Expected value and projection of a normal random variable onto a linear span…?
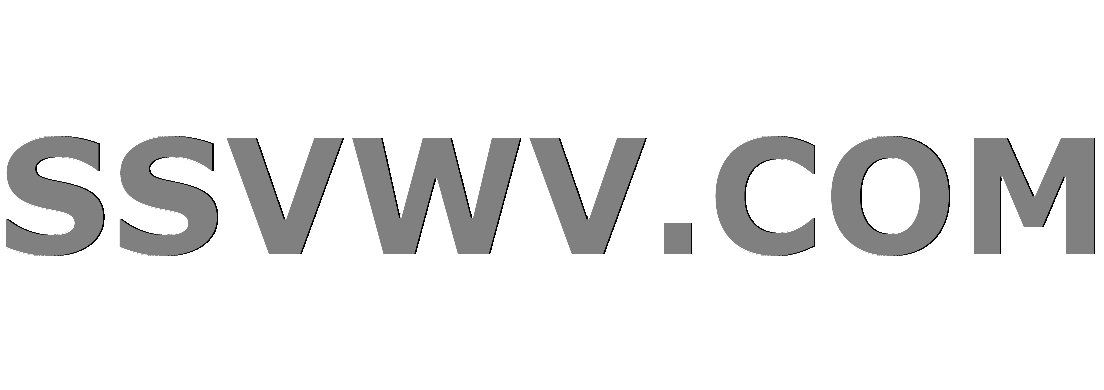
Multi tool use
$begingroup$
I just wanted to clarify a part of a proof which used the fact a random variable has zero mean.
Suppose $X, Z_{s_1},dots,Z_{s_n}$ are all jointly normal random variables for all $s_i leq t$ and $n geq 1$.
Define $mathcal{L}(Z,t)$ as set of random variables of the form
$$c_1 Z_{s_1}+ dots + c_n Z_{s_n}$$
for $c_i in mathbb{R}$ and $s_i leq t$, $n geq 1$, along with all their limit points (in $L^2(P)$).
Define $tilde{X} = X - mathcal{P}_L (X)$ where $mathcal{P}_L$ is the projection onto the space $mathcal{L}(Z,t)$.
Then the proof use $mathbb{E}[tilde{X}] = 0$.
My understanding of this, is that the projection $mathcal{P}_L (X)$ coincides with the conditional expectation
$$mathcal{P}_L (X) = E[X|mathcal{F}_L]$$
where $mathcal{F}_L$ is the $sigma$-field generated by the random variables in $mathcal{L}(Z,t)$. And hence by law of total expectation we have
$$mathbb{E}[tilde{X}] = mathbb{E}[X] - mathbb{E}[E[X|mathcal{F}_L]] = mathbb{E}[X]-mathbb{E}[X] =0$$
Is this correct? The proof doesn't really explain, and the relationship between projection and probability spaces is still a bit confusing to me.
geometry probability-theory projective-geometry
$endgroup$
add a comment |
$begingroup$
I just wanted to clarify a part of a proof which used the fact a random variable has zero mean.
Suppose $X, Z_{s_1},dots,Z_{s_n}$ are all jointly normal random variables for all $s_i leq t$ and $n geq 1$.
Define $mathcal{L}(Z,t)$ as set of random variables of the form
$$c_1 Z_{s_1}+ dots + c_n Z_{s_n}$$
for $c_i in mathbb{R}$ and $s_i leq t$, $n geq 1$, along with all their limit points (in $L^2(P)$).
Define $tilde{X} = X - mathcal{P}_L (X)$ where $mathcal{P}_L$ is the projection onto the space $mathcal{L}(Z,t)$.
Then the proof use $mathbb{E}[tilde{X}] = 0$.
