What are the foundations of probability and how are they dependent upon a $sigma$-field?
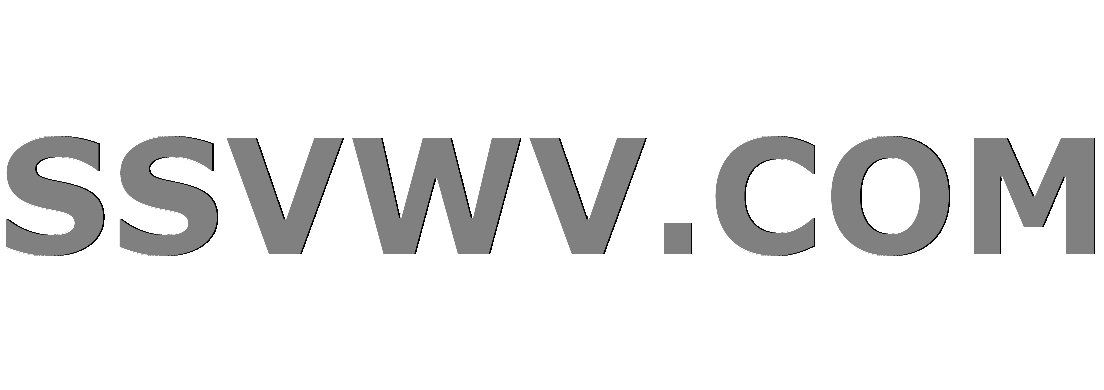
Multi tool use
I am reading Christopher D. Manning's Foundations of Statistical Natural Language Processing which gives an introduction on Probability Theory where it talks about $sigma$-fields. It says,
The foundations of probability theory depend on the set of events $mathscr{F}$ forming a $sigma$-field".
I understand the definition of a $sigma$-field, but what are these foundations of probability theory, and how are these foundations dependent upon a $sigma$-field?
probability-theory measure-theory
add a comment |
I am reading Christopher D. Manning's Foundations of Statistical Natural Language Processing which gives an introduction on Probability Theory where it talks about $sigma$-fields. It says,
The foundations of probability theory depend on the set of events $mathscr{F}$ forming a $sigma$-field".
I understand the definition of a $sigma$-field, but what are these foundations of probability theory, and how are these foundations dependent upon a $sigma$-field?
probability-theory measure-theory
5
But this is not a textbook on probability theory. This is a book on Natural Language Processing which includes some preliminaries of probability theory -- this is not the same thing. If you want to understand the foundations of probability, consult a textbook on that, not on something that merely uses probabilities.
– Clement C.
Dec 16 at 0:48
1
@eddard Some people are concerned with the scope of your question being too broad ("foundations of probability theory" is a topic of considerable size). Would you mind editing the post to be only about the second bullet point?
– Lord_Farin
Dec 16 at 22:00
2
@Lord_Farin someone tried to focus it and improve the title. Then someone didn't like it and rolled it back. Not sure why, since that would make this a good question.
– Don Hatch
Dec 18 at 8:09
add a comment |
I am reading Christopher D. Manning's Foundations of Statistical Natural Language Processing which gives an introduction on Probability Theory where it talks about $sigma$-fields. It says,
The foundations of probability theory depend on the set of events $mathscr{F}$ forming a $sigma$-field".
I understand the definition of a $sigma$-field, but what are these foundations of probability theory, and how are these foundations dependent upon a $sigma$-field?
probability-theory measure-theory
I am reading Christopher D. Manning's Foundations of Statistical Natural Language Processing which gives an introduction on Probability Theory where it talks about $sigma$-fields. It says,
The foundations of probability theory depend on the set of events $mathscr{F}$ forming a $sigma$-field".
I understand the definition of a $sigma$-field, but what are these foundations of probability theory, and how are these foundations dependent upon a $sigma$-field?
probability-theory measure-theory
probability-theory measure-theory
edited Dec 18 at 9:25


Mike Pierce
11.4k103583
11.4k103583
asked Dec 15 at 23:03
eddard.stark
20914
20914
5
But this is not a textbook on probability theory. This is a book on Natural Language Processing which includes some preliminaries of probability theory -- this is not the same thing. If you want to understand the foundations of probability, consult a textbook on that, not on something that merely uses probabilities.
– Clement C.
Dec 16 at 0:48
1
@eddard Some people are concerned with the scope of your question being too broad ("foundations of probability theory" is a topic of considerable size). Would you mind editing the post to be only about the second bullet point?
– Lord_Farin
Dec 16 at 22:00
2
@Lord_Farin someone tried to focus it and improve the title. Then someone didn't like it and rolled it back. Not sure why, since that would make this a good question.
– Don Hatch
Dec 18 at 8:09
add a comment |
5
But this is not a textbook on probability theory. This is a book on Natural Language Processing which includes some preliminaries of probability theory -- this is not the same thing. If you want to understand the foundations of probability, consult a textbook on that, not on something that merely uses probabilities.
– Clement C.
