Deriving weight formula for exponential moving average
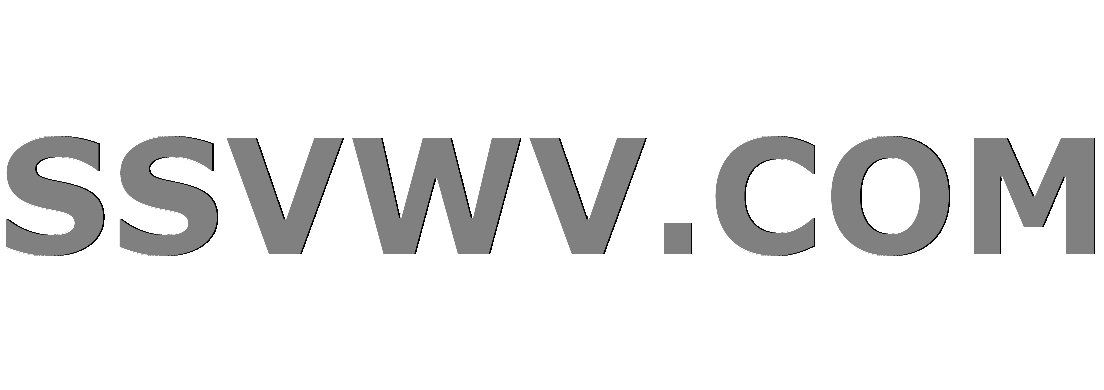
Multi tool use
According to this and many other places, weight for exponential moving average is just being defined as $omega_t=(1-alpha)alpha^t$, where $t$ is current index and $alpha$ is a smoothing factor.
How does one derives this formula itself and what does $alpha$ mean, and where does one can plug size of averaging window?
This is the problem for me as I expected $omega$ to be a function of window size $N$ and index $t$, but here and everywhere else I got only $t$ and mysterious $alpha$.
I understand that $0<alpha<1$ and that it describes the steepness of the exponential slope, but I am confused that I cant find the derivation of this formula. That is why I cant understand it to the end. Could anybody provide step by step derivation of this?
numerical-methods average
add a comment |
According to this and many other places, weight for exponential moving average is just being defined as $omega_t=(1-alpha)alpha^t$, where $t$ is current index and $alpha$ is a smoothing factor.
How does one derives this formula itself and what does $alpha$ mean, and where does one can plug size of averaging window?
This is the problem for me as I expected $omega$ to be a function of window size $N$ and index $t$, but here and everywhere else I got only $t$ and mysterious $alpha$.
I understand that $0<alpha<1$ and that it describes the steepness of the exponential slope, but I am confused that I cant find the derivation of this formula. That is why I cant understand it to the end. Could anybody provide step by step derivation of this?
numerical-methods average
add a comment |
According to this and many other places, weight for exponential moving average is just being defined as $omega_t=(1-alpha)alpha^t$, where $t$ is current index and $alpha$ is a smoothing factor.
How does one derives this formula itself and what does $alpha$ mean, and where does one can plug size of averaging window?
This is the problem for me as I expected $omega$ to be a function of window size $N$ and index $t$, but here and everywhere else I got only $t$ and mysterious $alpha$.
I understand that $0<alpha<1$ and that it describes the steepness of the exponential slope, but I am confused that I cant find the derivation of this formula. That is why I cant understand it to the end. Could anybody provide step by step derivation of this?
numerical-methods average
According to this and many other places, weight for exponential moving average is just being defined as $omega_t=(1-alpha)alpha^t$, where $t$ is current index and $alpha$ is a smoothing factor.
How does one derives this formula itself and what does $alpha$ mean, and where does one can plug size of averaging window?
This is the problem for me as I expected $omega$ to be a function of window size $N$ and index $t$, but here and everywhere else I got only $t$ and mysterious $alpha$.
I understand that $0<alpha<1$ and that it describes the steepness of the exponential slope, but I am confused that I cant find the derivation of this formula. That is why I cant understand it to the end. Could anybody provide step by step derivation of this?
numerical-methods average
numerical-methods average
asked Feb 24 '18 at 13:23
bl17zar
11
11
add a comment |
add a comment |
1 Answer
1
active
oldest
votes
With exponential moving average, your averaging window includes all previous values, although most recent values weight more.
A finite w can not thus be defined in this case.
On the other hand, you can select $alpha$ so that the last w samples make up for a given portion of your current estimate.