My understanding of this, is that the projection $mathcal{P}_L (X)$ coincides with the conditional expectation
$$mathcal{P}_L (X) = E[X|mathcal{F}_L]$$
where $mathcal{F}_L$ is the $sigma$-field generated by the random variables in $mathcal{L}(Z,t)$. And hence by law of total expectation we have
$$mathbb{E}[tilde{X}] = mathbb{E}[X] - mathbb{E}[E[X|mathcal{F}_L]] = mathbb{E}[X]-mathbb{E}[X] =0$$
Is this correct? The proof doesn't really explain, and the relationship between projection and probability spaces is still a bit confusing to me.
geometry probability-theory projective-geometry
$endgroup$
$begingroup$
What proof is this? What source?
$endgroup$
– Zachary Selk
Dec 21 '18 at 5:02
$begingroup$
It is part of Oksander's SDE book lemma 6.2.2
$endgroup$
– Xiaomi
Dec 21 '18 at 5:11
add a comment |
$begingroup$
I just wanted to clarify a part of a proof which used the fact a random variable has zero mean.
Suppose $X, Z_{s_1},dots,Z_{s_n}$ are all jointly normal random variables for all $s_i leq t$ and $n geq 1$.
Define $mathcal{L}(Z,t)$ as set of random variables of the form
$$c_1 Z_{s_1}+ dots + c_n Z_{s_n}$$
for $c_i in mathbb{R}$ and $s_i leq t$, $n geq 1$, along with all their limit points (in $L^2(P)$).
Define $tilde{X} = X - mathcal{P}_L (X)$ where $mathcal{P}_L$ is the projection onto the space $mathcal{L}(Z,t)$.
Then the proof use $mathbb{E}[tilde{X}] = 0$.
My understanding of this, is that the projection $mathcal{P}_L (X)$ coincides with the conditional expectation
$$mathcal{P}_L (X) = E[X|mathcal{F}_L]$$
where $mathcal{F}_L$ is the $sigma$-field generated by the random variables in $mathcal{L}(Z,t)$. And hence by law of total expectation we have
$$mathbb{E}[tilde{X}] = mathbb{E}[X] - mathbb{E}[E[X|mathcal{F}_L]] = mathbb{E}[X]-mathbb{E}[X] =0$$
Is this correct? The proof doesn't really explain, and the relationship between projection and probability spaces is still a bit confusing to me.
geometry probability-theory projective-geometry
$endgroup$
I just wanted to clarify a part of a proof which used the fact a random variable has zero mean.
Suppose $X, Z_{s_1},dots,Z_{s_n}$ are all jointly normal random variables for all $s_i leq t$ and $n geq 1$.
Define $mathcal{L}(Z,t)$ as set of random variables of the form
$$c_1 Z_{s_1}+ dots + c_n Z_{s_n}$$
for $c_i in mathbb{R}$ and $s_i leq t$, $n geq 1$, along with all their limit points (in $L^2(P)$).
Define $tilde{X} = X - mathcal{P}_L (X)$ where $mathcal{P}_L$ is the projection onto the space $mathcal{L}(Z,t)$.
Then the proof use $mathbb{E}[tilde{X}] = 0$.
My understanding of this, is that the projection $mathcal{P}_L (X)$ coincides with the conditional expectation
$$mathcal{P}_L (X) = E[X|mathcal{F}_L]$$
where $mathcal{F}_L$ is the $sigma$-field generated by the random variables in $mathcal{L}(Z,t)$. And hence by law of total expectation we have
$$mathbb{E}[tilde{X}] = mathbb{E}[X] - mathbb{E}[E[X|mathcal{F}_L]] = mathbb{E}[X]-mathbb{E}[X] =0$$
Is this correct? The proof doesn't really explain, and the relationship between projection and probability spaces is still a bit confusing to me.
geometry probability-theory projective-geometry
geometry probability-theory projective-geometry
edited Dec 21 '18 at 5:59
Xiaomi
asked Dec 21 '18 at 4:54
XiaomiXiaomi
1,066115
1,066115
$begingroup$
What proof is this? What source?