Dec 16 at 0:48
1
@eddard Some people are concerned with the scope of your question being too broad ("foundations of probability theory" is a topic of considerable size). Would you mind editing the post to be only about the second bullet point?
– Lord_Farin
Dec 16 at 22:00
2
@Lord_Farin someone tried to focus it and improve the title. Then someone didn't like it and rolled it back. Not sure why, since that would make this a good question.
– Don Hatch
Dec 18 at 8:09
5
5
But this is not a textbook on probability theory. This is a book on Natural Language Processing which includes some preliminaries of probability theory -- this is not the same thing. If you want to understand the foundations of probability, consult a textbook on that, not on something that merely uses probabilities.
– Clement C.
Dec 16 at 0:48
But this is not a textbook on probability theory. This is a book on Natural Language Processing which includes some preliminaries of probability theory -- this is not the same thing. If you want to understand the foundations of probability, consult a textbook on that, not on something that merely uses probabilities.
– Clement C.
Dec 16 at 0:48
1
1
@eddard Some people are concerned with the scope of your question being too broad ("foundations of probability theory" is a topic of considerable size). Would you mind editing the post to be only about the second bullet point?
– Lord_Farin
Dec 16 at 22:00
@eddard Some people are concerned with the scope of your question being too broad ("foundations of probability theory" is a topic of considerable size). Would you mind editing the post to be only about the second bullet point?
– Lord_Farin
Dec 16 at 22:00
2
2
@Lord_Farin someone tried to focus it and improve the title. Then someone didn't like it and rolled it back. Not sure why, since that would make this a good question.
– Don Hatch
Dec 18 at 8:09
@Lord_Farin someone tried to focus it and improve the title. Then someone didn't like it and rolled it back. Not sure why, since that would make this a good question.
– Don Hatch
Dec 18 at 8:09
add a comment |
2 Answers
2
active
oldest
votes
Probability when there are only finitely many outcomes is a matter of counting. There are $36$ possible results from a roll of two dice and $6$ of them sum to $7$ so the probability of a sum of $7$ is $6/36$. You've measured the size of the set of outcomes that you are interested in.
It's harder to make rigorous sense of things when the set of possible results is infinite. What does it mean to choose two numbers at random in the interval $[1,6]$ and ask for their sum? Any particular pair, like $(1.3, pi)$, will have probability $0$.
You deal with this problem by replacing counting with integration. Unfortunately, the integration you learn in first year calculus ("Riemann integration") isn't powerful enough to derive all you need about probability. (It is enough to determine the probability that your two rolls total exactly $7$ is $0$, and to find the probability that it's at least $7$.)
For the definitions and theorems of rigorous probability theory (those are the "foundations" you ask about) you need "Lebesgue integration". That requires first carefully specifying the sets that you are going to ask for the probabilities of - and not every set is allowed, for technical reasons without which you can't make the mathematics work the way you want. It turns out that the set of sets whose probability you are going to ask about carries the name "$sigma$-field" or "sigma algebra". (It's not a field in the arithmetic sense.)
The essential point is that it's closed under countable set operations. That's what the "$sigma$" says. Your text may not provide a formal definition - you may not need it for NLP applications.
I am reading it a couple of times. I think it is going to take me some time to understand all of it. Thanks for the answer anyways.
– eddard.stark
Dec 16 at 1:43
1
I find the following answer related and useful Why do we need sigma-algebras to define probability spaces
– Jesper Hybel
Dec 24 at 15:18
"Probability when there are only finitely many outcomes is a matter of counting." This is a rather dangerous simplification since it assumes a uniform distribution on those outcomes. Cue the old joke, "I have a 50% chance to win the lottery, since I'll either win or I'll lose."
– user7530
yesterday
1
@user7530 Fair enough. I suppose I could have said "simple algebra" or "weighted averages" instead of "counting". But as an introduction to an answer to the OP's question I think what I wrote is OK.
– Ethan Bolker
yesterday
add a comment |
To add more to the answer by Ethan Bolker and flesh this out, probability functions are defined on sets, representing events, i.e. some set of outcomes of which we're interested in the probability of whatever we are querying or observing to happen as falling into or not, e.g. the probability that the temperature at noon tomorrow will be in the range $[25, 30]$.
Every probability function, which assigns to each event set $E$, itself a subset of the total set of possible outcomes, or sample space, $S$, is required satisfy the following rules, called the Kolmogorov axioms. The reason for this is they capture the most basic rules of how we expect probabilities to behave intuitively:
- Rule 1: There are no negative probabilities. That is, for every event $E$ we have $P(E) ge 0$. Since probabilities are meant to formalize the idea of "how many chances in..." is there for something to happen, it makes no sense to talk of a negative number of chances for the same reason that it makes no sense to talk of a negative number of apples. What does it mean to have -3 occurrences of something, or -6 apples held in my hand right now at this very moment in time?
- Rule 2: The probability of the entire sample space is 1. i.e. $P(S) = 1$. This should be intuitive, because at least some outcome must occur, and the set $S$ is the set of all possible outcomes, so whatever outcome occurs has to be within it. Thus the event $S$ will always occur no matter what.
