How to Simplify Yule Walker Expression for AR Coefficients?
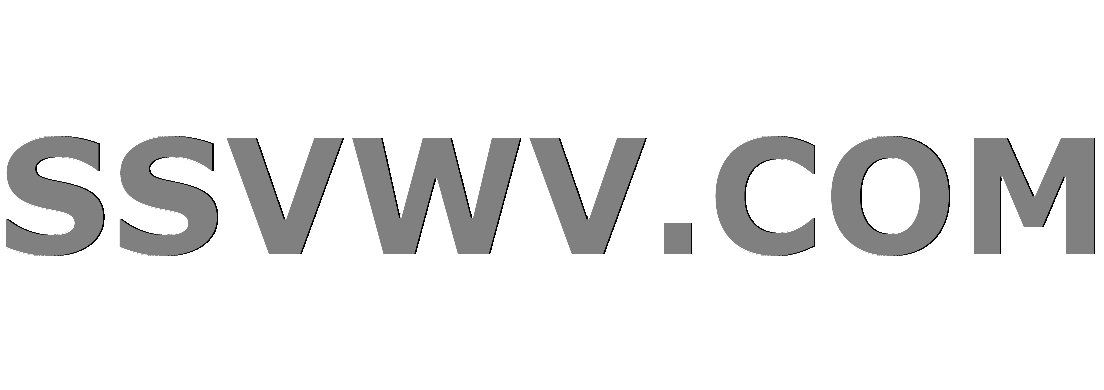
Multi tool use
$begingroup$
We have an AR(2) process (w/ intercept omitted):
$$
y _ { t } = a _ { 1 } y _ { t - 1 } + a _ { 2 } y _ { t - 2 } + varepsilon _ { t }
$$
We multiply the second order equation by $ y_{t-s} text{ for } s = 0, s = 1, s = 2 ldots $
$$
begin{aligned} E y _ { t } y _ { t } & = a _ { 1 } E y _ { t - 1 } y _ { t } + a _ { 2 } E y _ { t - 2 } y _ { t } + E varepsilon _ { t } y _ { t } \ E y _ { t } y _ { t - 1 } & = a _ { 1 } E y _ { t - 1 } y _ { t - 1 } + a _ { 2 } E y _ { t - 2 } y _ { t - 1 } + E varepsilon _ { t } y _ { t - 1 } \ E y _ { t } y _ { t - 2 } & = a _ { 1 } E y _ { t - 1 } y _ { t - 2 } + a _ { 2 } E y _ { t - 2 } y _ { t - 2 } + E varepsilon _ { t } y _ { t - 2 } \ cdots & \ & cdots \ E y _ { t } y _ { t - s } & = a _ { 1 } E y _ { t - 1 } y _ { t - s } + a _ { 2 } E y _ { t - 2 } y _ { t - s } + E varepsilon _ { t } y _ { t - s } end{aligned}
$$
By definition, the autocovariances of a stationary series are such that $E y _ { t } y _ { t - s } =$
$E y _ { t - s } y _ { t } = E y _ { t - k } y _ { t - k - s } = gamma _ { s } .$ We also know that $E varepsilon _ { t } y _ { t } = sigma ^ { 2 }$ and $E varepsilon _ { t } y _ { t - s } = 0 .$ Hence, we
can use the equations in $( 2.24 )$ to form
$$
gamma _ { 0 } = a _ { 1 } gamma _ { 1 } + a _ { 2 } gamma _ { 2 } + sigma ^ { 2 }
$$
$$
begin{aligned} gamma _ { 1 } & = a _ { 1 } gamma _ { 0 } + a _ { 2 } gamma _ { 1 } \ gamma _ { s } & = a _ { 1 } gamma _ { s - 1 } + a _ { 2 } gamma _ { s - 2 } \ text { Dividing by } gamma _ { 0 } text { yields } \ rho _ { 1 } & = a _ { 1 } rho _ { 0 } + a _ { 2 } rho _ { 1 } \ rho _ { s } & = a _ { 1 } rho _ { s - 1 } + a _ { 2 } rho _ { s - 2 } end{aligned}
$$
Question: How did $ gamma_1 / gamma_0 $ turn into the $rho_1$ function?
The simple algebra of this division isn't making sense. What happens to the Variance $ sigma^2 $ that's in $ gamma_0 $ but somehow eliminated in the $ gamma_1$ function?