$endgroup$
– Zachary Selk
Dec 21 '18 at 5:02
$begingroup$
It is part of Oksander's SDE book lemma 6.2.2
$endgroup$
– Xiaomi
Dec 21 '18 at 5:11
add a comment |
$begingroup$
What proof is this? What source?
$endgroup$
– Zachary Selk
Dec 21 '18 at 5:02
$begingroup$
It is part of Oksander's SDE book lemma 6.2.2
$endgroup$
– Xiaomi
Dec 21 '18 at 5:11
$begingroup$
What proof is this? What source?
$endgroup$
– Zachary Selk
Dec 21 '18 at 5:02
$begingroup$
What proof is this? What source?
$endgroup$
– Zachary Selk
Dec 21 '18 at 5:02
$begingroup$
It is part of Oksander's SDE book lemma 6.2.2
$endgroup$
– Xiaomi
Dec 21 '18 at 5:11
$begingroup$
It is part of Oksander's SDE book lemma 6.2.2
$endgroup$
– Xiaomi
Dec 21 '18 at 5:11
add a comment |
2 Answers
2
active
oldest
votes
$begingroup$
As @Kavi Rama Murthy pointed out, it holds that
$$
mathcal{L}(Z,t) neq L^2(sigma(Z_s;sleq t)).
$$ But if we assume additionally that $$(X,Z_s;sleq t)$$
is jointly normally distributed, it holds that
$$
P_{mathcal{L}(Z,t) }X = P_{L^2(sigma(Z_s;sleq t))}X.
$$ To see this, notice that if $(X,Y_1,ldots ,Y_n)$ is jointly normal, then
$$
operatorname{Cov}(X,Y_i) = 0,,forall ileq n Leftrightarrow Xtext{ and }(Y_i)_{ileq n} text{ are independent.}
$$ It holds that
$$
operatorname{Cov}(X-P_{mathcal{L}(Z,t) }X, Z_s) = 0,quadforall sleq t,
$$ by the definition of the projection. And since $(X,Z_s;sleq t)$ is jointly normal, so is $(X-P_{mathcal{L}(Z,t) }X,Z_s;sleq t).$ This implies that
$$
X-P_{mathcal{L}(Z,t) }X perp !!! perp (Z_s)_{sleq t}.
$$ Therefore, we have
$$
P_{L^2(sigma(Z_s;sleq t))}[X-P_{mathcal{L}(Z,t) }X]=P_{L^2(sigma(Z_s;sleq t))}X-P_{mathcal{L}(Z,t) }X=0,
$$as desired. In your notation, $mathcal{F}_L=sigma(Z_s;sleq t)$ and $P_{L^2(sigma(Z_s;sleq t))}X = E[X|mathcal{F}_L].$
$endgroup$
add a comment |
$begingroup$
Your argument is not valid. Conditional expectation is the projection on the space of all random variables measurable w.r.t. $sigma {Z_s:sleq t}$, not the projection on to the vector space spanned by $ {Z_s:sleq t}$. The former space contains many nonlinear functions of $ {Z_s:sleq t}$ like $Z_t^{2}$.