- Rule 3: Probabilities of mutually exclusive events add. If we have an up-to-countable sequence of mutually exclusive events $E_1, E_2, E_3, cdots$, i.e. that $E_i cap E_j = emptyset$ for all possible pairs with $i ne j$, then we should have
$$P(E_1 cup E_2 cup E_3 ...) = sum_{i=1}^{infty} P(E_i)$$
Now as mentioned, we may not be able to assign every event a probability. For the case of a discrete sample space, i.e. where $S$ is a finite or at most countably infinite set, this may be doable. But for continuous sample spaces (e.g. $mathbb{R}$), there are subtleties that make it difficult to define a useful probability function in most cases for most sets using methods that are convenient to use such as integration, and thus we must restrict the domain of $P$ to not all subsets of $S$, but only some selected amount, which we call the $sigma$-field, usually denoted $Sigma$. That is, $mathrm{dom}(P) = Sigma subseteq 2^S$, and we are not thus allowed to consider events $E notin Sigma$. The definition of a $sigma$-field is just whatever is required to ensure that with regard to the above definition, all the sets involved in it make sense. Which basically means we must have
- Because of rule 2, in order for us to have $P(S) = 1$ we need $S$ to be in the domain of $P$ in the first place, so we must have $S in Sigma$.
- While this second stipulation is not strictly speaking required simply to make the above definition valid, we typically take that the complement $bar{E} = S backslash E$ of any event should be in $Sigma$. This is because very often we are interested in the probability of something NOT happening (e.g. the probability that a given number of people do NOT get better with some sort of medical treatment we are testing), and we want that question to make sense and thus must be able to have the event corresponding to this as an available input to our probability function $P$.
- Finally, so that rule 3 can make sense, given any countable sequence of members $E_1, E_2, E_3, cdots in Sigma$ we must have $(E_1 cup E_2 cup E_3 cup cdots) in Sigma$.
And that's about it.
add a comment |
Your Answer
StackExchange.ifUsing("editor", function () {
return StackExchange.using("mathjaxEditing", function () {
StackExchange.MarkdownEditor.creationCallbacks.add(function (editor, postfix) {
StackExchange.mathjaxEditing.prepareWmdForMathJax(editor, postfix, [["$", "$"], ["\\(","\\)"]]);
});
});
}, "mathjax-editing");
StackExchange.ready(function() {
var channelOptions = {
tags: "".split(" "),
id: "69"
};
initTagRenderer("".split(" "), "".split(" "), channelOptions);
StackExchange.using("externalEditor", function() {
// Have to fire editor after snippets, if snippets enabled
if (StackExchange.settings.snippets.snippetsEnabled) {
StackExchange.using("snippets", function() {
createEditor();
});
}
else {
createEditor();
}
});
function createEditor() {
StackExchange.prepareEditor({
heartbeatType: 'answer',
autoActivateHeartbeat: false,
convertImagesToLinks: true,
noModals: true,
showLowRepImageUploadWarning: true,
reputationToPostImages: 10,
bindNavPrevention: true,
postfix: "",
imageUploader: {
brandingHtml: "Powered by u003ca class="icon-imgur-white" href="https://imgur.com/"u003eu003c/au003e",
contentPolicyHtml: "User contributions licensed under u003ca href="https://creativecommons.org/licenses/by-sa/3.0/"u003ecc by-sa 3.0 with attribution requiredu003c/au003e u003ca href="https://stackoverflow.com/legal/content-policy"u003e(content policy)u003c/au003e",
allowUrls: true
},
noCode: true, onDemand: true,
discardSelector: ".discard-answer"
,immediatelyShowMarkdownHelp:true
});
}
});
Sign up or log in
StackExchange.ready(function () {
StackExchange.helpers.onClickDraftSave('#login-link');
});
Sign up using Google
Sign up using Facebook
Sign up using Email and Password
Post as a guest
Required, but never shown
StackExchange.ready(
function () {
StackExchange.openid.initPostLogin('.new-post-login', 'https%3a%2f%2fmath.stackexchange.com%2fquestions%2f3042059%2fwhat-are-the-foundations-of-probability-and-how-are-they-dependent-upon-a-sigm%23new-answer', 'question_page');
}
);
Post as a guest
Required, but never shown
2 Answers
2
active
oldest
votes
2 Answers
2
active
oldest
votes
active
oldest
votes
active
oldest
votes
Probability when there are only finitely many outcomes is a matter of counting. There are $36$ possible results from a roll of two dice and $6$ of them sum to $7$ so the probability of a sum of $7$ is $6/36$. You've measured the size of the set of outcomes that you are interested in.
It's harder to make rigorous sense of things when the set of possible results is infinite. What does it mean to choose two numbers at random in the interval $[1,6]$ and ask for their sum? Any particular pair, like $(1.3, pi)$, will have probability $0$.
