How does the constraint change when the Lagrange multiplier changes?
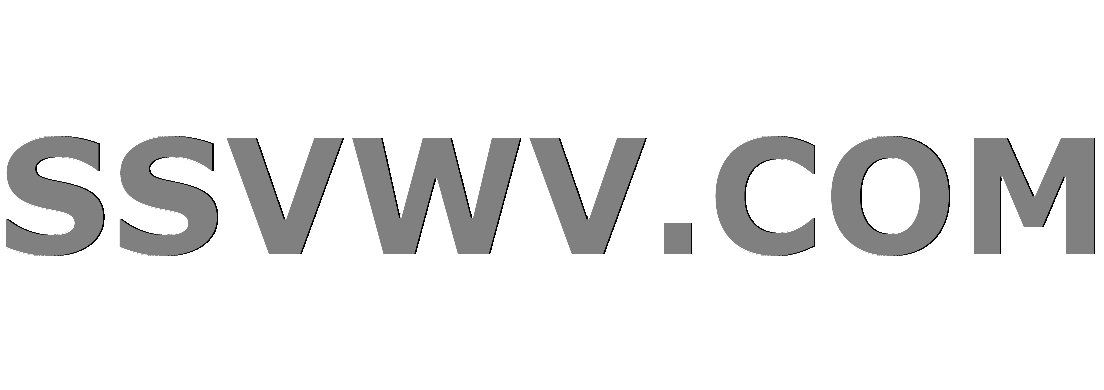
Multi tool use
Now I have a convex function $f(x)$, $xin mathbb{R}^n$, consider the minimization problem: $min_x f(x)+lambda x^Ts$, where $s$ is a positive real vector and $lambda$ is a parameter, I am wondering for different values of $lambda$, when the above min problem reaches its minimization, how does $x^Ts$ changes? Is there any rules?
convex-optimization lagrange-multiplier
add a comment |
Now I have a convex function $f(x)$, $xin mathbb{R}^n$, consider the minimization problem: $min_x f(x)+lambda x^Ts$, where $s$ is a positive real vector and $lambda$ is a parameter, I am wondering for different values of $lambda$, when the above min problem reaches its minimization, how does $x^Ts$ changes? Is there any rules?
convex-optimization lagrange-multiplier
add a comment |
Now I have a convex function $f(x)$, $xin mathbb{R}^n$, consider the minimization problem: $min_x f(x)+lambda x^Ts$, where $s$ is a positive real vector and $lambda$ is a parameter, I am wondering for different values of $lambda$, when the above min problem reaches its minimization, how does $x^Ts$ changes? Is there any rules?
convex-optimization lagrange-multiplier
Now I have a convex function $f(x)$, $xin mathbb{R}^n$, consider the minimization problem: $min_x f(x)+lambda x^Ts$, where $s$ is a positive real vector and $lambda$ is a parameter, I am wondering for different values of $lambda$, when the above min problem reaches its minimization, how does $x^Ts$ changes? Is there any rules?
convex-optimization lagrange-multiplier
convex-optimization lagrange-multiplier
asked Nov 26 at 2:22
Hardy
83
83
add a comment |
add a comment |
1 Answer
1
active
oldest
votes
Given $lambda$ and $s$, you want to find $x in mathbb{R}^n$ to minimize
$$ f(x) + lambda x^Ts $$
Assume at least one minimizer exists for each value of $lambda$ that is considered.
If $lambda_1<lambda_2$ and $x_1$ minimizes for $lambda_1$, $x_2$ minimizes for $lambda_2$, then indeed:
$$x_1^Ts geq x_2^Ts$$
Intuitively, since we penalize the constraint function $x^Ts$ more with the larger $lambda_2$, we get a smaller constraint value $x_2^Ts$. This is easy to prove in 2-3 lines by just using the definition of "minimum," and convexity assumptions are not required. See related and/or more general statements here (Theorem III.2 page 14, Exercises IX-B.5, IX-B.6 page 41): http://ee.usc.edu/stochastic-nets/docs/network-optimization-notes.pdf
More generally if $mathcal{X}subseteq mathbb{R}^n$ is any set (possibly nonconvex and/or disconnected), $f:mathcal{X}rightarrowmathbb{R}$, $g:mathcal{X}rightarrowmathbb{R}$ are any functions (not necessarily convex or continuous) and we consider:
begin{align}
&mbox{Minimize:} quad f(x) + lambda g(x) \
&mbox{Subject to:} quad x in mathcal{X}
end{align}
Then the same holds: If $lambda_1<lambda_2$ and $x_1inmathcal{X}$ and $x_2 in mathcal{X}$ are respective minimizers (assuming minimizers exist), then $g(x_1)geq g(x_2)$. (The proof is the same 2-3 line argument.)
