected it; thank you.
– saz
Nov 12 at 9:25
add a comment |
Sign up or log in
StackExchange.ready(function () {
StackExchange.helpers.onClickDraftSave('#login-link');
});
Sign up using Google
Sign up using Facebook
Sign up using Email and Password
Post as a guest
Required, but never shown
StackExchange.ready(
function () {
StackExchange.openid.initPostLogin('.new-post-login', 'https%3a%2f%2fmath.stackexchange.com%2fquestions%2f2993928%2fconvergence-in-distribution-implies-convergence-in-expectation-uniformly-for-an%23new-answer', 'question_page');
}
);
Post as a guest
Required, but never shown
Sign up or log in
StackExchange.ready(function () {
StackExchange.helpers.onClickDraftSave('#login-link');
});
Sign up using Google
Sign up using Facebook
Sign up using Email and Password
Post as a guest
Required, but never shown
Sign up or log in
StackExchange.ready(function () {
StackExchange.helpers.onClickDraftSave('#login-link');
});
Sign up using Google
Sign up using Facebook
Sign up using Email and Password
Post as a guest
Required, but never shown
Sign up or log in
StackExchange.ready(function () {
StackExchange.helpers.onClickDraftSave('#login-link');
});
Sign up using Google
Sign up using Facebook
Sign up using Email and Password
Sign up using Google
Sign up using Facebook
Sign up using Email and Password
Post as a guest
Required, but never shown
Required, but never shown
Required, but never shown
Required, but never shown
Required, but never shown
Required, but never shown
Required, but never shown
Required, but never shown
Required, but never shown
8Iq,OZKwJCI,4 dUd,ty1O