$endgroup$
add a comment |
Your Answer
StackExchange.ifUsing("editor", function () {
return StackExchange.using("mathjaxEditing", function () {
StackExchange.MarkdownEditor.creationCallbacks.add(function (editor, postfix) {
StackExchange.mathjaxEditing.prepareWmdForMathJax(editor, postfix, [["$", "$"], ["\\(","\\)"]]);
});
});
}, "mathjax-editing");
StackExchange.ready(function() {
var channelOptions = {
tags: "".split(" "),
id: "69"
};
initTagRenderer("".split(" "), "".split(" "), channelOptions);
StackExchange.using("externalEditor", function() {
// Have to fire editor after snippets, if snippets enabled
if (StackExchange.settings.snippets.snippetsEnabled) {
StackExchange.using("snippets", function() {
createEditor();
});
}
else {
createEditor();
}
});
function createEditor() {
StackExchange.prepareEditor({
heartbeatType: 'answer',
autoActivateHeartbeat: false,
convertImagesToLinks: true,
noModals: true,
showLowRepImageUploadWarning: true,
reputationToPostImages: 10,
bindNavPrevention: true,
postfix: "",
imageUploader: {
brandingHtml: "Powered by u003ca class="icon-imgur-white" href="https://imgur.com/"u003eu003c/au003e",
contentPolicyHtml: "User contributions licensed under u003ca href="https://creativecommons.org/licenses/by-sa/3.0/"u003ecc by-sa 3.0 with attribution requiredu003c/au003e u003ca href="https://stackoverflow.com/legal/content-policy"u003e(content policy)u003c/au003e",
allowUrls: true
},
noCode: true, onDemand: true,
discardSelector: ".discard-answer"
,immediatelyShowMarkdownHelp:true
});
}
});
Sign up or log in
StackExchange.ready(function () {
StackExchange.helpers.onClickDraftSave('#login-link');
});
Sign up using Google
Sign up using Facebook
Sign up using Email and Password
Post as a guest
Required, but never shown
StackExchange.ready(
function () {
StackExchange.openid.initPostLogin('.new-post-login', 'https%3a%2f%2fmath.stackexchange.com%2fquestions%2f3048185%2fexpected-value-and-projection-of-a-normal-random-variable-onto-a-linear-span%23new-answer', 'question_page');
}
);
Post as a guest
Required, but never shown
2 Answers
2
active
oldest
votes
2 Answers
2
active
oldest
votes
active
oldest
votes
active
oldest
votes
$begingroup$
As @Kavi Rama Murthy pointed out, it holds that
$$
mathcal{L}(Z,t) neq L^2(sigma(Z_s;sleq t)).
$$ But if we assume additionally that $$(X,Z_s;sleq t)$$
is jointly normally distributed, it holds that
$$
P_{mathcal{L}(Z,t) }X = P_{L^2(sigma(Z_s;sleq t))}X.
$$ To see this, notice that if $(X,Y_1,ldots ,Y_n)$ is jointly normal, then
$$
operatorname{Cov}(X,Y_i) = 0,,forall ileq n Leftrightarrow Xtext{ and }(Y_i)_{ileq n} text{ are independent.}
$$ It holds that
$$
operatorname{Cov}(X-P_{mathcal{L}(Z,t) }X, Z_s) = 0,quadforall sleq t,
$$ by the definition of the projection. And since $(X,Z_s;sleq t)$ is jointly normal, so is $(X-P_{mathcal{L}(Z,t) }X,Z_s;sleq t).$ This implies that
$$
X-P_{mathcal{L}(Z,t) }X perp !!! perp (Z_s)_{sleq t}.
$$ Therefore, we have
$$
P_{L^2(sigma(Z_s;sleq t))}[X-P_{mathcal{L}(Z,t) }X]=P_{L^2(sigma(Z_s;sleq t))}X-P_{mathcal{L}(Z,t) }X=0,
$$as desired. In your notation, $mathcal{F}_L=sigma(Z_s;sleq t)$ and $P_{L^2(sigma(Z_s;sleq t))}X = E[X|mathcal{F}_L].$
$endgroup$
add a comment |
$begingroup$
As @Kavi Rama Murthy pointed out, it holds that
$$
mathcal{L}(Z,t) neq L^2(sigma(Z_s;sleq t)).
$$ But if we assume additionally that $$(X,Z_s;sleq t)$$
is jointly normally distributed, it holds that
$$
P_{mathcal{L}(Z,t) }X = P_{L^2(sigma(Z_s;sleq t))}X.
$$ To see this, notice that if $(X,Y_1,ldots ,Y_n)$ is jointly normal, then
$$
operatorname{Cov}(X,Y_i) = 0,,forall ileq n Leftrightarrow Xtext{ and }(Y_i)_{ileq n} text{ are independent.}
$$ It holds that
$$
operatorname{Cov}(X-P_{mathcal{L}(Z,t) }X, Z_s) = 0,quadforall sleq t,
$$ by the definition of the projection. And since $(X,Z_s;sleq t)$ is jointly normal, so is $(X-P_{mathcal{L}(Z,t) }X,Z_s;sleq t).$ This implies that
$$
X-P_{mathcal{L}(Z,t) }X perp !!! perp (Z_s)_{sleq t}.