As described in the notes of the above link, this motivates a simple bisection procedure for optimization subject to one constraint, where we zero-in on a good solution (with an optimized $lambda$) exponentially fast (whether or not this bisection procedure always finds a solution, i.e. if there exists a $lambda$ that leads to an optimized $x$ that satisfies the desired constraint, depends on existence of "Hidden/Unhidden" Pareto optimal points, and convexity is then of use for this extended question.)
Thank you! I have come up with the same result and proved it. Thanks for your time anyway!
– Hardy
Nov 27 at 0:33
add a comment |
Your Answer
StackExchange.ifUsing("editor", function () {
return StackExchange.using("mathjaxEditing", function () {
StackExchange.MarkdownEditor.creationCallbacks.add(function (editor, postfix) {
StackExchange.mathjaxEditing.prepareWmdForMathJax(editor, postfix, [["$", "$"], ["\\(","\\)"]]);
});
});
}, "mathjax-editing");
StackExchange.ready(function() {
var channelOptions = {
tags: "".split(" "),
id: "69"
};
initTagRenderer("".split(" "), "".split(" "), channelOptions);
StackExchange.using("externalEditor", function() {
// Have to fire editor after snippets, if snippets enabled
if (StackExchange.settings.snippets.snippetsEnabled) {
StackExchange.using("snippets", function() {
createEditor();
});
}
else {
createEditor();
}
});
function createEditor() {
StackExchange.prepareEditor({
heartbeatType: 'answer',
autoActivateHeartbeat: false,
convertImagesToLinks: true,
noModals: true,
showLowRepImageUploadWarning: true,
reputationToPostImages: 10,
bindNavPrevention: true,
postfix: "",
imageUploader: {
brandingHtml: "Powered by u003ca class="icon-imgur-white" href="https://imgur.com/"u003eu003c/au003e",
contentPolicyHtml: "User contributions licensed under u003ca href="https://creativecommons.org/licenses/by-sa/3.0/"u003ecc by-sa 3.0 with attribution requiredu003c/au003e u003ca href="https://stackoverflow.com/legal/content-policy"u003e(content policy)u003c/au003e",
allowUrls: true
},
noCode: true, onDemand: true,
discardSelector: ".discard-answer"
,immediatelyShowMarkdownHelp:true
});
}
});
Sign up or log in
StackExchange.ready(function () {
StackExchange.helpers.onClickDraftSave('#login-link');
});
Sign up using Google
Sign up using Facebook
Sign up using Email and Password
Post as a guest
Required, but never shown
StackExchange.ready(
function () {
StackExchange.openid.initPostLogin('.new-post-login', 'https%3a%2f%2fmath.stackexchange.com%2fquestions%2f3013725%2fhow-does-the-constraint-change-when-the-lagrange-multiplier-changes%23new-answer', 'question_page');
}
);
Post as a guest
Required, but never shown
1 Answer
1
active
oldest
votes
1 Answer
1
active
oldest
votes
active
oldest
votes
active
oldest
votes
Given $lambda$ and $s$, you want to find $x in mathbb{R}^n$ to minimize
$$ f(x) + lambda x^Ts $$
Assume at least one minimizer exists for each value of $lambda$ that is considered.
If $lambda_1<lambda_2$ and $x_1$ minimizes for $lambda_1$, $x_2$ minimizes for $lambda_2$, then indeed:
$$x_1^Ts geq x_2^Ts$$
Intuitively, since we penalize the constraint function $x^Ts$ more with the larger $lambda_2$, we get a smaller constraint value $x_2^Ts$. This is easy to prove in 2-3 lines by just using the definition of "minimum," and convexity assumptions are not required. See related and/or more general statements here (Theorem III.2 page 14, Exercises IX-B.5, IX-B.6 page 41): http://ee.usc.edu/stochastic-nets/docs/network-optimization-notes.pdf
More generally if $mathcal{X}subseteq mathbb{R}^n$ is any set (possibly nonconvex and/or disconnected), $f:mathcal{X}rightarrowmathbb{R}$, $g:mathcal{X}rightarrowmathbb{R}$ are any functions (not necessarily convex or continuous) and we consider:
begin{align}
&mbox{Minimize:} quad f(x) + lambda g(x) \
&mbox{Subject to:} quad x in mathcal{X}
end{align}
Then the same holds: If $lambda_1<lambda_2$ and $x_1inmathcal{X}$ and $x_2 in mathcal{X}$ are respective minimizers (assuming minimizers exist), then $g(x_1)geq g(x_2)$. (The proof is the same 2-3 line argument.)
