Existence of a limiting sum of random variables
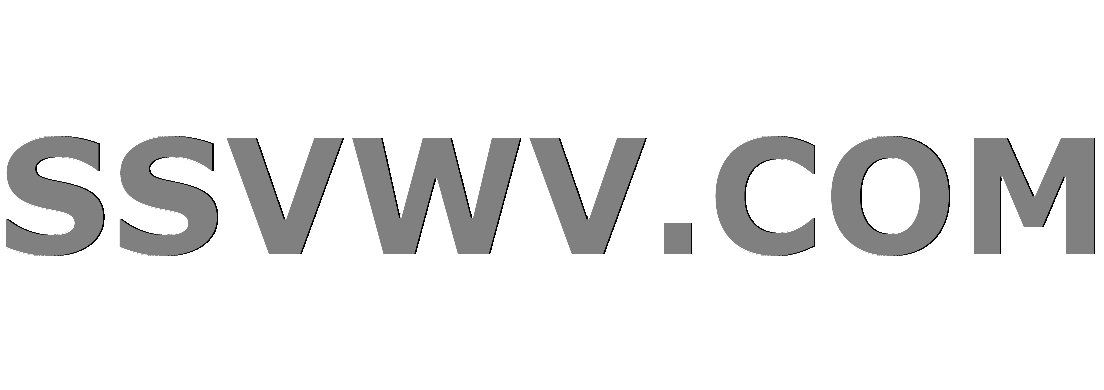
Multi tool use
up vote
1
down vote
favorite
Consider that $X_i$‘s are independent exponentially distributed random variables with mean $1/i$ (and thus variance $1/i^2$).Then the sum of them seems to converge to a “random variable” with finite variance but unbounded mean. What’s the problem here? Why does not such random variable exist?
Thanks
probability statistics random-variables
add a comment |
up vote
1
down vote
favorite
Consider that $X_i$‘s are independent exponentially distributed random variables with mean $1/i$ (and thus variance $1/i^2$).Then the sum of them seems to converge to a “random variable” with finite variance but unbounded mean. What’s the problem here? Why does not such random variable exist?
Thanks
probability statistics random-variables
1
By the same reasoning the "random variable" sequence ${Y_i}_{i=1}^{infty}$ defined by $Y_i=i$ for all $i$ (with prob 1) has partial sums $sum_{i=1}^n Y_i$ with zero variance but mean that goes to infinity. But there is no mystery in this. We certainly do not say that $sum_{i=1}^n i$ "converges" in any sense (it diverges to $infty$). [I put "random variable" in quotes simply because the ${Y_i}$ sequence is deterministic in this case.]
– Michael
Nov 14 at 22:49
add a comment |
up vote
1
down vote
favorite
up vote
1
down vote
favorite
Consider that $X_i$‘s are independent exponentially distributed random variables with mean $1/i$ (and thus variance $1/i^2$).Then the sum of them seems to converge to a “random variable” with finite variance but unbounded mean. What’s the problem here? Why does not such random variable exist?
Thanks
probability statistics random-variables
Consider that $X_i$‘s are independent exponentially distributed random variables with mean $1/i$ (and thus variance $1/i^2$).Then the sum of them seems to converge to a “random variable” with finite variance but unbounded mean. What’s the problem here? Why does not such random variable exist?
Thanks
probability statistics random-variables
probability statistics random-variables
edited Nov 15 at 3:04
asked Nov 14 at 22:35
user49229
144
144
1
By the same reasoning the "random variable" sequence ${Y_i}_{i=1}^{infty}$ defined by $Y_i=i$ for all $i$ (with prob 1) has partial sums $sum_{i=1}^n Y_i$ with zero variance but mean that goes to infinity. But there is no mystery in this. We certainly do not say that $sum_{i=1}^n i$ "converges" in any sense (it diverges to $infty$). [I put "random variable" in quotes simply because the ${Y_i}$ sequence is deterministic in this case.]
– Michael
Nov 14 at 22:49
add a comment |
1
By the same reasoning the "random variable" sequence ${Y_i}_{i=1}^{infty}$ defined by $Y_i=i$ for all $i$ (with prob 1) has partial sums $sum_{i=1}^n Y_i$ with zero variance but mean that goes to infinity. But there is no mystery in this. We certainly do not say that $sum_{i=1}^n i$ "converges" in any sense (it diverges to $infty$). [I put "random variable" in quotes simply because the ${Y_i}$ sequence is deterministic in this case.]
– Michael
Nov 14 at 22:49
1
1
By the same reasoning the "random variable" sequence ${Y_i}_{i=1}^{infty}$ defined by $Y_i=i$ for all $i$ (with prob 1) has partial sums $sum_{i=1}^n Y_i$ with zero variance but mean that goes to infinity. But there is no mystery in this. We certainly do not say that $sum_{i=1}^n i$ "converges" in any sense (it diverges to $infty$). [I put "random variable" in quotes simply because the ${Y_i}$ sequence is deterministic in this case.]