You deal with this problem by replacing counting with integration. Unfortunately, the integration you learn in first year calculus ("Riemann integration") isn't powerful enough to derive all you need about probability. (It is enough to determine the probability that your two rolls total exactly $7$ is $0$, and to find the probability that it's at least $7$.)
For the definitions and theorems of rigorous probability theory (those are the "foundations" you ask about) you need "Lebesgue integration". That requires first carefully specifying the sets that you are going to ask for the probabilities of - and not every set is allowed, for technical reasons without which you can't make the mathematics work the way you want. It turns out that the set of sets whose probability you are going to ask about carries the name "$sigma$-field" or "sigma algebra". (It's not a field in the arithmetic sense.)
The essential point is that it's closed under countable set operations. That's what the "$sigma$" says. Your text may not provide a formal definition - you may not need it for NLP applications.
I am reading it a couple of times. I think it is going to take me some time to understand all of it. Thanks for the answer anyways.
– eddard.stark
Dec 16 at 1:43
1
I find the following answer related and useful Why do we need sigma-algebras to define probability spaces
– Jesper Hybel
Dec 24 at 15:18
"Probability when there are only finitely many outcomes is a matter of counting." This is a rather dangerous simplification since it assumes a uniform distribution on those outcomes. Cue the old joke, "I have a 50% chance to win the lottery, since I'll either win or I'll lose."
– user7530
yesterday
1
@user7530 Fair enough. I suppose I could have said "simple algebra" or "weighted averages" instead of "counting". But as an introduction to an answer to the OP's question I think what I wrote is OK.
– Ethan Bolker
yesterday
add a comment |
Probability when there are only finitely many outcomes is a matter of counting. There are $36$ possible results from a roll of two dice and $6$ of them sum to $7$ so the probability of a sum of $7$ is $6/36$. You've measured the size of the set of outcomes that you are interested in.
It's harder to make rigorous sense of things when the set of possible results is infinite. What does it mean to choose two numbers at random in the interval $[1,6]$ and ask for their sum? Any particular pair, like $(1.3, pi)$, will have probability $0$.
You deal with this problem by replacing counting with integration. Unfortunately, the integration you learn in first year calculus ("Riemann integration") isn't powerful enough to derive all you need about probability. (It is enough to determine the probability that your two rolls total exactly $7$ is $0$, and to find the probability that it's at least $7$.)
For the definitions and theorems of rigorous probability theory (those are the "foundations" you ask about) you need "Lebesgue integration". That requires first carefully specifying the sets that you are going to ask for the probabilities of - and not every set is allowed, for technical reasons without which you can't make the mathematics work the way you want. It turns out that the set of sets whose probability you are going to ask about carries the name "$sigma$-field" or "sigma algebra". (It's not a field in the arithmetic sense.)
The essential point is that it's closed under countable set operations. That's what the "$sigma$" says. Your text may not provide a formal definition - you may not need it for NLP applications.
I am reading it a couple of times. I think it is going to take me some time to understand all of it. Thanks for the answer anyways.
– eddard.stark
Dec 16 at 1:43
1
I find the following answer related and useful Why do we need sigma-algebras to define probability spaces
– Jesper Hybel
Dec 24 at 15:18
"Probability when there are only finitely many outcomes is a matter of counting." This is a rather dangerous simplification since it assumes a uniform distribution on those outcomes. Cue the old joke, "I have a 50% chance to win the lottery, since I'll either win or I'll lose."
– user7530
yesterday
1
@user7530 Fair enough. I suppose I could have said "simple algebra" or "weighted averages" instead of "counting". But as an introduction to an answer to the OP's question I think what I wrote is OK.
– Ethan Bolker
yesterday
add a comment |
Probability when there are only finitely many outcomes is a matter of counting. There are $36$ possible results from a roll of two dice and $6$ of them sum to $7$ so the probability of a sum of $7$ is $6/36$. You've measured the size of the set of outcomes that you are interested in.
It's harder to make rigorous sense of things when the set of possible results is infinite. What does it mean to choose two numbers at random in the interval $[1,6]$ and ask for their sum? Any particular pair, like $(1.3, pi)$, will have probability $0$.
You deal with this problem by replacing counting with integration. Unfortunately, the integration you learn in first year calculus ("Riemann integration") isn't powerful enough to derive all you need about probability. (It is enough to determine the probability that your two rolls total exactly $7$ is $0$, and to find the probability that it's at least $7$.)
For the definitions and theorems of rigorous probability theory (those are the "foundations" you ask about) you need "Lebesgue integration". That requires first carefully specifying the sets that you are going to ask for the probabilities of - and not every set is allowed, for technical reasons without which you can't make the mathematics work the way you want. It turns out that the set of sets whose probability you are going to ask about carries the name "$sigma$-field" or "sigma algebra". (It's not a field in the arithmetic sense.)
The essential point is that it's closed under countable set operations. That's what the "$sigma$" says. Your text may not provide a formal definition - you may not need it for NLP applications.
Probability when there are only finitely many outcomes is a matter of counting. There are $36$ possible results from a roll of two dice and $6$ of them sum to $7$ so the probability of a sum of $7$ is $6/36$. You've measured the size of the set of outcomes that you are interested in.