As described in the notes of the above link, this motivates a simple bisection procedure for optimization subject to one constraint, where we zero-in on a good solution (with an optimized $lambda$) exponentially fast (whether or not this bisection procedure always finds a solution, i.e. if there exists a $lambda$ that leads to an optimized $x$ that satisfies the desired constraint, depends on existence of "Hidden/Unhidden" Pareto optimal points, and convexity is then of use for this extended question.)
Thank you! I have come up with the same result and proved it. Thanks for your time anyway!
– Hardy
Nov 27 at 0:33
add a comment |
Given $lambda$ and $s$, you want to find $x in mathbb{R}^n$ to minimize
$$ f(x) + lambda x^Ts $$
Assume at least one minimizer exists for each value of $lambda$ that is considered.
If $lambda_1<lambda_2$ and $x_1$ minimizes for $lambda_1$, $x_2$ minimizes for $lambda_2$, then indeed:
$$x_1^Ts geq x_2^Ts$$
Intuitively, since we penalize the constraint function $x^Ts$ more with the larger $lambda_2$, we get a smaller constraint value $x_2^Ts$. This is easy to prove in 2-3 lines by just using the definition of "minimum," and convexity assumptions are not required. See related and/or more general statements here (Theorem III.2 page 14, Exercises IX-B.5, IX-B.6 page 41): http://ee.usc.edu/stochastic-nets/docs/network-optimization-notes.pdf
More generally if $mathcal{X}subseteq mathbb{R}^n$ is any set (possibly nonconvex and/or disconnected), $f:mathcal{X}rightarrowmathbb{R}$, $g:mathcal{X}rightarrowmathbb{R}$ are any functions (not necessarily convex or continuous) and we consider:
begin{align}
&mbox{Minimize:} quad f(x) + lambda g(x) \
&mbox{Subject to:} quad x in mathcal{X}
end{align}
Then the same holds: If $lambda_1<lambda_2$ and $x_1inmathcal{X}$ and $x_2 in mathcal{X}$ are respective minimizers (assuming minimizers exist), then $g(x_1)geq g(x_2)$. (The proof is the same 2-3 line argument.)
As described in the notes of the above link, this motivates a simple bisection procedure for optimization subject to one constraint, where we zero-in on a good solution (with an optimized $lambda$) exponentially fast (whether or not this bisection procedure always finds a solution, i.e. if there exists a $lambda$ that leads to an optimized $x$ that satisfies the desired constraint, depends on existence of "Hidden/Unhidden" Pareto optimal points, and convexity is then of use for this extended question.)
Thank you! I have come up with the same result and proved it. Thanks for your time anyway!
– Hardy
Nov 27 at 0:33
add a comment |
Given $lambda$ and $s$, you want to find $x in mathbb{R}^n$ to minimize
$$ f(x) + lambda x^Ts $$
Assume at least one minimizer exists for each value of $lambda$ that is considered.
If $lambda_1<lambda_2$ and $x_1$ minimizes for $lambda_1$, $x_2$ minimizes for $lambda_2$, then indeed:
$$x_1^Ts geq x_2^Ts$$
Intuitively, since we penalize the constraint function $x^Ts$ more with the larger $lambda_2$, we get a smaller constraint value $x_2^Ts$. This is easy to prove in 2-3 lines by just using the definition of "minimum," and convexity assumptions are not required. See related and/or more general statements here (Theorem III.2 page 14, Exercises IX-B.5, IX-B.6 page 41): http://ee.usc.edu/stochastic-nets/docs/network-optimization-notes.pdf
More generally if $mathcal{X}subseteq mathbb{R}^n$ is any set (possibly nonconvex and/or disconnected), $f:mathcal{X}rightarrowmathbb{R}$, $g:mathcal{X}rightarrowmathbb{R}$ are any functions (not necessarily convex or continuous) and we consider:
begin{align}
&mbox{Minimize:} quad f(x) + lambda g(x) \
&mbox{Subject to:} quad x in mathcal{X}
end{align}
Then the same holds: If $lambda_1<lambda_2$ and $x_1inmathcal{X}$ and $x_2 in mathcal{X}$ are respective minimizers (assuming minimizers exist), then $g(x_1)geq g(x_2)$. (The proof is the same 2-3 line argument.)
As described in the notes of the above link, this motivates a simple bisection procedure for optimization subject to one constraint, where we zero-in on a good solution (with an optimized $lambda$) exponentially fast (whether or not this bisection procedure always finds a solution, i.e. if there exists a $lambda$ that leads to an optimized $x$ that satisfies the desired constraint, depends on existence of "Hidden/Unhidden" Pareto optimal points, and convexity is then of use for this extended question.)
Given $lambda$ and $s$, you want to find $x in mathbb{R}^n$ to minimize
$$ f(x) + lambda x^Ts $$
Assume at least one minimizer exists for each value of $lambda$ that is considered.