sequences-and-series functions stochastic-processes systems-of-equations
$endgroup$
|
show 2 more comments
$begingroup$
We have an AR(2) process (w/ intercept omitted):
$$
y _ { t } = a _ { 1 } y _ { t - 1 } + a _ { 2 } y _ { t - 2 } + varepsilon _ { t }
$$
We multiply the second order equation by $ y_{t-s} text{ for } s = 0, s = 1, s = 2 ldots $
$$
begin{aligned} E y _ { t } y _ { t } & = a _ { 1 } E y _ { t - 1 } y _ { t } + a _ { 2 } E y _ { t - 2 } y _ { t } + E varepsilon _ { t } y _ { t } \ E y _ { t } y _ { t - 1 } & = a _ { 1 } E y _ { t - 1 } y _ { t - 1 } + a _ { 2 } E y _ { t - 2 } y _ { t - 1 } + E varepsilon _ { t } y _ { t - 1 } \ E y _ { t } y _ { t - 2 } & = a _ { 1 } E y _ { t - 1 } y _ { t - 2 } + a _ { 2 } E y _ { t - 2 } y _ { t - 2 } + E varepsilon _ { t } y _ { t - 2 } \ cdots & \ & cdots \ E y _ { t } y _ { t - s } & = a _ { 1 } E y _ { t - 1 } y _ { t - s } + a _ { 2 } E y _ { t - 2 } y _ { t - s } + E varepsilon _ { t } y _ { t - s } end{aligned}
$$
By definition, the autocovariances of a stationary series are such that $E y _ { t } y _ { t - s } =$
$E y _ { t - s } y _ { t } = E y _ { t - k } y _ { t - k - s } = gamma _ { s } .$ We also know that $E varepsilon _ { t } y _ { t } = sigma ^ { 2 }$ and $E varepsilon _ { t } y _ { t - s } = 0 .$ Hence, we
can use the equations in $( 2.24 )$ to form
$$
gamma _ { 0 } = a _ { 1 } gamma _ { 1 } + a _ { 2 } gamma _ { 2 } + sigma ^ { 2 }
$$
$$
begin{aligned} gamma _ { 1 } & = a _ { 1 } gamma _ { 0 } + a _ { 2 } gamma _ { 1 } \ gamma _ { s } & = a _ { 1 } gamma _ { s - 1 } + a _ { 2 } gamma _ { s - 2 } \ text { Dividing by } gamma _ { 0 } text { yields } \ rho _ { 1 } & = a _ { 1 } rho _ { 0 } + a _ { 2 } rho _ { 1 } \ rho _ { s } & = a _ { 1 } rho _ { s - 1 } + a _ { 2 } rho _ { s - 2 } end{aligned}
$$
Question: How did $ gamma_1 / gamma_0 $ turn into the $rho_1$ function?
The simple algebra of this division isn't making sense. What happens to the Variance $ sigma^2 $ that's in $ gamma_0 $ but somehow eliminated in the $ gamma_1$ function?
sequences-and-series functions stochastic-processes systems-of-equations
$endgroup$
$begingroup$
You forgot the 1st line with $gamma 0$
$endgroup$
– Damien
Dec 24 '18 at 17:01
$begingroup$
@Damien I think it's there? Just above the line for $ gamma_{1} $ and centered. Maybe I'm misunderstanding?
$endgroup$
– AIS91
Dec 24 '18 at 17:05
$begingroup$
Yes this line. Don't forget it!
$endgroup$
– Damien
Dec 24 '18 at 17:32
$begingroup$
I realized the first small caveat that helps me solve the problem. $E varepsilon _ { t } y _ { t } = sigma^2 $ but this has to be 0 to eliminate serial correlation.
$endgroup$
– AIS91
Dec 24 '18 at 17:49
$begingroup$
What do you mean with eliminate ? This term is very inportant ...
$endgroup$
– Damien
Dec 24 '18 at 18:18
|
show 2 more comments
$begingroup$
We have an AR(2) process (w/ intercept omitted):
$$
y _ { t } = a _ { 1 } y _ { t - 1 } + a _ { 2 } y _ { t - 2 } + varepsilon _ { t }
$$
We multiply the second order equation by $ y_{t-s} text{ for } s = 0, s = 1, s = 2 ldots $
$$
begin{aligned} E y _ { t } y _ { t } & = a _ { 1 } E y _ { t - 1 } y _ { t } + a _ { 2 } E y _ { t - 2 } y _ { t } + E varepsilon _ { t } y _ { t } \ E y _ { t } y _ { t - 1 } & = a _ { 1 } E y _ { t - 1 } y _ { t - 1 } + a _ { 2 } E y _ { t - 2 } y _ { t - 1 } + E varepsilon _ { t } y _ { t - 1 } \ E y _ { t } y _ { t - 2 } & = a _ { 1 } E y _ { t - 1 } y _ { t - 2 } + a _ { 2 } E y _ { t - 2 } y _ { t - 2 } + E varepsilon _ { t } y _ { t - 2 } \ cdots & \ & cdots \ E y _ { t } y _ { t - s } & = a _ { 1 } E y _ { t - 1 } y _ { t - s } + a _ { 2 } E y _ { t - 2 } y _ { t - s } + E varepsilon _ { t } y _ { t - s } end{aligned}
$$
By definition, the autocovariances of a stationary series are such that $E y _ { t } y _ { t - s } =$
$E y _ { t - s } y _ { t } = E y _ { t - k } y _ { t - k - s } = gamma _ { s } .$ We also know that $E varepsilon _ { t } y _ { t } = sigma ^ { 2 }$ and $E varepsilon _ { t } y _ { t - s } = 0 .$ Hence, we
can use the equations in $( 2.24 )$ to form
$$
gamma _ { 0 } = a _ { 1 } gamma _ { 1 } + a _ { 2 } gamma _ { 2 } + sigma ^ { 2 }
$$
$$
begin{aligned} gamma _ { 1 } & = a _ { 1 } gamma _ { 0 } + a _ { 2 } gamma _ { 1 } \ gamma _ { s } & = a _ { 1 } gamma _ { s - 1 } + a _ { 2 } gamma _ { s - 2 } \ text { Dividing by } gamma _ { 0 } text { yields } \ rho _ { 1 } & = a _ { 1 } rho _ { 0 } + a _ { 2 } rho _ { 1 } \ rho _ { s } & = a _ { 1 } rho _ { s - 1 } + a _ { 2 } rho _ { s - 2 } end{aligned}
$$
Question: How did $ gamma_1 / gamma_0 $ turn into the $rho_1$ function?
