Does ADMM work for nonconvex optimization problems?
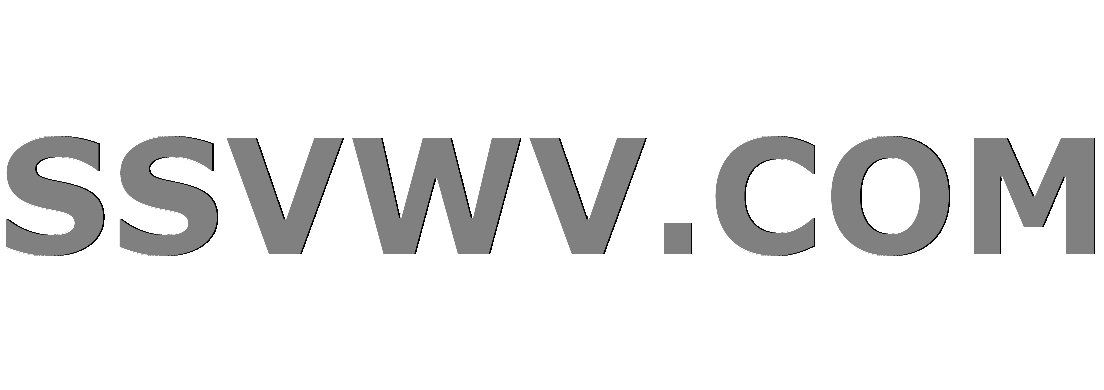
Multi tool use
$begingroup$
I need to solve the following nonconvex optimization problem:
begin{equation}
begin{split}
min_{x,y}quad &f(x)+g(y)\
mathrm{s.t.}quad &Ax+By=b
end{split}
end{equation}
where $f$ is noncovex and $g$ is convex. A natural way is to use ADMM to solve this problem, which can be outlined as follows:
Define the augmented Lagrangian as
$$mathcal{L}_{beta}(x,y;omega)=f(x)+g(y)+w^{T}(Ax+By-b)+frac{beta}{2}||Ax+By-b||_2^2$$ then ADMM repeats as:
Step 1: $x^{k+1}inargmin_{x} mathcal{L}_{beta}(x,y^k;omega^k)$;
Step 2: $y^{k+1}inargmin_{y} mathcal{L}_{beta}(x^{k+1},y;omega^k)$;
Step 1: $omega^{k+1}=omega^{k}+beta(Ax^{k+1}+By^{k+1}-b)$;
As we know, ADMM works for convex optimization problrm with the guarantee of global convergence, but for this nonconvex problem, what's the convergence behavior?
optimization nonlinear-optimization numerical-optimization non-convex-optimization
$endgroup$
add a comment |
$begingroup$
I need to solve the following nonconvex optimization problem:
begin{equation}
begin{split}
min_{x,y}quad &f(x)+g(y)\
mathrm{s.t.}quad &Ax+By=b
end{split}
end{equation}
where $f$ is noncovex and $g$ is convex. A natural way is to use ADMM to solve this problem, which can be outlined as follows:
Define the augmented Lagrangian as
$$mathcal{L}_{beta}(x,y;omega)=f(x)+g(y)+w^{T}(Ax+By-b)+frac{beta}{2}||Ax+By-b||_2^2$$ then ADMM repeats as:
Step 1: $x^{k+1}inargmin_{x} mathcal{L}_{beta}(x,y^k;omega^k)$;
Step 2: $y^{k+1}inargmin_{y} mathcal{L}_{beta}(x^{k+1},y;omega^k)$;
Step 1: $omega^{k+1}=omega^{k}+beta(Ax^{k+1}+By^{k+1}-b)$;
As we know, ADMM works for convex optimization problrm with the guarantee of global convergence, but for this nonconvex problem, what's the convergence behavior?
optimization nonlinear-optimization numerical-optimization non-convex-optimization
$endgroup$
add a comment |
$begingroup$
I need to solve the following nonconvex optimization problem:
begin{equation}
begin{split}
min_{x,y}quad &f(x)+g(y)\
mathrm{s.t.}quad &Ax+By=b
end{split}
end{equation}
where $f$ is noncovex and $g$ is convex. A natural way is to use ADMM to solve this problem, which can be outlined as follows:
Define the augmented Lagrangian as
$$mathcal{L}_{beta}(x,y;omega)=f(x)+g(y)+w^{T}(Ax+By-b)+frac{beta}{2}||Ax+By-b||_2^2$$ then ADMM repeats as:
Step 1: $x^{k+1}inargmin_{x} mathcal{L}_{beta}(x,y^k;omega^k)$;
Step 2: $y^{k+1}inargmin_{y} mathcal{L}_{beta}(x^{k+1},y;omega^k)$;
Step 1: $omega^{k+1}=omega^{k}+beta(Ax^{k+1}+By^{k+1}-b)$;
As we know, ADMM works for convex optimization problrm with the guarantee of global convergence, but for this nonconvex problem, what's the convergence behavior?