$$ Therefore, we have
$$
P_{L^2(sigma(Z_s;sleq t))}[X-P_{mathcal{L}(Z,t) }X]=P_{L^2(sigma(Z_s;sleq t))}X-P_{mathcal{L}(Z,t) }X=0,
$$as desired. In your notation, $mathcal{F}_L=sigma(Z_s;sleq t)$ and $P_{L^2(sigma(Z_s;sleq t))}X = E[X|mathcal{F}_L].$
$endgroup$
add a comment |
$begingroup$
As @Kavi Rama Murthy pointed out, it holds that
$$
mathcal{L}(Z,t) neq L^2(sigma(Z_s;sleq t)).
$$ But if we assume additionally that $$(X,Z_s;sleq t)$$
is jointly normally distributed, it holds that
$$
P_{mathcal{L}(Z,t) }X = P_{L^2(sigma(Z_s;sleq t))}X.
$$ To see this, notice that if $(X,Y_1,ldots ,Y_n)$ is jointly normal, then
$$
operatorname{Cov}(X,Y_i) = 0,,forall ileq n Leftrightarrow Xtext{ and }(Y_i)_{ileq n} text{ are independent.}
$$ It holds that
$$
operatorname{Cov}(X-P_{mathcal{L}(Z,t) }X, Z_s) = 0,quadforall sleq t,
$$ by the definition of the projection. And since $(X,Z_s;sleq t)$ is jointly normal, so is $(X-P_{mathcal{L}(Z,t) }X,Z_s;sleq t).$ This implies that
$$
X-P_{mathcal{L}(Z,t) }X perp !!! perp (Z_s)_{sleq t}.
$$ Therefore, we have
$$
P_{L^2(sigma(Z_s;sleq t))}[X-P_{mathcal{L}(Z,t) }X]=P_{L^2(sigma(Z_s;sleq t))}X-P_{mathcal{L}(Z,t) }X=0,
$$as desired. In your notation, $mathcal{F}_L=sigma(Z_s;sleq t)$ and $P_{L^2(sigma(Z_s;sleq t))}X = E[X|mathcal{F}_L].$
$endgroup$
As @Kavi Rama Murthy pointed out, it holds that
$$
mathcal{L}(Z,t) neq L^2(sigma(Z_s;sleq t)).
$$ But if we assume additionally that $$(X,Z_s;sleq t)$$
is jointly normally distributed, it holds that
$$
P_{mathcal{L}(Z,t) }X = P_{L^2(sigma(Z_s;sleq t))}X.
$$ To see this, notice that if $(X,Y_1,ldots ,Y_n)$ is jointly normal, then
$$
operatorname{Cov}(X,Y_i) = 0,,forall ileq n Leftrightarrow Xtext{ and }(Y_i)_{ileq n} text{ are independent.}
$$ It holds that
$$
operatorname{Cov}(X-P_{mathcal{L}(Z,t) }X, Z_s) = 0,quadforall sleq t,
$$ by the definition of the projection. And since $(X,Z_s;sleq t)$ is jointly normal, so is $(X-P_{mathcal{L}(Z,t) }X,Z_s;sleq t).$ This implies that
$$
X-P_{mathcal{L}(Z,t) }X perp !!! perp (Z_s)_{sleq t}.
$$ Therefore, we have
$$
P_{L^2(sigma(Z_s;sleq t))}[X-P_{mathcal{L}(Z,t) }X]=P_{L^2(sigma(Z_s;sleq t))}X-P_{mathcal{L}(Z,t) }X=0,
$$as desired. In your notation, $mathcal{F}_L=sigma(Z_s;sleq t)$ and $P_{L^2(sigma(Z_s;sleq t))}X = E[X|mathcal{F}_L].$
edited Dec 21 '18 at 6:15
answered Dec 21 '18 at 6:08


SongSong
16.7k1941
16.7k1941
add a comment |
add a comment |
$begingroup$
Your argument is not valid. Conditional expectation is the projection on the space of all random variables measurable w.r.t. $sigma {Z_s:sleq t}$, not the projection on to the vector space spanned by $ {Z_s:sleq t}$. The former space contains many nonlinear functions of $ {Z_s:sleq t}$ like $Z_t^{2}$.