The simple algebra of this division isn't making sense. What happens to the Variance $ sigma^2 $ that's in $ gamma_0 $ but somehow eliminated in the $ gamma_1$ function?
sequences-and-series functions stochastic-processes systems-of-equations
$endgroup$
We have an AR(2) process (w/ intercept omitted):
$$
y _ { t } = a _ { 1 } y _ { t - 1 } + a _ { 2 } y _ { t - 2 } + varepsilon _ { t }
$$
We multiply the second order equation by $ y_{t-s} text{ for } s = 0, s = 1, s = 2 ldots $
$$
begin{aligned} E y _ { t } y _ { t } & = a _ { 1 } E y _ { t - 1 } y _ { t } + a _ { 2 } E y _ { t - 2 } y _ { t } + E varepsilon _ { t } y _ { t } \ E y _ { t } y _ { t - 1 } & = a _ { 1 } E y _ { t - 1 } y _ { t - 1 } + a _ { 2 } E y _ { t - 2 } y _ { t - 1 } + E varepsilon _ { t } y _ { t - 1 } \ E y _ { t } y _ { t - 2 } & = a _ { 1 } E y _ { t - 1 } y _ { t - 2 } + a _ { 2 } E y _ { t - 2 } y _ { t - 2 } + E varepsilon _ { t } y _ { t - 2 } \ cdots & \ & cdots \ E y _ { t } y _ { t - s } & = a _ { 1 } E y _ { t - 1 } y _ { t - s } + a _ { 2 } E y _ { t - 2 } y _ { t - s } + E varepsilon _ { t } y _ { t - s } end{aligned}
$$
By definition, the autocovariances of a stationary series are such that $E y _ { t } y _ { t - s } =$
$E y _ { t - s } y _ { t } = E y _ { t - k } y _ { t - k - s } = gamma _ { s } .$ We also know that $E varepsilon _ { t } y _ { t } = sigma ^ { 2 }$ and $E varepsilon _ { t } y _ { t - s } = 0 .$ Hence, we
can use the equations in $( 2.24 )$ to form
$$
gamma _ { 0 } = a _ { 1 } gamma _ { 1 } + a _ { 2 } gamma _ { 2 } + sigma ^ { 2 }
$$
$$
begin{aligned} gamma _ { 1 } & = a _ { 1 } gamma _ { 0 } + a _ { 2 } gamma _ { 1 } \ gamma _ { s } & = a _ { 1 } gamma _ { s - 1 } + a _ { 2 } gamma _ { s - 2 } \ text { Dividing by } gamma _ { 0 } text { yields } \ rho _ { 1 } & = a _ { 1 } rho _ { 0 } + a _ { 2 } rho _ { 1 } \ rho _ { s } & = a _ { 1 } rho _ { s - 1 } + a _ { 2 } rho _ { s - 2 } end{aligned}
$$
Question: How did $ gamma_1 / gamma_0 $ turn into the $rho_1$ function?
The simple algebra of this division isn't making sense. What happens to the Variance $ sigma^2 $ that's in $ gamma_0 $ but somehow eliminated in the $ gamma_1$ function?
sequences-and-series functions stochastic-processes systems-of-equations
sequences-and-series functions stochastic-processes systems-of-equations
asked Dec 24 '18 at 16:49


AIS91AIS91
183
183
$begingroup$
You forgot the 1st line with $gamma 0$
$endgroup$
– Damien
Dec 24 '18 at 17:01
$begingroup$
@Damien I think it's there? Just above the line for $ gamma_{1} $ and centered. Maybe I'm misunderstanding?
$endgroup$
– AIS91
Dec 24 '18 at 17:05
$begingroup$
Yes this line. Don't forget it!
$endgroup$
– Damien
Dec 24 '18 at 17:32
$begingroup$
I realized the first small caveat that helps me solve the problem. $E varepsilon _ { t } y _ { t } = sigma^2 $ but this has to be 0 to eliminate serial correlation.
$endgroup$
– AIS91
Dec 24 '18 at 17:49
$begingroup$
What do you mean with eliminate ? This term is very inportant ...
$endgroup$
– Damien
Dec 24 '18 at 18:18
|
show 2 more comments
$begingroup$
You forgot the 1st line with $gamma 0$
$endgroup$
– Damien
Dec 24 '18 at 17:01
$begingroup$
@Damien I think it's there? Just above the line for $ gamma_{1} $ and centered. Maybe I'm misunderstanding?
$endgroup$
– AIS91
Dec 24 '18 at 17:05
$begingroup$
Yes this line. Don't forget it!
$endgroup$
– Damien
Dec 24 '18 at 17:32
$begingroup$
I realized the first small caveat that helps me solve the problem. $E varepsilon _ { t } y _ { t } = sigma^2 $ but this has to be 0 to eliminate serial correlation.