optimization nonlinear-optimization numerical-optimization non-convex-optimization
$endgroup$
I need to solve the following nonconvex optimization problem:
begin{equation}
begin{split}
min_{x,y}quad &f(x)+g(y)\
mathrm{s.t.}quad &Ax+By=b
end{split}
end{equation}
where $f$ is noncovex and $g$ is convex. A natural way is to use ADMM to solve this problem, which can be outlined as follows:
Define the augmented Lagrangian as
$$mathcal{L}_{beta}(x,y;omega)=f(x)+g(y)+w^{T}(Ax+By-b)+frac{beta}{2}||Ax+By-b||_2^2$$ then ADMM repeats as:
Step 1: $x^{k+1}inargmin_{x} mathcal{L}_{beta}(x,y^k;omega^k)$;
Step 2: $y^{k+1}inargmin_{y} mathcal{L}_{beta}(x^{k+1},y;omega^k)$;
Step 1: $omega^{k+1}=omega^{k}+beta(Ax^{k+1}+By^{k+1}-b)$;
As we know, ADMM works for convex optimization problrm with the guarantee of global convergence, but for this nonconvex problem, what's the convergence behavior?
optimization nonlinear-optimization numerical-optimization non-convex-optimization
optimization nonlinear-optimization numerical-optimization non-convex-optimization
edited Dec 16 '18 at 18:48
Rodrigo de Azevedo
13k41958
13k41958
asked Dec 15 '18 at 4:06
ChenflChenfl
215
215
add a comment |
add a comment |
1 Answer
1
active
oldest
votes
$begingroup$
In general, the convergence behavior can be arbitrarily bad. But, it all depends on the structure of $f(x)$. If you can find nice convex envelopes of the $f(x)$ you can get numerical bounds on the convergence. E.g., if $f(x)$ is bilinear, like $f(x)=x_1 x_2$. McCormick's relaxations provide envelopes https://optimization.mccormick.northwestern.edu/index.php/McCormick_envelopes
I would recommend finding convex envelopes to $f(x)$. Solving the relaxations like you would solve convex problems. Then evaluating the actual objective function at feasible points close to the solution of the enveloped functions.
$endgroup$
add a comment |
Your Answer
StackExchange.ifUsing("editor", function () {
return StackExchange.using("mathjaxEditing", function () {
StackExchange.MarkdownEditor.creationCallbacks.add(function (editor, postfix) {
StackExchange.mathjaxEditing.prepareWmdForMathJax(editor, postfix, [["$", "$"], ["\\(","\\)"]]);
});
});
}, "mathjax-editing");
StackExchange.ready(function() {
var channelOptions = {
tags: "".split(" "),
id: "69"
};
initTagRenderer("".split(" "), "".split(" "), channelOptions);
StackExchange.using("externalEditor", function() {
// Have to fire editor after snippets, if snippets enabled
if (StackExchange.settings.snippets.snippetsEnabled) {
StackExchange.using("snippets", function() {
createEditor();
});
}
else {
createEditor();
}
});
function createEditor() {
StackExchange.prepareEditor({
heartbeatType: 'answer',
autoActivateHeartbeat: false,
convertImagesToLinks: true,
noModals: true,
showLowRepImageUploadWarning: true,
reputationToPostImages: 10,
bindNavPrevention: true,
postfix: "",
imageUploader: {
brandingHtml: "Powered by u003ca class="icon-imgur-white" href="https://imgur.com/"u003eu003c/au003e",
contentPolicyHtml: "User contributions licensed under u003ca href="https://creativecommons.org/licenses/by-sa/3.0/"u003ecc by-sa 3.0 with attribution requiredu003c/au003e u003ca href="https://stackoverflow.com/legal/content-policy"u003e(content policy)u003c/au003e",
allowUrls: true
},
noCode: true, onDemand: true,
discardSelector: ".discard-answer"
,immediatelyShowMarkdownHelp:true
});
}
});
Sign up or log in
StackExchange.ready(function () {
StackExchange.helpers.onClickDraftSave('#login-link');
});
Sign up using Google
Sign up using Facebook
Sign up using Email and Password
Post as a guest
Required, but never shown
StackExchange.ready(
function () {
StackExchange.openid.initPostLogin('.new-post-login', 'https%3a%2f%2fmath.stackexchange.com%2fquestions%2f3040157%2fdoes-admm-work-for-nonconvex-optimization-problems%23new-answer', 'question_page');
}
);
Post as a guest
Required, but never shown
1 Answer
1
active
oldest
votes
1 Answer
1
active
oldest
votes
active
oldest
votes
active
oldest
votes
$begingroup$
In general, the convergence behavior can be arbitrarily bad. But, it all depends on the structure of $f(x)$. If you can find nice convex envelopes of the $f(x)$ you can get numerical bounds on the convergence. E.g., if $f(x)$ is bilinear, like $f(x)=x_1 x_2$. McCormick's relaxations provide envelopes https://optimization.mccormick.northwestern.edu/index.php/McCormick_envelopes
I would recommend finding convex envelopes to $f(x)$. Solving the relaxations like you would solve convex problems. Then evaluating the actual objective function at feasible points close to the solution of the enveloped functions.