If $lambda_1<lambda_2$ and $x_1$ minimizes for $lambda_1$, $x_2$ minimizes for $lambda_2$, then indeed:
$$x_1^Ts geq x_2^Ts$$
Intuitively, since we penalize the constraint function $x^Ts$ more with the larger $lambda_2$, we get a smaller constraint value $x_2^Ts$. This is easy to prove in 2-3 lines by just using the definition of "minimum," and convexity assumptions are not required. See related and/or more general statements here (Theorem III.2 page 14, Exercises IX-B.5, IX-B.6 page 41): http://ee.usc.edu/stochastic-nets/docs/network-optimization-notes.pdf
More generally if $mathcal{X}subseteq mathbb{R}^n$ is any set (possibly nonconvex and/or disconnected), $f:mathcal{X}rightarrowmathbb{R}$, $g:mathcal{X}rightarrowmathbb{R}$ are any functions (not necessarily convex or continuous) and we consider:
begin{align}
&mbox{Minimize:} quad f(x) + lambda g(x) \
&mbox{Subject to:} quad x in mathcal{X}
end{align}
Then the same holds: If $lambda_1<lambda_2$ and $x_1inmathcal{X}$ and $x_2 in mathcal{X}$ are respective minimizers (assuming minimizers exist), then $g(x_1)geq g(x_2)$. (The proof is the same 2-3 line argument.)
As described in the notes of the above link, this motivates a simple bisection procedure for optimization subject to one constraint, where we zero-in on a good solution (with an optimized $lambda$) exponentially fast (whether or not this bisection procedure always finds a solution, i.e. if there exists a $lambda$ that leads to an optimized $x$ that satisfies the desired constraint, depends on existence of "Hidden/Unhidden" Pareto optimal points, and convexity is then of use for this extended question.)
edited Nov 26 at 6:46
answered Nov 26 at 6:39
Michael
13.2k11325
13.2k11325
Thank you! I have come up with the same result and proved it. Thanks for your time anyway!
– Hardy
Nov 27 at 0:33
add a comment |
Thank you! I have come up with the same result and proved it. Thanks for your time anyway!
– Hardy
Nov 27 at 0:33
Thank you! I have come up with the same result and proved it. Thanks for your time anyway!
– Hardy
Nov 27 at 0:33
Thank you! I have come up with the same result and proved it. Thanks for your time anyway!
– Hardy
Nov 27 at 0:33
add a comment |
Thanks for contributing an answer to Mathematics Stack Exchange!
- Please be sure to answer the question. Provide details and share your research!
But avoid …
- Asking for help, clarification, or responding to other answers.
- Making statements based on opinion; back them up with references or personal experience.
Use MathJax to format equations. MathJax reference.
To learn more, see our tips on writing great answers.
Some of your past answers have not been well-received, and you're in danger of being blocked from answering.
Please pay close attention to the following guidance:
- Please be sure to answer the question. Provide details and share your research!
But avoid …
- Asking for help, clarification, or responding to other answers.
- Making statements based on opinion; back them up with references or personal experience.
To learn more, see our tips on writing great answers.
Sign up or log in
StackExchange.ready(function () {
StackExchange.helpers.onClickDraftSave('#login-link');
});
Sign up using Google
Sign up using Facebook
Sign up using Email and Password
Post as a guest
Required, but never shown
StackExchange.ready(
function () {
StackExchange.openid.initPostLogin('.new-post-login', 'https%3a%2f%2fmath.stackexchange.com%2fquestions%2f3013725%2fhow-does-the-constraint-change-when-the-lagrange-multiplier-changes%23new-answer', 'question_page');
}
);
Post as a guest
Required, but never shown
Sign up or log in
StackExchange.ready(function () {
StackExchange.helpers.onClickDraftSave('#login-link');
});
Sign up using Google
Sign up using Facebook
Sign up using Email and Password
Post as a guest
Required, but never shown
Sign up or log in
StackExchange.ready(function () {
StackExchange.helpers.onClickDraftSave('#login-link');
});
Sign up using Google
Sign up using Facebook
Sign up using Email and Password
Post as a guest
Required, but never shown
Sign up or log in
StackExchange.ready(function () {
StackExchange.helpers.onClickDraftSave('#login-link');
});
Sign up using Google
Sign up using Facebook
Sign up using Email and Password
Sign up using Google
Sign up using Facebook
Sign up using Email and Password
Post as a guest
Required, but never shown
Required, but never shown
Required, but never shown
Required, but never shown
Required, but never shown
Required, but never shown
Required, but never shown
Required, but never shown
Required, but never shown
YNviceycQkhFMSV,VFuQG5toY7