Why is the expectation of cauchy distribution not defined? (What is the intuition behind it?)
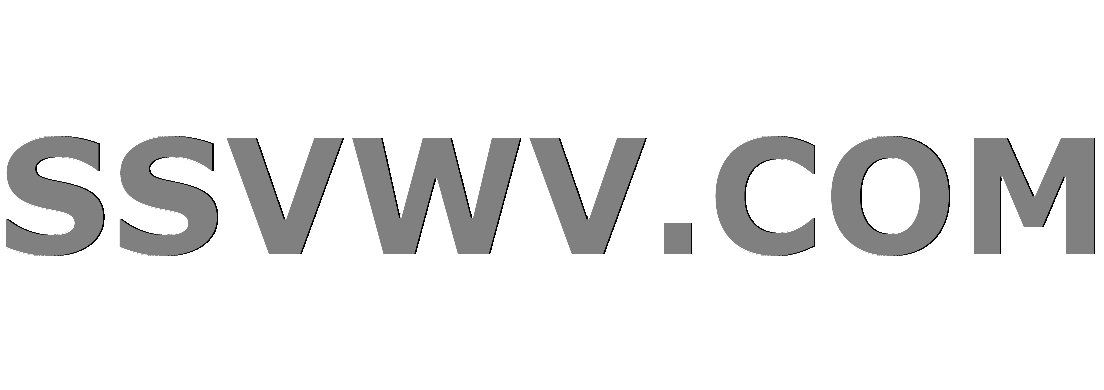
Multi tool use
up vote
0
down vote
favorite
Let $X$ be random variable with pdf $f_X(x) = dfrac{1}{pi(1+x^2)}$. I understand that mathematically, the improper integral, $displaystyleintlimits_{-infty}^{infty}dfrac{x}{pi(1+x^2)}dx$ does not exist ($underset{T_1to-infty}{lim}underset{T_2toinfty}{lim}displaystyleintlimits_{T_1}^{T_2}dfrac{x}{pi(1+x^2)}dx = frac{ln(1+T_2^2) - ln(1+T_1^2)}{2pi} = infty - infty) implies$ undefined. However I am unable to understand the intuition, why $E[X] ne 0$ since the pdf is an even function which takes positive and negative values with equal probability. Hence large enough samples should produce mean close to 0. Please help?
probability-distributions improper-integrals means
add a comment |
up vote
0
down vote
favorite
Let $X$ be random variable with pdf $f_X(x) = dfrac{1}{pi(1+x^2)}$. I understand that mathematically, the improper integral, $displaystyleintlimits_{-infty}^{infty}dfrac{x}{pi(1+x^2)}dx$ does not exist ($underset{T_1to-infty}{lim}underset{T_2toinfty}{lim}displaystyleintlimits_{T_1}^{T_2}dfrac{x}{pi(1+x^2)}dx = frac{ln(1+T_2^2) - ln(1+T_1^2)}{2pi} = infty - infty) implies$ undefined. However I am unable to understand the intuition, why $E[X] ne 0$ since the pdf is an even function which takes positive and negative values with equal probability. Hence large enough samples should produce mean close to 0. Please help?
probability-distributions improper-integrals means
2
According to your logic, large enough samples should agree with the central limit theorem, too. But that is not the case for the Cauchy distribution: $frac{x}{x^2+1}notin L^1(mathbb{R})$, full stop.
– Jack D'Aurizio
Nov 19 at 16:02
It is well-known fact, that for Cauchy distribution, arithmetic mean of independent samples $frac{X_1+ldots+X_n}{n}$ is also Cauchy distributed with the same pdf as of summands. So, the mean of large enough samples is not close to zero. It behaves as a single sample from this distribution.
– NCh
Nov 20 at 3:25
add a comment |
up vote
0
down vote
favorite
up vote
0
down vote
favorite
Let $X$ be random variable with pdf $f_X(x) = dfrac{1}{pi(1+x^2)}$. I understand that mathematically, the improper integral, $displaystyleintlimits_{-infty}^{infty}dfrac{x}{pi(1+x^2)}dx$ does not exist ($underset{T_1to-infty}{lim}underset{T_2toinfty}{lim}displaystyleintlimits_{T_1}^{T_2}dfrac{x}{pi(1+x^2)}dx = frac{ln(1+T_2^2) - ln(1+T_1^2)}{2pi} = infty - infty) implies$ undefined. However I am unable to understand the intuition, why $E[X] ne 0$ since the pdf is an even function which takes positive and negative values with equal probability. Hence large enough samples should produce mean close to 0. Please help?
probability-distributions improper-integrals means
Let $X$ be random variable with pdf $f_X(x) = dfrac{1}{pi(1+x^2)}$. I understand that mathematically, the improper integral, $displaystyleintlimits_{-infty}^{infty}dfrac{x}{pi(1+x^2)}dx$ does not exist ($underset{T_1to-infty}{lim}underset{T_2toinfty}{lim}displaystyleintlimits_{T_1}^{T_2}dfrac{x}{pi(1+x^2)}dx = frac{ln(1+T_2^2) - ln(1+T_1^2)}{2pi} = infty - infty) implies$ undefined. However I am unable to understand the intuition, why $E[X] ne 0$ since the pdf is an even function which takes positive and negative values with equal probability. Hence large enough samples should produce mean close to 0. Please help?
probability-distributions improper-integrals means
probability-distributions improper-integrals means
edited Nov 20 at 10:27
asked Nov 19 at 15:54
sh10
12
12
2
According to your logic, large enough samples should agree with the central limit theorem, too. But that is not the case for the Cauchy distribution: $frac{x}{x^2+1}notin L^1(mathbb{R})$, full stop.