$endgroup$
– AIS91
Dec 24 '18 at 17:49
$begingroup$
What do you mean with eliminate ? This term is very inportant ...
$endgroup$
– Damien
Dec 24 '18 at 18:18
$begingroup$
You forgot the 1st line with $gamma 0$
$endgroup$
– Damien
Dec 24 '18 at 17:01
$begingroup$
You forgot the 1st line with $gamma 0$
$endgroup$
– Damien
Dec 24 '18 at 17:01
$begingroup$
@Damien I think it's there? Just above the line for $ gamma_{1} $ and centered. Maybe I'm misunderstanding?
$endgroup$
– AIS91
Dec 24 '18 at 17:05
$begingroup$
@Damien I think it's there? Just above the line for $ gamma_{1} $ and centered. Maybe I'm misunderstanding?
$endgroup$
– AIS91
Dec 24 '18 at 17:05
$begingroup$
Yes this line. Don't forget it!
$endgroup$
– Damien
Dec 24 '18 at 17:32
$begingroup$
Yes this line. Don't forget it!
$endgroup$
– Damien
Dec 24 '18 at 17:32
$begingroup$
I realized the first small caveat that helps me solve the problem. $E varepsilon _ { t } y _ { t } = sigma^2 $ but this has to be 0 to eliminate serial correlation.
$endgroup$
– AIS91
Dec 24 '18 at 17:49
$begingroup$
I realized the first small caveat that helps me solve the problem. $E varepsilon _ { t } y _ { t } = sigma^2 $ but this has to be 0 to eliminate serial correlation.
$endgroup$
– AIS91
Dec 24 '18 at 17:49
$begingroup$
What do you mean with eliminate ? This term is very inportant ...
$endgroup$
– Damien
Dec 24 '18 at 18:18
$begingroup$
What do you mean with eliminate ? This term is very inportant ...
$endgroup$
– Damien
Dec 24 '18 at 18:18
|
show 2 more comments
1 Answer
1
active
oldest
votes
$begingroup$
Suppose we have an AR$(2)$ process
$$X_t=frac{1}{3}X_{t-1}+frac{1}{2}X_{t-2}+Z_t$$
From properties of the lag operator:
$$L(B)=1-frac{1}{3}B-frac{1}{2}B^2$$
This has real roots, both are outside the unit circle. This implies this process is weakly stationary.
Remark: A stochastic process is said to be weakly stationary when its mean and autocovariance does not vary with respect to time. Also,
the second moment is finite at all times. For more details see this
Next multiply both sides by $X_{t-k}$ and take expectation to obtain $E(X_{t-k}X_t)=gamma(k)$. (now the part you are interested in). Since $mu=0$, assuming $gamma(k)=0$, then:
$$gamma(-k)=frac{1}{3}gamma(-k+1)+frac{1}{2}gamma(-k+2)$$
Here note $gamma(k)=gamma(-k)$ for any $k$.
$$gamma(k)=frac{1}{3}gamma(k-1)+frac{1}{2}gamma(k-2)$$
Dividing both sides by $gamma(0)=sigma^2$ gives:
$$rho(k)=frac{1}{3}rho(k-1)+frac{1}{2}rho(k-2)$$
Notice $Var(X)=(E(X))^2-E(XY)$. See the above assumption and for stationary time series $E(X)=mu=0$. The definition of autocorrelation is given here.
The example is from the course by the state university of New York
$endgroup$
$begingroup$
Thanks a ton for this! Are you assuming this process is white noise? Hence why you're able to define $mu = frac { 1 } { 3 } mu + frac { 1 } { 2 } mu$ as being 0? Or is this an assumption for stationarity? I would think the former?
$endgroup$
– AIS91
Dec 26 '18 at 21:13
$begingroup$
No, see the update.
$endgroup$
– Waqas
Dec 26 '18 at 21:22
$begingroup$
$$ begin{array} { l } { E left( y _ { t } right) = E left( y _ { t - s } right) = mu } \ { E left[ left( y _ { t } - mu right) ^ { 2 } right] = E left[ left( y _ { t - s } - mu right) ^ { 2 } right] = sigma _ { y } ^ { 2 } } end{array} $$ and $$ E left[ left( y _ { t } - mu right) left( y _ { t - s } - mu right) right] = E left[ left( y _ { t - j } - mu right) left( y _ { t - j - s } - mu right) right] = gamma _ { s } $$. Where $ mu, sigma^2, gamma_s $ constant. But how does that make $ mu = 0 $ from your equation?
$endgroup$
– AIS91
Dec 26 '18 at 21:28
1
$begingroup$
Yes, here $epsilon_t$ is indeed white noise. Upvote :)
$endgroup$
– Waqas
Dec 26 '18 at 21:42
1
$begingroup$
When you consider $gamma(k)=E(X_{t-k},X_t)$ for the given time series you get $gamma(-k)$, which is equal to $gamma(k)$. There is a lot of material available on the web regarding Time series modelling; you may find that helpful.