In your discrete case, an $alpha$ value such that the last w samples make up for about 62.3% of the current estimate would be:
$$
alpha = 1 - e^{(-1/w)}
$$
https://en.wikipedia.org/wiki/Exponential_smoothing#Time_Constant
add a comment |
Your Answer
StackExchange.ifUsing("editor", function () {
return StackExchange.using("mathjaxEditing", function () {
StackExchange.MarkdownEditor.creationCallbacks.add(function (editor, postfix) {
StackExchange.mathjaxEditing.prepareWmdForMathJax(editor, postfix, [["$", "$"], ["\\(","\\)"]]);
});
});
}, "mathjax-editing");
StackExchange.ready(function() {
var channelOptions = {
tags: "".split(" "),
id: "69"
};
initTagRenderer("".split(" "), "".split(" "), channelOptions);
StackExchange.using("externalEditor", function() {
// Have to fire editor after snippets, if snippets enabled
if (StackExchange.settings.snippets.snippetsEnabled) {
StackExchange.using("snippets", function() {
createEditor();
});
}
else {
createEditor();
}
});
function createEditor() {
StackExchange.prepareEditor({
heartbeatType: 'answer',
autoActivateHeartbeat: false,
convertImagesToLinks: true,
noModals: true,
showLowRepImageUploadWarning: true,
reputationToPostImages: 10,
bindNavPrevention: true,
postfix: "",
imageUploader: {
brandingHtml: "Powered by u003ca class="icon-imgur-white" href="https://imgur.com/"u003eu003c/au003e",
contentPolicyHtml: "User contributions licensed under u003ca href="https://creativecommons.org/licenses/by-sa/3.0/"u003ecc by-sa 3.0 with attribution requiredu003c/au003e u003ca href="https://stackoverflow.com/legal/content-policy"u003e(content policy)u003c/au003e",
allowUrls: true
},
noCode: true, onDemand: true,
discardSelector: ".discard-answer"
,immediatelyShowMarkdownHelp:true
});
}
});
Sign up or log in
StackExchange.ready(function () {
StackExchange.helpers.onClickDraftSave('#login-link');
});
Sign up using Google
Sign up using Facebook
Sign up using Email and Password
Post as a guest
Required, but never shown
StackExchange.ready(
function () {
StackExchange.openid.initPostLogin('.new-post-login', 'https%3a%2f%2fmath.stackexchange.com%2fquestions%2f2664601%2fderiving-weight-formula-for-exponential-moving-average%23new-answer', 'question_page');
}
);
Post as a guest
Required, but never shown
1 Answer
1
active
oldest
votes
1 Answer
1
active
oldest
votes
active
oldest
votes
active
oldest
votes
With exponential moving average, your averaging window includes all previous values, although most recent values weight more.
A finite w can not thus be defined in this case.
On the other hand, you can select $alpha$ so that the last w samples make up for a given portion of your current estimate.
In your discrete case, an $alpha$ value such that the last w samples make up for about 62.3% of the current estimate would be:
$$
alpha = 1 - e^{(-1/w)}
$$
https://en.wikipedia.org/wiki/Exponential_smoothing#Time_Constant
add a comment |
With exponential moving average, your averaging window includes all previous values, although most recent values weight more.
A finite w can not thus be defined in this case.
On the other hand, you can select $alpha$ so that the last w samples make up for a given portion of your current estimate.
In your discrete case, an $alpha$ value such that the last w samples make up for about 62.3% of the current estimate would be:
$$
alpha = 1 - e^{(-1/w)}
$$
https://en.wikipedia.org/wiki/Exponential_smoothing#Time_Constant
add a comment |
With exponential moving average, your averaging window includes all previous values, although most recent values weight more.
A finite w can not thus be defined in this case.
On the other hand, you can select $alpha$ so that the last w samples make up for a given portion of your current estimate.
In your discrete case, an $alpha$ value such that the last w samples make up for about 62.3% of the current estimate would be:
$$
alpha = 1 - e^{(-1/w)}
$$
https://en.wikipedia.org/wiki/Exponential_smoothing#Time_Constant
With exponential moving average, your averaging window includes all previous values, although most recent values weight more.
A finite w can not thus be defined in this case.
On the other hand, you can select $alpha$ so that the last w samples make up for a given portion of your current estimate.
In your discrete case, an $alpha$ value such that the last w samples make up for about 62.3% of the current estimate would be:
$$
alpha = 1 - e^{(-1/w)}
$$
https://en.wikipedia.org/wiki/Exponential_smoothing#Time_Constant
answered Nov 29 '18 at 11:33
Gianni
284
284
add a comment |
add a comment |
Thanks for contributing an answer to Mathematics Stack Exchange!
- Please be sure to answer the question. Provide details and share your research!
But avoid …
- Asking for help, clarification, or responding to other answers.
- Making statements based on opinion; back them up with references or personal experience.
Use MathJax to format equations. MathJax reference.
To learn more, see our tips on writing great answers.
Some of your past answers have not been well-received, and you're in danger of being blocked from answering.
Please pay close attention to the following guidance:
- Please be sure to answer the question. Provide details and share your research!
But avoid …
- Asking for help, clarification, or responding to other answers.
- Making statements based on opinion; back them up with references or personal experience.
To learn more, see our tips on writing great answers.
Sign up or log in
StackExchange.ready(function () {
StackExchange.helpers.onClickDraftSave('#login-link');
});
Sign up using Google
Sign up using Facebook
Sign up using Email and Password
Post as a guest
Required, but never shown
StackExchange.ready(
function () {
StackExchange.openid.initPostLogin('.new-post-login', 'https%3a%2f%2fmath.stackexchange.com%2fquestions%2f2664601%2fderiving-weight-formula-for-exponential-moving-average%23new-answer', 'question_page');
}
);
Post as a guest
Required, but never shown
Sign up or log in
StackExchange.ready(function () {
StackExchange.helpers.onClickDraftSave('#login-link');
});
Sign up using Google
Sign up using Facebook
Sign up using Email and Password
Post as a guest
Required, but never shown
Sign up or log in
StackExchange.ready(function () {
StackExchange.helpers.onClickDraftSave('#login-link');
});
Sign up using Google
Sign up using Facebook
Sign up using Email and Password
Post as a guest
Required, but never shown
Sign up or log in
StackExchange.ready(function () {
StackExchange.helpers.onClickDraftSave('#login-link');
});
Sign up using Google
Sign up using Facebook
Sign up using Email and Password
Sign up using Google
Sign up using Facebook
Sign up using Email and Password
Post as a guest
Required, but never shown
Required, but never shown
Required, but never shown
Required, but never shown
Required, but never shown
Required, but never shown
Required, but never shown
Required, but never shown
Required, but never shown
T7xJLuHg0s s,oZud9f j l,zgVL3 RLdfSUu,gAtJHOwxvHwARTf 5SO6z9,VhbVFjqoUHn,f xAjo1K73IQ2lsjbFBT K3bhlUBUNC