It's harder to make rigorous sense of things when the set of possible results is infinite. What does it mean to choose two numbers at random in the interval $[1,6]$ and ask for their sum? Any particular pair, like $(1.3, pi)$, will have probability $0$.
You deal with this problem by replacing counting with integration. Unfortunately, the integration you learn in first year calculus ("Riemann integration") isn't powerful enough to derive all you need about probability. (It is enough to determine the probability that your two rolls total exactly $7$ is $0$, and to find the probability that it's at least $7$.)
For the definitions and theorems of rigorous probability theory (those are the "foundations" you ask about) you need "Lebesgue integration". That requires first carefully specifying the sets that you are going to ask for the probabilities of - and not every set is allowed, for technical reasons without which you can't make the mathematics work the way you want. It turns out that the set of sets whose probability you are going to ask about carries the name "$sigma$-field" or "sigma algebra". (It's not a field in the arithmetic sense.)
The essential point is that it's closed under countable set operations. That's what the "$sigma$" says. Your text may not provide a formal definition - you may not need it for NLP applications.
edited Dec 16 at 22:17


Lord_Farin
15.5k636108
15.5k636108
answered Dec 16 at 1:00
Ethan Bolker
41.2k547108
41.2k547108
I am reading it a couple of times. I think it is going to take me some time to understand all of it. Thanks for the answer anyways.
– eddard.stark
Dec 16 at 1:43
1
I find the following answer related and useful Why do we need sigma-algebras to define probability spaces
– Jesper Hybel
Dec 24 at 15:18
"Probability when there are only finitely many outcomes is a matter of counting." This is a rather dangerous simplification since it assumes a uniform distribution on those outcomes. Cue the old joke, "I have a 50% chance to win the lottery, since I'll either win or I'll lose."
– user7530
yesterday
1
@user7530 Fair enough. I suppose I could have said "simple algebra" or "weighted averages" instead of "counting". But as an introduction to an answer to the OP's question I think what I wrote is OK.
– Ethan Bolker
yesterday
add a comment |
I am reading it a couple of times. I think it is going to take me some time to understand all of it. Thanks for the answer anyways.
– eddard.stark
Dec 16 at 1:43
1
I find the following answer related and useful Why do we need sigma-algebras to define probability spaces
– Jesper Hybel
Dec 24 at 15:18
"Probability when there are only finitely many outcomes is a matter of counting." This is a rather dangerous simplification since it assumes a uniform distribution on those outcomes. Cue the old joke, "I have a 50% chance to win the lottery, since I'll either win or I'll lose."
– user7530
yesterday
1
@user7530 Fair enough. I suppose I could have said "simple algebra" or "weighted averages" instead of "counting". But as an introduction to an answer to the OP's question I think what I wrote is OK.
– Ethan Bolker
yesterday
I am reading it a couple of times. I think it is going to take me some time to understand all of it. Thanks for the answer anyways.
– eddard.stark
Dec 16 at 1:43
I am reading it a couple of times. I think it is going to take me some time to understand all of it. Thanks for the answer anyways.
– eddard.stark
Dec 16 at 1:43
1
1
I find the following answer related and useful Why do we need sigma-algebras to define probability spaces
– Jesper Hybel
Dec 24 at 15:18
I find the following answer related and useful Why do we need sigma-algebras to define probability spaces
– Jesper Hybel
Dec 24 at 15:18
"Probability when there are only finitely many outcomes is a matter of counting." This is a rather dangerous simplification since it assumes a uniform distribution on those outcomes. Cue the old joke, "I have a 50% chance to win the lottery, since I'll either win or I'll lose."
– user7530
yesterday
"Probability when there are only finitely many outcomes is a matter of counting." This is a rather dangerous simplification since it assumes a uniform distribution on those outcomes. Cue the old joke, "I have a 50% chance to win the lottery, since I'll either win or I'll lose."
– user7530
yesterday
1
1
@user7530 Fair enough. I suppose I could have said "simple algebra" or "weighted averages" instead of "counting". But as an introduction to an answer to the OP's question I think what I wrote is OK.
– Ethan Bolker
yesterday
@user7530 Fair enough. I suppose I could have said "simple algebra" or "weighted averages" instead of "counting". But as an introduction to an answer to the OP's question I think what I wrote is OK.
– Ethan Bolker
yesterday
add a comment |
To add more to the answer by Ethan Bolker and flesh this out, probability functions are defined on sets, representing events, i.e. some set of outcomes of which we're interested in the probability of whatever we are querying or observing to happen as falling into or not, e.g. the probability that the temperature at noon tomorrow will be in the range $[25, 30]$.