$endgroup$
– Waqas
Dec 27 '18 at 22:23
|
show 4 more comments
Your Answer
StackExchange.ifUsing("editor", function () {
return StackExchange.using("mathjaxEditing", function () {
StackExchange.MarkdownEditor.creationCallbacks.add(function (editor, postfix) {
StackExchange.mathjaxEditing.prepareWmdForMathJax(editor, postfix, [["$", "$"], ["\\(","\\)"]]);
});
});
}, "mathjax-editing");
StackExchange.ready(function() {
var channelOptions = {
tags: "".split(" "),
id: "69"
};
initTagRenderer("".split(" "), "".split(" "), channelOptions);
StackExchange.using("externalEditor", function() {
// Have to fire editor after snippets, if snippets enabled
if (StackExchange.settings.snippets.snippetsEnabled) {
StackExchange.using("snippets", function() {
createEditor();
});
}
else {
createEditor();
}
});
function createEditor() {
StackExchange.prepareEditor({
heartbeatType: 'answer',
autoActivateHeartbeat: false,
convertImagesToLinks: true,
noModals: true,
showLowRepImageUploadWarning: true,
reputationToPostImages: 10,
bindNavPrevention: true,
postfix: "",
imageUploader: {
brandingHtml: "Powered by u003ca class="icon-imgur-white" href="https://imgur.com/"u003eu003c/au003e",
contentPolicyHtml: "User contributions licensed under u003ca href="https://creativecommons.org/licenses/by-sa/3.0/"u003ecc by-sa 3.0 with attribution requiredu003c/au003e u003ca href="https://stackoverflow.com/legal/content-policy"u003e(content policy)u003c/au003e",
allowUrls: true
},
noCode: true, onDemand: true,
discardSelector: ".discard-answer"
,immediatelyShowMarkdownHelp:true
});
}
});
Sign up or log in
StackExchange.ready(function () {
StackExchange.helpers.onClickDraftSave('#login-link');
});
Sign up using Google
Sign up using Facebook
Sign up using Email and Password
Post as a guest
Required, but never shown
StackExchange.ready(
function () {
StackExchange.openid.initPostLogin('.new-post-login', 'https%3a%2f%2fmath.stackexchange.com%2fquestions%2f3051444%2fhow-to-simplify-yule-walker-expression-for-ar-coefficients%23new-answer', 'question_page');
}
);
Post as a guest
Required, but never shown
1 Answer
1
active
oldest
votes
1 Answer
1
active
oldest
votes
active
oldest
votes
active
oldest
votes
$begingroup$
Suppose we have an AR$(2)$ process
$$X_t=frac{1}{3}X_{t-1}+frac{1}{2}X_{t-2}+Z_t$$
From properties of the lag operator:
$$L(B)=1-frac{1}{3}B-frac{1}{2}B^2$$
This has real roots, both are outside the unit circle. This implies this process is weakly stationary.
Remark: A stochastic process is said to be weakly stationary when its mean and autocovariance does not vary with respect to time. Also,
the second moment is finite at all times. For more details see this
Next multiply both sides by $X_{t-k}$ and take expectation to obtain $E(X_{t-k}X_t)=gamma(k)$. (now the part you are interested in). Since $mu=0$, assuming $gamma(k)=0$, then:
$$gamma(-k)=frac{1}{3}gamma(-k+1)+frac{1}{2}gamma(-k+2)$$
Here note $gamma(k)=gamma(-k)$ for any $k$.
$$gamma(k)=frac{1}{3}gamma(k-1)+frac{1}{2}gamma(k-2)$$
Dividing both sides by $gamma(0)=sigma^2$ gives:
$$rho(k)=frac{1}{3}rho(k-1)+frac{1}{2}rho(k-2)$$
Notice $Var(X)=(E(X))^2-E(XY)$. See the above assumption and for stationary time series $E(X)=mu=0$. The definition of autocorrelation is given here.
The example is from the course by the state university of New York
$endgroup$
$begingroup$
Thanks a ton for this! Are you assuming this process is white noise? Hence why you're able to define $mu = frac { 1 } { 3 } mu + frac { 1 } { 2 } mu$ as being 0? Or is this an assumption for stationarity? I would think the former?
$endgroup$
– AIS91
Dec 26 '18 at 21:13
$begingroup$
No, see the update.
$endgroup$
– Waqas
Dec 26 '18 at 21:22
$begingroup$
$$ begin{array} { l } { E left( y _ { t } right) = E left( y _ { t - s } right) = mu } \ { E left[ left( y _ { t } - mu right) ^ { 2 } right] = E left[ left( y _ { t - s } - mu right) ^ { 2 } right] = sigma _ { y } ^ { 2 } } end{array} $$ and $$ E left[ left( y _ { t } - mu right) left( y _ { t - s } - mu right) right] = E left[ left( y _ { t - j } - mu right) left( y _ { t - j - s } - mu right) right] = gamma _ { s } $$. Where $ mu, sigma^2, gamma_s $ constant. But how does that make $ mu = 0 $ from your equation?
$endgroup$
– AIS91
Dec 26 '18 at 21:28
1
$begingroup$
Yes, here $epsilon_t$ is indeed white noise. Upvote :)
$endgroup$
– Waqas
Dec 26 '18 at 21:42
1
$begingroup$
When you consider $gamma(k)=E(X_{t-k},X_t)$ for the given time series you get $gamma(-k)$, which is equal to $gamma(k)$. There is a lot of material available on the web regarding Time series modelling; you may find that helpful.