$endgroup$
add a comment |
$begingroup$
Your argument is not valid. Conditional expectation is the projection on the space of all random variables measurable w.r.t. $sigma {Z_s:sleq t}$, not the projection on to the vector space spanned by $ {Z_s:sleq t}$. The former space contains many nonlinear functions of $ {Z_s:sleq t}$ like $Z_t^{2}$.
$endgroup$
add a comment |
$begingroup$
Your argument is not valid. Conditional expectation is the projection on the space of all random variables measurable w.r.t. $sigma {Z_s:sleq t}$, not the projection on to the vector space spanned by $ {Z_s:sleq t}$. The former space contains many nonlinear functions of $ {Z_s:sleq t}$ like $Z_t^{2}$.
$endgroup$
Your argument is not valid. Conditional expectation is the projection on the space of all random variables measurable w.r.t. $sigma {Z_s:sleq t}$, not the projection on to the vector space spanned by $ {Z_s:sleq t}$. The former space contains many nonlinear functions of $ {Z_s:sleq t}$ like $Z_t^{2}$.
answered Dec 21 '18 at 5:29


Kavi Rama MurthyKavi Rama Murthy
64.7k42766
64.7k42766
add a comment |
add a comment |
Thanks for contributing an answer to Mathematics Stack Exchange!
- Please be sure to answer the question. Provide details and share your research!
But avoid …
- Asking for help, clarification, or responding to other answers.
- Making statements based on opinion; back them up with references or personal experience.
Use MathJax to format equations. MathJax reference.
To learn more, see our tips on writing great answers.
Sign up or log in
StackExchange.ready(function () {
StackExchange.helpers.onClickDraftSave('#login-link');
});
Sign up using Google
Sign up using Facebook
Sign up using Email and Password
Post as a guest
Required, but never shown
StackExchange.ready(
function () {
StackExchange.openid.initPostLogin('.new-post-login', 'https%3a%2f%2fmath.stackexchange.com%2fquestions%2f3048185%2fexpected-value-and-projection-of-a-normal-random-variable-onto-a-linear-span%23new-answer', 'question_page');
}
);
Post as a guest
Required, but never shown
Sign up or log in
StackExchange.ready(function () {
StackExchange.helpers.onClickDraftSave('#login-link');
});
Sign up using Google
Sign up using Facebook
Sign up using Email and Password
Post as a guest
Required, but never shown
Sign up or log in
StackExchange.ready(function () {
StackExchange.helpers.onClickDraftSave('#login-link');
});
Sign up using Google
Sign up using Facebook
Sign up using Email and Password
Post as a guest
Required, but never shown
Sign up or log in
StackExchange.ready(function () {
StackExchange.helpers.onClickDraftSave('#login-link');
});
Sign up using Google
Sign up using Facebook
Sign up using Email and Password
Sign up using Google
Sign up using Facebook
Sign up using Email and Password
Post as a guest
Required, but never shown
Required, but never shown
Required, but never shown
Required, but never shown
Required, but never shown
Required, but never shown
Required, but never shown
Required, but never shown
Required, but never shown
BG50,jfQuORT QhkGI7T,0D,l0uymD,1adlPnr521
$begingroup$
What proof is this? What source?
$endgroup$
– Zachary Selk
Dec 21 '18 at 5:02
$begingroup$
It is part of Oksander's SDE book lemma 6.2.2
$endgroup$
– Xiaomi
Dec 21 '18 at 5:11