Every probability function, which assigns to each event set $E$, itself a subset of the total set of possible outcomes, or sample space, $S$, is required satisfy the following rules, called the Kolmogorov axioms. The reason for this is they capture the most basic rules of how we expect probabilities to behave intuitively:
- Rule 1: There are no negative probabilities. That is, for every event $E$ we have $P(E) ge 0$. Since probabilities are meant to formalize the idea of "how many chances in..." is there for something to happen, it makes no sense to talk of a negative number of chances for the same reason that it makes no sense to talk of a negative number of apples. What does it mean to have -3 occurrences of something, or -6 apples held in my hand right now at this very moment in time?
- Rule 2: The probability of the entire sample space is 1. i.e. $P(S) = 1$. This should be intuitive, because at least some outcome must occur, and the set $S$ is the set of all possible outcomes, so whatever outcome occurs has to be within it. Thus the event $S$ will always occur no matter what.
- Rule 3: Probabilities of mutually exclusive events add. If we have an up-to-countable sequence of mutually exclusive events $E_1, E_2, E_3, cdots$, i.e. that $E_i cap E_j = emptyset$ for all possible pairs with $i ne j$, then we should have
$$P(E_1 cup E_2 cup E_3 ...) = sum_{i=1}^{infty} P(E_i)$$
Now as mentioned, we may not be able to assign every event a probability. For the case of a discrete sample space, i.e. where $S$ is a finite or at most countably infinite set, this may be doable. But for continuous sample spaces (e.g. $mathbb{R}$), there are subtleties that make it difficult to define a useful probability function in most cases for most sets using methods that are convenient to use such as integration, and thus we must restrict the domain of $P$ to not all subsets of $S$, but only some selected amount, which we call the $sigma$-field, usually denoted $Sigma$. That is, $mathrm{dom}(P) = Sigma subseteq 2^S$, and we are not thus allowed to consider events $E notin Sigma$. The definition of a $sigma$-field is just whatever is required to ensure that with regard to the above definition, all the sets involved in it make sense. Which basically means we must have
- Because of rule 2, in order for us to have $P(S) = 1$ we need $S$ to be in the domain of $P$ in the first place, so we must have $S in Sigma$.
- While this second stipulation is not strictly speaking required simply to make the above definition valid, we typically take that the complement $bar{E} = S backslash E$ of any event should be in $Sigma$. This is because very often we are interested in the probability of something NOT happening (e.g. the probability that a given number of people do NOT get better with some sort of medical treatment we are testing), and we want that question to make sense and thus must be able to have the event corresponding to this as an available input to our probability function $P$.
- Finally, so that rule 3 can make sense, given any countable sequence of members $E_1, E_2, E_3, cdots in Sigma$ we must have $(E_1 cup E_2 cup E_3 cup cdots) in Sigma$.
And that's about it.
add a comment |
To add more to the answer by Ethan Bolker and flesh this out, probability functions are defined on sets, representing events, i.e. some set of outcomes of which we're interested in the probability of whatever we are querying or observing to happen as falling into or not, e.g. the probability that the temperature at noon tomorrow will be in the range $[25, 30]$.
Every probability function, which assigns to each event set $E$, itself a subset of the total set of possible outcomes, or sample space, $S$, is required satisfy the following rules, called the Kolmogorov axioms. The reason for this is they capture the most basic rules of how we expect probabilities to behave intuitively:
- Rule 1: There are no negative probabilities. That is, for every event $E$ we have $P(E) ge 0$. Since probabilities are meant to formalize the idea of "how many chances in..." is there for something to happen, it makes no sense to talk of a negative number of chances for the same reason that it makes no sense to talk of a negative number of apples. What does it mean to have -3 occurrences of something, or -6 apples held in my hand right now at this very moment in time?
- Rule 2: The probability of the entire sample space is 1. i.e. $P(S) = 1$. This should be intuitive, because at least some outcome must occur, and the set $S$ is the set of all possible outcomes, so whatever outcome occurs has to be within it. Thus the event $S$ will always occur no matter what.
- Rule 3: Probabilities of mutually exclusive events add. If we have an up-to-countable sequence of mutually exclusive events $E_1, E_2, E_3, cdots$, i.e. that $E_i cap E_j = emptyset$ for all possible pairs with $i ne j$, then we should have
$$P(E_1 cup E_2 cup E_3 ...) = sum_{i=1}^{infty} P(E_i)$$
Now as mentioned, we may not be able to assign every event a probability. For the case of a discrete sample space, i.e. where $S$ is a finite or at most countably infinite set, this may be doable. But for continuous sample spaces (e.g. $mathbb{R}$), there are subtleties that make it difficult to define a useful probability function in most cases for most sets using methods that are convenient to use such as integration, and thus we must restrict the domain of $P$ to not all subsets of $S$, but only some selected amount, which we call the $sigma$-field, usually denoted $Sigma$. That is, $mathrm{dom}(P) = Sigma subseteq 2^S$, and we are not thus allowed to consider events $E notin Sigma$. The definition of a $sigma$-field is just whatever is required to ensure that with regard to the above definition, all the sets involved in it make sense. Which basically means we must have
- Because of rule 2, in order for us to have $P(S) = 1$ we need $S$ to be in the domain of $P$ in the first place, so we must have $S in Sigma$.