– Michael
Nov 14 at 22:49
By the same reasoning the "random variable" sequence ${Y_i}_{i=1}^{infty}$ defined by $Y_i=i$ for all $i$ (with prob 1) has partial sums $sum_{i=1}^n Y_i$ with zero variance but mean that goes to infinity. But there is no mystery in this. We certainly do not say that $sum_{i=1}^n i$ "converges" in any sense (it diverges to $infty$). [I put "random variable" in quotes simply because the ${Y_i}$ sequence is deterministic in this case.]
– Michael
Nov 14 at 22:49
add a comment |
1 Answer
1
active
oldest
votes
up vote
1
down vote
Since $X_i$'s are positive $sum_{i=1}^{n} X_i$ converges (to a possibly infinte ) sum $X$ and Monotone Convergence Theorem gives $EX=sum_{i=1}^{infty} frac 1 i =infty$. There is no way a random variable with infinite mean can have finite variance. It is not even true that $X <infty$ almost surely. [This can be shown using Laplace transforms].
add a comment |
1 Answer
1
active
oldest
votes
1 Answer
1
active
oldest
votes
active
oldest
votes
active
oldest
votes
up vote
1
down vote
Since $X_i$'s are positive $sum_{i=1}^{n} X_i$ converges (to a possibly infinte ) sum $X$ and Monotone Convergence Theorem gives $EX=sum_{i=1}^{infty} frac 1 i =infty$. There is no way a random variable with infinite mean can have finite variance. It is not even true that $X <infty$ almost surely. [This can be shown using Laplace transforms].
add a comment |
up vote
1
down vote
Since $X_i$'s are positive $sum_{i=1}^{n} X_i$ converges (to a possibly infinte ) sum $X$ and Monotone Convergence Theorem gives $EX=sum_{i=1}^{infty} frac 1 i =infty$. There is no way a random variable with infinite mean can have finite variance. It is not even true that $X <infty$ almost surely. [This can be shown using Laplace transforms].
add a comment |
up vote
1
down vote
up vote
1
down vote
Since $X_i$'s are positive $sum_{i=1}^{n} X_i$ converges (to a possibly infinte ) sum $X$ and Monotone Convergence Theorem gives $EX=sum_{i=1}^{infty} frac 1 i =infty$. There is no way a random variable with infinite mean can have finite variance. It is not even true that $X <infty$ almost surely. [This can be shown using Laplace transforms].
Since $X_i$'s are positive $sum_{i=1}^{n} X_i$ converges (to a possibly infinte ) sum $X$ and Monotone Convergence Theorem gives $EX=sum_{i=1}^{infty} frac 1 i =infty$. There is no way a random variable with infinite mean can have finite variance. It is not even true that $X <infty$ almost surely. [This can be shown using Laplace transforms].
edited Nov 14 at 23:35
answered Nov 14 at 23:30


Kavi Rama Murthy
41.3k31751
41.3k31751
add a comment |
add a comment |
Sign up or log in
StackExchange.ready(function () {
StackExchange.helpers.onClickDraftSave('#login-link');
});
Sign up using Google
Sign up using Facebook
Sign up using Email and Password
Post as a guest
Required, but never shown
StackExchange.ready(
function () {
StackExchange.openid.initPostLogin('.new-post-login', 'https%3a%2f%2fmath.stackexchange.com%2fquestions%2f2998915%2fexistence-of-a-limiting-sum-of-random-variables%23new-answer', 'question_page');
}
);
Post as a guest
Required, but never shown
Sign up or log in
StackExchange.ready(function () {
StackExchange.helpers.onClickDraftSave('#login-link');
});
Sign up using Google
Sign up using Facebook
Sign up using Email and Password
Post as a guest
Required, but never shown
Sign up or log in
StackExchange.ready(function () {
StackExchange.helpers.onClickDraftSave('#login-link');
});
Sign up using Google
Sign up using Facebook
Sign up using Email and Password
Post as a guest
Required, but never shown
Sign up or log in
StackExchange.ready(function () {
StackExchange.helpers.onClickDraftSave('#login-link');
});
Sign up using Google
Sign up using Facebook
Sign up using Email and Password
Sign up using Google
Sign up using Facebook
Sign up using Email and Password
Post as a guest
Required, but never shown
Required, but never shown
Required, but never shown
Required, but never shown
Required, but never shown
Required, but never shown
Required, but never shown
Required, but never shown
Required, but never shown
vj1ShUJQqLCC6yGkT4VqZdt xqs9p3PAFboGez,5LAj,3s1FTOhu,vj,E c0O1SF2ZISJ,MOJ U9kRkuzE5icLdY1
1
By the same reasoning the "random variable" sequence ${Y_i}_{i=1}^{infty}$ defined by $Y_i=i$ for all $i$ (with prob 1) has partial sums $sum_{i=1}^n Y_i$ with zero variance but mean that goes to infinity. But there is no mystery in this. We certainly do not say that $sum_{i=1}^n i$ "converges" in any sense (it diverges to $infty$). [I put "random variable" in quotes simply because the ${Y_i}$ sequence is deterministic in this case.]
– Michael
Nov 14 at 22:49