$endgroup$
– Waqas
Dec 27 '18 at 22:23
|
show 4 more comments
$begingroup$
Suppose we have an AR$(2)$ process
$$X_t=frac{1}{3}X_{t-1}+frac{1}{2}X_{t-2}+Z_t$$
From properties of the lag operator:
$$L(B)=1-frac{1}{3}B-frac{1}{2}B^2$$
This has real roots, both are outside the unit circle. This implies this process is weakly stationary.
Remark: A stochastic process is said to be weakly stationary when its mean and autocovariance does not vary with respect to time. Also,
the second moment is finite at all times. For more details see this
Next multiply both sides by $X_{t-k}$ and take expectation to obtain $E(X_{t-k}X_t)=gamma(k)$. (now the part you are interested in). Since $mu=0$, assuming $gamma(k)=0$, then:
$$gamma(-k)=frac{1}{3}gamma(-k+1)+frac{1}{2}gamma(-k+2)$$
Here note $gamma(k)=gamma(-k)$ for any $k$.
$$gamma(k)=frac{1}{3}gamma(k-1)+frac{1}{2}gamma(k-2)$$
Dividing both sides by $gamma(0)=sigma^2$ gives:
$$rho(k)=frac{1}{3}rho(k-1)+frac{1}{2}rho(k-2)$$
Notice $Var(X)=(E(X))^2-E(XY)$. See the above assumption and for stationary time series $E(X)=mu=0$. The definition of autocorrelation is given here.
The example is from the course by the state university of New York
$endgroup$
$begingroup$
Thanks a ton for this! Are you assuming this process is white noise? Hence why you're able to define $mu = frac { 1 } { 3 } mu + frac { 1 } { 2 } mu$ as being 0? Or is this an assumption for stationarity? I would think the former?
$endgroup$
– AIS91
Dec 26 '18 at 21:13
$begingroup$
No, see the update.
$endgroup$
– Waqas
Dec 26 '18 at 21:22
$begingroup$
$$ begin{array} { l } { E left( y _ { t } right) = E left( y _ { t - s } right) = mu } \ { E left[ left( y _ { t } - mu right) ^ { 2 } right] = E left[ left( y _ { t - s } - mu right) ^ { 2 } right] = sigma _ { y } ^ { 2 } } end{array} $$ and $$ E left[ left( y _ { t } - mu right) left( y _ { t - s } - mu right) right] = E left[ left( y _ { t - j } - mu right) left( y _ { t - j - s } - mu right) right] = gamma _ { s } $$. Where $ mu, sigma^2, gamma_s $ constant. But how does that make $ mu = 0 $ from your equation?
$endgroup$
– AIS91
Dec 26 '18 at 21:28
1
$begingroup$
Yes, here $epsilon_t$ is indeed white noise. Upvote :)
$endgroup$
– Waqas
Dec 26 '18 at 21:42
1
$begingroup$
When you consider $gamma(k)=E(X_{t-k},X_t)$ for the given time series you get $gamma(-k)$, which is equal to $gamma(k)$. There is a lot of material available on the web regarding Time series modelling; you may find that helpful.
$endgroup$
– Waqas
Dec 27 '18 at 22:23
|
show 4 more comments
$begingroup$
Suppose we have an AR$(2)$ process
$$X_t=frac{1}{3}X_{t-1}+frac{1}{2}X_{t-2}+Z_t$$
From properties of the lag operator:
$$L(B)=1-frac{1}{3}B-frac{1}{2}B^2$$
This has real roots, both are outside the unit circle. This implies this process is weakly stationary.
Remark: A stochastic process is said to be weakly stationary when its mean and autocovariance does not vary with respect to time. Also,
the second moment is finite at all times. For more details see this
Next multiply both sides by $X_{t-k}$ and take expectation to obtain $E(X_{t-k}X_t)=gamma(k)$. (now the part you are interested in). Since $mu=0$, assuming $gamma(k)=0$, then:
$$gamma(-k)=frac{1}{3}gamma(-k+1)+frac{1}{2}gamma(-k+2)$$
Here note $gamma(k)=gamma(-k)$ for any $k$.
$$gamma(k)=frac{1}{3}gamma(k-1)+frac{1}{2}gamma(k-2)$$
Dividing both sides by $gamma(0)=sigma^2$ gives:
$$rho(k)=frac{1}{3}rho(k-1)+frac{1}{2}rho(k-2)$$
Notice $Var(X)=(E(X))^2-E(XY)$. See the above assumption and for stationary time series $E(X)=mu=0$. The definition of autocorrelation is given here.
The example is from the course by the state university of New York
$endgroup$
Suppose we have an AR$(2)$ process
$$X_t=frac{1}{3}X_{t-1}+frac{1}{2}X_{t-2}+Z_t$$
From properties of the lag operator:
$$L(B)=1-frac{1}{3}B-frac{1}{2}B^2$$
This has real roots, both are outside the unit circle. This implies this process is weakly stationary.