- While this second stipulation is not strictly speaking required simply to make the above definition valid, we typically take that the complement $bar{E} = S backslash E$ of any event should be in $Sigma$. This is because very often we are interested in the probability of something NOT happening (e.g. the probability that a given number of people do NOT get better with some sort of medical treatment we are testing), and we want that question to make sense and thus must be able to have the event corresponding to this as an available input to our probability function $P$.
- Finally, so that rule 3 can make sense, given any countable sequence of members $E_1, E_2, E_3, cdots in Sigma$ we must have $(E_1 cup E_2 cup E_3 cup cdots) in Sigma$.
And that's about it.
add a comment |
To add more to the answer by Ethan Bolker and flesh this out, probability functions are defined on sets, representing events, i.e. some set of outcomes of which we're interested in the probability of whatever we are querying or observing to happen as falling into or not, e.g. the probability that the temperature at noon tomorrow will be in the range $[25, 30]$.
Every probability function, which assigns to each event set $E$, itself a subset of the total set of possible outcomes, or sample space, $S$, is required satisfy the following rules, called the Kolmogorov axioms. The reason for this is they capture the most basic rules of how we expect probabilities to behave intuitively:
- Rule 1: There are no negative probabilities. That is, for every event $E$ we have $P(E) ge 0$. Since probabilities are meant to formalize the idea of "how many chances in..." is there for something to happen, it makes no sense to talk of a negative number of chances for the same reason that it makes no sense to talk of a negative number of apples. What does it mean to have -3 occurrences of something, or -6 apples held in my hand right now at this very moment in time?
- Rule 2: The probability of the entire sample space is 1. i.e. $P(S) = 1$. This should be intuitive, because at least some outcome must occur, and the set $S$ is the set of all possible outcomes, so whatever outcome occurs has to be within it. Thus the event $S$ will always occur no matter what.
- Rule 3: Probabilities of mutually exclusive events add. If we have an up-to-countable sequence of mutually exclusive events $E_1, E_2, E_3, cdots$, i.e. that $E_i cap E_j = emptyset$ for all possible pairs with $i ne j$, then we should have
$$P(E_1 cup E_2 cup E_3 ...) = sum_{i=1}^{infty} P(E_i)$$
Now as mentioned, we may not be able to assign every event a probability. For the case of a discrete sample space, i.e. where $S$ is a finite or at most countably infinite set, this may be doable. But for continuous sample spaces (e.g. $mathbb{R}$), there are subtleties that make it difficult to define a useful probability function in most cases for most sets using methods that are convenient to use such as integration, and thus we must restrict the domain of $P$ to not all subsets of $S$, but only some selected amount, which we call the $sigma$-field, usually denoted $Sigma$. That is, $mathrm{dom}(P) = Sigma subseteq 2^S$, and we are not thus allowed to consider events $E notin Sigma$. The definition of a $sigma$-field is just whatever is required to ensure that with regard to the above definition, all the sets involved in it make sense. Which basically means we must have
- Because of rule 2, in order for us to have $P(S) = 1$ we need $S$ to be in the domain of $P$ in the first place, so we must have $S in Sigma$.
- While this second stipulation is not strictly speaking required simply to make the above definition valid, we typically take that the complement $bar{E} = S backslash E$ of any event should be in $Sigma$. This is because very often we are interested in the probability of something NOT happening (e.g. the probability that a given number of people do NOT get better with some sort of medical treatment we are testing), and we want that question to make sense and thus must be able to have the event corresponding to this as an available input to our probability function $P$.
- Finally, so that rule 3 can make sense, given any countable sequence of members $E_1, E_2, E_3, cdots in Sigma$ we must have $(E_1 cup E_2 cup E_3 cup cdots) in Sigma$.
And that's about it.
To add more to the answer by Ethan Bolker and flesh this out, probability functions are defined on sets, representing events, i.e. some set of outcomes of which we're interested in the probability of whatever we are querying or observing to happen as falling into or not, e.g. the probability that the temperature at noon tomorrow will be in the range $[25, 30]$.
Every probability function, which assigns to each event set $E$, itself a subset of the total set of possible outcomes, or sample space, $S$, is required satisfy the following rules, called the Kolmogorov axioms. The reason for this is they capture the most basic rules of how we expect probabilities to behave intuitively:
- Rule 1: There are no negative probabilities. That is, for every event $E$ we have $P(E) ge 0$. Since probabilities are meant to formalize the idea of "how many chances in..." is there for something to happen, it makes no sense to talk of a negative number of chances for the same reason that it makes no sense to talk of a negative number of apples. What does it mean to have -3 occurrences of something, or -6 apples held in my hand right now at this very moment in time?
- Rule 2: The probability of the entire sample space is 1. i.e. $P(S) = 1$. This should be intuitive, because at least some outcome must occur, and the set $S$ is the set of all possible outcomes, so whatever outcome occurs has to be within it. Thus the event $S$ will always occur no matter what.