$endgroup$
add a comment |
$begingroup$
In general, the convergence behavior can be arbitrarily bad. But, it all depends on the structure of $f(x)$. If you can find nice convex envelopes of the $f(x)$ you can get numerical bounds on the convergence. E.g., if $f(x)$ is bilinear, like $f(x)=x_1 x_2$. McCormick's relaxations provide envelopes https://optimization.mccormick.northwestern.edu/index.php/McCormick_envelopes
I would recommend finding convex envelopes to $f(x)$. Solving the relaxations like you would solve convex problems. Then evaluating the actual objective function at feasible points close to the solution of the enveloped functions.
$endgroup$
add a comment |
$begingroup$
In general, the convergence behavior can be arbitrarily bad. But, it all depends on the structure of $f(x)$. If you can find nice convex envelopes of the $f(x)$ you can get numerical bounds on the convergence. E.g., if $f(x)$ is bilinear, like $f(x)=x_1 x_2$. McCormick's relaxations provide envelopes https://optimization.mccormick.northwestern.edu/index.php/McCormick_envelopes
I would recommend finding convex envelopes to $f(x)$. Solving the relaxations like you would solve convex problems. Then evaluating the actual objective function at feasible points close to the solution of the enveloped functions.
$endgroup$
In general, the convergence behavior can be arbitrarily bad. But, it all depends on the structure of $f(x)$. If you can find nice convex envelopes of the $f(x)$ you can get numerical bounds on the convergence. E.g., if $f(x)$ is bilinear, like $f(x)=x_1 x_2$. McCormick's relaxations provide envelopes https://optimization.mccormick.northwestern.edu/index.php/McCormick_envelopes
I would recommend finding convex envelopes to $f(x)$. Solving the relaxations like you would solve convex problems. Then evaluating the actual objective function at feasible points close to the solution of the enveloped functions.
answered Dec 16 '18 at 18:13
skrskr
17411
17411
add a comment |
add a comment |
Thanks for contributing an answer to Mathematics Stack Exchange!
- Please be sure to answer the question. Provide details and share your research!
But avoid …
- Asking for help, clarification, or responding to other answers.
- Making statements based on opinion; back them up with references or personal experience.
Use MathJax to format equations. MathJax reference.
To learn more, see our tips on writing great answers.
Sign up or log in
StackExchange.ready(function () {
StackExchange.helpers.onClickDraftSave('#login-link');
});
Sign up using Google
Sign up using Facebook
Sign up using Email and Password
Post as a guest
Required, but never shown
StackExchange.ready(
function () {
StackExchange.openid.initPostLogin('.new-post-login', 'https%3a%2f%2fmath.stackexchange.com%2fquestions%2f3040157%2fdoes-admm-work-for-nonconvex-optimization-problems%23new-answer', 'question_page');
}
);
Post as a guest
Required, but never shown
Sign up or log in
StackExchange.ready(function () {
StackExchange.helpers.onClickDraftSave('#login-link');
});
Sign up using Google
Sign up using Facebook
Sign up using Email and Password
Post as a guest
Required, but never shown
Sign up or log in
StackExchange.ready(function () {
StackExchange.helpers.onClickDraftSave('#login-link');
});
Sign up using Google
Sign up using Facebook
Sign up using Email and Password
Post as a guest
Required, but never shown
Sign up or log in
StackExchange.ready(function () {
StackExchange.helpers.onClickDraftSave('#login-link');
});
Sign up using Google
Sign up using Facebook
Sign up using Email and Password
Sign up using Google
Sign up using Facebook
Sign up using Email and Password
Post as a guest
Required, but never shown
Required, but never shown
Required, but never shown
Required, but never shown
Required, but never shown
Required, but never shown
Required, but never shown
Required, but never shown
Required, but never shown
Ya,wTNfomsdOm4kYdKqDAZC a8dc,no