Remark: A stochastic process is said to be weakly stationary when its mean and autocovariance does not vary with respect to time. Also,
the second moment is finite at all times. For more details see this
Next multiply both sides by $X_{t-k}$ and take expectation to obtain $E(X_{t-k}X_t)=gamma(k)$. (now the part you are interested in). Since $mu=0$, assuming $gamma(k)=0$, then:
$$gamma(-k)=frac{1}{3}gamma(-k+1)+frac{1}{2}gamma(-k+2)$$
Here note $gamma(k)=gamma(-k)$ for any $k$.
$$gamma(k)=frac{1}{3}gamma(k-1)+frac{1}{2}gamma(k-2)$$
Dividing both sides by $gamma(0)=sigma^2$ gives:
$$rho(k)=frac{1}{3}rho(k-1)+frac{1}{2}rho(k-2)$$
Notice $Var(X)=(E(X))^2-E(XY)$. See the above assumption and for stationary time series $E(X)=mu=0$. The definition of autocorrelation is given here.
The example is from the course by the state university of New York
edited Dec 26 '18 at 21:39
answered Dec 25 '18 at 11:27


WaqasWaqas
15913
15913
$begingroup$
Thanks a ton for this! Are you assuming this process is white noise? Hence why you're able to define $mu = frac { 1 } { 3 } mu + frac { 1 } { 2 } mu$ as being 0? Or is this an assumption for stationarity? I would think the former?
$endgroup$
– AIS91
Dec 26 '18 at 21:13
$begingroup$
No, see the update.
$endgroup$
– Waqas
Dec 26 '18 at 21:22
$begingroup$
$$ begin{array} { l } { E left( y _ { t } right) = E left( y _ { t - s } right) = mu } \ { E left[ left( y _ { t } - mu right) ^ { 2 } right] = E left[ left( y _ { t - s } - mu right) ^ { 2 } right] = sigma _ { y } ^ { 2 } } end{array} $$ and $$ E left[ left( y _ { t } - mu right) left( y _ { t - s } - mu right) right] = E left[ left( y _ { t - j } - mu right) left( y _ { t - j - s } - mu right) right] = gamma _ { s } $$. Where $ mu, sigma^2, gamma_s $ constant. But how does that make $ mu = 0 $ from your equation?
$endgroup$
– AIS91
Dec 26 '18 at 21:28
1
$begingroup$
Yes, here $epsilon_t$ is indeed white noise. Upvote :)
$endgroup$
– Waqas
Dec 26 '18 at 21:42
1
$begingroup$
When you consider $gamma(k)=E(X_{t-k},X_t)$ for the given time series you get $gamma(-k)$, which is equal to $gamma(k)$. There is a lot of material available on the web regarding Time series modelling; you may find that helpful.
$endgroup$
– Waqas
Dec 27 '18 at 22:23
|
show 4 more comments
$begingroup$
Thanks a ton for this! Are you assuming this process is white noise? Hence why you're able to define $mu = frac { 1 } { 3 } mu + frac { 1 } { 2 } mu$ as being 0? Or is this an assumption for stationarity? I would think the former?
$endgroup$
– AIS91
Dec 26 '18 at 21:13
$begingroup$
No, see the update.
$endgroup$
– Waqas
Dec 26 '18 at 21:22
$begingroup$
$$ begin{array} { l } { E left( y _ { t } right) = E left( y _ { t - s } right) = mu } \ { E left[ left( y _ { t } - mu right) ^ { 2 } right] = E left[ left( y _ { t - s } - mu right) ^ { 2 } right] = sigma _ { y } ^ { 2 } } end{array} $$ and $$ E left[ left( y _ { t } - mu right) left( y _ { t - s } - mu right) right] = E left[ left( y _ { t - j } - mu right) left( y _ { t - j - s } - mu right) right] = gamma _ { s } $$. Where $ mu, sigma^2, gamma_s $ constant. But how does that make $ mu = 0 $ from your equation?
$endgroup$
– AIS91
Dec 26 '18 at 21:28
1
$begingroup$
Yes, here $epsilon_t$ is indeed white noise. Upvote :)
$endgroup$
– Waqas
Dec 26 '18 at 21:42
1
$begingroup$
When you consider $gamma(k)=E(X_{t-k},X_t)$ for the given time series you get $gamma(-k)$, which is equal to $gamma(k)$. There is a lot of material available on the web regarding Time series modelling; you may find that helpful.
$endgroup$
– Waqas
Dec 27 '18 at 22:23
$begingroup$
Thanks a ton for this! Are you assuming this process is white noise? Hence why you're able to define $mu = frac { 1 } { 3 } mu + frac { 1 } { 2 } mu$ as being 0? Or is this an assumption for stationarity? I would think the former?
$endgroup$
– AIS91
Dec 26 '18 at 21:13
$begingroup$
Thanks a ton for this! Are you assuming this process is white noise? Hence why you're able to define $mu = frac { 1 } { 3 } mu + frac { 1 } { 2 } mu$ as being 0? Or is this an assumption for stationarity? I would think the former?
$endgroup$
– AIS91
Dec 26 '18 at 21:13
$begingroup$
No, see the update.
$endgroup$
– Waqas
Dec 26 '18 at 21:22
$begingroup$
No, see the update.