- Rule 3: Probabilities of mutually exclusive events add. If we have an up-to-countable sequence of mutually exclusive events $E_1, E_2, E_3, cdots$, i.e. that $E_i cap E_j = emptyset$ for all possible pairs with $i ne j$, then we should have
$$P(E_1 cup E_2 cup E_3 ...) = sum_{i=1}^{infty} P(E_i)$$
Now as mentioned, we may not be able to assign every event a probability. For the case of a discrete sample space, i.e. where $S$ is a finite or at most countably infinite set, this may be doable. But for continuous sample spaces (e.g. $mathbb{R}$), there are subtleties that make it difficult to define a useful probability function in most cases for most sets using methods that are convenient to use such as integration, and thus we must restrict the domain of $P$ to not all subsets of $S$, but only some selected amount, which we call the $sigma$-field, usually denoted $Sigma$. That is, $mathrm{dom}(P) = Sigma subseteq 2^S$, and we are not thus allowed to consider events $E notin Sigma$. The definition of a $sigma$-field is just whatever is required to ensure that with regard to the above definition, all the sets involved in it make sense. Which basically means we must have
- Because of rule 2, in order for us to have $P(S) = 1$ we need $S$ to be in the domain of $P$ in the first place, so we must have $S in Sigma$.
- While this second stipulation is not strictly speaking required simply to make the above definition valid, we typically take that the complement $bar{E} = S backslash E$ of any event should be in $Sigma$. This is because very often we are interested in the probability of something NOT happening (e.g. the probability that a given number of people do NOT get better with some sort of medical treatment we are testing), and we want that question to make sense and thus must be able to have the event corresponding to this as an available input to our probability function $P$.
- Finally, so that rule 3 can make sense, given any countable sequence of members $E_1, E_2, E_3, cdots in Sigma$ we must have $(E_1 cup E_2 cup E_3 cup cdots) in Sigma$.
And that's about it.
answered 12 hours ago
The_Sympathizer
7,1672243
7,1672243
add a comment |
add a comment |
Thanks for contributing an answer to Mathematics Stack Exchange!
- Please be sure to answer the question. Provide details and share your research!
But avoid …
- Asking for help, clarification, or responding to other answers.
- Making statements based on opinion; back them up with references or personal experience.
Use MathJax to format equations. MathJax reference.
To learn more, see our tips on writing great answers.
Some of your past answers have not been well-received, and you're in danger of being blocked from answering.
Please pay close attention to the following guidance:
- Please be sure to answer the question. Provide details and share your research!
But avoid …
- Asking for help, clarification, or responding to other answers.
- Making statements based on opinion; back them up with references or personal experience.
To learn more, see our tips on writing great answers.
Sign up or log in
StackExchange.ready(function () {
StackExchange.helpers.onClickDraftSave('#login-link');
});
Sign up using Google
Sign up using Facebook
Sign up using Email and Password
Post as a guest
Required, but never shown
StackExchange.ready(
function () {
StackExchange.openid.initPostLogin('.new-post-login', 'https%3a%2f%2fmath.stackexchange.com%2fquestions%2f3042059%2fwhat-are-the-foundations-of-probability-and-how-are-they-dependent-upon-a-sigm%23new-answer', 'question_page');
}
);
Post as a guest
Required, but never shown
Sign up or log in
StackExchange.ready(function () {
StackExchange.helpers.onClickDraftSave('#login-link');
});
Sign up using Google
Sign up using Facebook
Sign up using Email and Password
Post as a guest
Required, but never shown
Sign up or log in
StackExchange.ready(function () {
StackExchange.helpers.onClickDraftSave('#login-link');
});
Sign up using Google
Sign up using Facebook
Sign up using Email and Password
Post as a guest
Required, but never shown
Sign up or log in
StackExchange.ready(function () {
StackExchange.helpers.onClickDraftSave('#login-link');
});
Sign up using Google
Sign up using Facebook
Sign up using Email and Password
Sign up using Google
Sign up using Facebook
Sign up using Email and Password
Post as a guest
Required, but never shown
Required, but never shown
Required, but never shown
Required, but never shown
Required, but never shown
Required, but never shown
Required, but never shown
Required, but never shown
Required, but never shown
veb,MgZX7JKxfnU,Lr4UaHPXggphqREBPne6Aj,34S nzd7bmjWS2 qzS sBUKidF gmyQMp9lTY,Hvg I4yiZz,i
5
But this is not a textbook on probability theory. This is a book on Natural Language Processing which includes some preliminaries of probability theory -- this is not the same thing. If you want to understand the foundations of probability, consult a textbook on that, not on something that merely uses probabilities.
– Clement C.
Dec 16 at 0:48
1
@eddard Some people are concerned with the scope of your question being too broad ("foundations of probability theory" is a topic of considerable size). Would you mind editing the post to be only about the second bullet point?
– Lord_Farin
Dec 16 at 22:00
2
@Lord_Farin someone tried to focus it and improve the title. Then someone didn't like it and rolled it back. Not sure why, since that would make this a good question.
– Don Hatch
Dec 18 at 8:09