$endgroup$
– Waqas
Dec 26 '18 at 21:22
$begingroup$
$$ begin{array} { l } { E left( y _ { t } right) = E left( y _ { t - s } right) = mu } \ { E left[ left( y _ { t } - mu right) ^ { 2 } right] = E left[ left( y _ { t - s } - mu right) ^ { 2 } right] = sigma _ { y } ^ { 2 } } end{array} $$ and $$ E left[ left( y _ { t } - mu right) left( y _ { t - s } - mu right) right] = E left[ left( y _ { t - j } - mu right) left( y _ { t - j - s } - mu right) right] = gamma _ { s } $$. Where $ mu, sigma^2, gamma_s $ constant. But how does that make $ mu = 0 $ from your equation?
$endgroup$
– AIS91
Dec 26 '18 at 21:28
$begingroup$
$$ begin{array} { l } { E left( y _ { t } right) = E left( y _ { t - s } right) = mu } \ { E left[ left( y _ { t } - mu right) ^ { 2 } right] = E left[ left( y _ { t - s } - mu right) ^ { 2 } right] = sigma _ { y } ^ { 2 } } end{array} $$ and $$ E left[ left( y _ { t } - mu right) left( y _ { t - s } - mu right) right] = E left[ left( y _ { t - j } - mu right) left( y _ { t - j - s } - mu right) right] = gamma _ { s } $$. Where $ mu, sigma^2, gamma_s $ constant. But how does that make $ mu = 0 $ from your equation?
$endgroup$
– AIS91
Dec 26 '18 at 21:28
1
1
$begingroup$
Yes, here $epsilon_t$ is indeed white noise. Upvote :)
$endgroup$
– Waqas
Dec 26 '18 at 21:42
$begingroup$
Yes, here $epsilon_t$ is indeed white noise. Upvote :)
$endgroup$
– Waqas
Dec 26 '18 at 21:42
1
1
$begingroup$
When you consider $gamma(k)=E(X_{t-k},X_t)$ for the given time series you get $gamma(-k)$, which is equal to $gamma(k)$. There is a lot of material available on the web regarding Time series modelling; you may find that helpful.
$endgroup$
– Waqas
Dec 27 '18 at 22:23
$begingroup$
When you consider $gamma(k)=E(X_{t-k},X_t)$ for the given time series you get $gamma(-k)$, which is equal to $gamma(k)$. There is a lot of material available on the web regarding Time series modelling; you may find that helpful.
$endgroup$
– Waqas
Dec 27 '18 at 22:23
|
show 4 more comments
Thanks for contributing an answer to Mathematics Stack Exchange!
- Please be sure to answer the question. Provide details and share your research!
But avoid …
- Asking for help, clarification, or responding to other answers.
- Making statements based on opinion; back them up with references or personal experience.
Use MathJax to format equations. MathJax reference.
To learn more, see our tips on writing great answers.
Sign up or log in
StackExchange.ready(function () {
StackExchange.helpers.onClickDraftSave('#login-link');
});
Sign up using Google
Sign up using Facebook
Sign up using Email and Password
Post as a guest
Required, but never shown
StackExchange.ready(
function () {
StackExchange.openid.initPostLogin('.new-post-login', 'https%3a%2f%2fmath.stackexchange.com%2fquestions%2f3051444%2fhow-to-simplify-yule-walker-expression-for-ar-coefficients%23new-answer', 'question_page');
}
);
Post as a guest
Required, but never shown
Sign up or log in
StackExchange.ready(function () {
StackExchange.helpers.onClickDraftSave('#login-link');
});
Sign up using Google
Sign up using Facebook
Sign up using Email and Password
Post as a guest
Required, but never shown
Sign up or log in
StackExchange.ready(function () {
StackExchange.helpers.onClickDraftSave('#login-link');
});
Sign up using Google
Sign up using Facebook
Sign up using Email and Password
Post as a guest
Required, but never shown
Sign up or log in
StackExchange.ready(function () {
StackExchange.helpers.onClickDraftSave('#login-link');
});
Sign up using Google
Sign up using Facebook
Sign up using Email and Password
Sign up using Google
Sign up using Facebook
Sign up using Email and Password
Post as a guest
Required, but never shown
Required, but never shown
Required, but never shown
Required, but never shown
Required, but never shown
Required, but never shown
Required, but never shown
Required, but never shown
Required, but never shown
3 ONxjbVqFIB dXIEVFE8lR K4 5yZG24l1,nHQbNExnLIYpd2iqBkGs9E,Gp
$begingroup$
You forgot the 1st line with $gamma 0$
$endgroup$
– Damien
Dec 24 '18 at 17:01
$begingroup$
@Damien I think it's there? Just above the line for $ gamma_{1} $ and centered. Maybe I'm misunderstanding?
$endgroup$
– AIS91
Dec 24 '18 at 17:05
$begingroup$
Yes this line. Don't forget it!
$endgroup$
– Damien
Dec 24 '18 at 17:32
$begingroup$
I realized the first small caveat that helps me solve the problem. $E varepsilon _ { t } y _ { t } = sigma^2 $ but this has to be 0 to eliminate serial correlation.
$endgroup$
– AIS91
Dec 24 '18 at 17:49
$begingroup$
What do you mean with eliminate ? This term is very inportant ...
$endgroup$
– Damien
Dec 24 '18 at 18:18