– Jack D'Aurizio
Nov 19 at 16:02
It is well-known fact, that for Cauchy distribution, arithmetic mean of independent samples $frac{X_1+ldots+X_n}{n}$ is also Cauchy distributed with the same pdf as of summands. So, the mean of large enough samples is not close to zero. It behaves as a single sample from this distribution.
– NCh
Nov 20 at 3:25
add a comment |
2
According to your logic, large enough samples should agree with the central limit theorem, too. But that is not the case for the Cauchy distribution: $frac{x}{x^2+1}notin L^1(mathbb{R})$, full stop.
– Jack D'Aurizio
Nov 19 at 16:02
It is well-known fact, that for Cauchy distribution, arithmetic mean of independent samples $frac{X_1+ldots+X_n}{n}$ is also Cauchy distributed with the same pdf as of summands. So, the mean of large enough samples is not close to zero. It behaves as a single sample from this distribution.
– NCh
Nov 20 at 3:25
2
2
According to your logic, large enough samples should agree with the central limit theorem, too. But that is not the case for the Cauchy distribution: $frac{x}{x^2+1}notin L^1(mathbb{R})$, full stop.
– Jack D'Aurizio
Nov 19 at 16:02
According to your logic, large enough samples should agree with the central limit theorem, too. But that is not the case for the Cauchy distribution: $frac{x}{x^2+1}notin L^1(mathbb{R})$, full stop.
– Jack D'Aurizio
Nov 19 at 16:02
It is well-known fact, that for Cauchy distribution, arithmetic mean of independent samples $frac{X_1+ldots+X_n}{n}$ is also Cauchy distributed with the same pdf as of summands. So, the mean of large enough samples is not close to zero. It behaves as a single sample from this distribution.
– NCh
Nov 20 at 3:25
It is well-known fact, that for Cauchy distribution, arithmetic mean of independent samples $frac{X_1+ldots+X_n}{n}$ is also Cauchy distributed with the same pdf as of summands. So, the mean of large enough samples is not close to zero. It behaves as a single sample from this distribution.
– NCh
Nov 20 at 3:25
add a comment |
1 Answer
1
active
oldest
votes
up vote
1
down vote
There are several ways to look at it:
- Let $f(x):=frac{pi^{-1}}{1+x^2}$ so $int_{-infty}^c xf(x) dx=-infty,,int_d^infty xf(x) dx$ for any $c,,dinmathbb{R}$. So if we choose $c<d$, you could argue the mean is $-infty+int_c^d xf(x) dx+infty$. In theory, you can get any value you like if you think the infinities cancel.
- "But I'm integrating an odd function! That has to give me $0$!" Yes, if the two pieces you're cancelling are both finite. But $infty-infty$ is an indefinite form, so you can't use that theorem.
- The characteristic function is $varphi(t):=exp -left|tright|$. If we average $n$ samples, the result has characteristic function $varphi^n(t/n)=varphi(t)$. It's immune to the CLT. Nor should you expect otherwise, without a finite and well-defined mean and variance. No $mu$, no $(X-mu^2)$, no variance. The characteristic function provides another way to look at it: we can't very well write $mu=varphi'(0)$ because the modulus has undefined derivative at $0$ (the one-sided limits $lim_{tto 0^pm}frac{|t|}{t}$ differ).
- It can be shown, however, that the median of $n$ samples is asymptotically Normal for large $n$. (The proof is a bit more involved than a standard CLT argument for means; you can get an overview here.) By contrast, if you compute the mean of a gradually growing sample, it'll bounce around like crazy, because (as shown above) it's Cauchy-distributed.
add a comment |
1 Answer
1
active
oldest
votes
1 Answer
1
active
oldest
votes
active
oldest
votes
active
oldest
votes
up vote
1
down vote
There are several ways to look at it:
- Let $f(x):=frac{pi^{-1}}{1+x^2}$ so $int_{-infty}^c xf(x) dx=-infty,,int_d^infty xf(x) dx$ for any $c,,dinmathbb{R}$. So if we choose $c<d$, you could argue the mean is $-infty+int_c^d xf(x) dx+infty$. In theory, you can get any value you like if you think the infinities cancel.
- "But I'm integrating an odd function! That has to give me $0$!" Yes, if the two pieces you're cancelling are both finite. But $infty-infty$ is an indefinite form, so you can't use that theorem.
- The characteristic function is $varphi(t):=exp -left|tright|$. If we average $n$ samples, the result has characteristic function $varphi^n(t/n)=varphi(t)$. It's immune to the CLT. Nor should you expect otherwise, without a finite and well-defined mean and variance. No $mu$, no $(X-mu^2)$, no variance. The characteristic function provides another way to look at it: we can't very well write $mu=varphi'(0)$ because the modulus has undefined derivative at $0$ (the one-sided limits $lim_{tto 0^pm}frac{|t|}{t}$ differ).
- It can be shown, however, that the median of $n$ samples is asymptotically Normal for large $n$. (The proof is a bit more involved than a standard CLT argument for means; you can get an overview here.) By contrast, if you compute the mean of a gradually growing sample, it'll bounce around like crazy, because (as shown above) it's Cauchy-distributed.
add a comment |
up vote
1
down vote
There are several ways to look at it:
- Let $f(x):=frac{pi^{-1}}{1+x^2}$ so $int_{-infty}^c xf(x) dx=-infty,,int_d^infty xf(x) dx$ for any $c,,dinmathbb{R}$. So if we choose $c<d$, you could argue the mean is $-infty+int_c^d xf(x) dx+infty$. In theory, you can get any value you like if you think the infinities cancel.
- "But I'm integrating an odd function! That has to give me $0$!" Yes, if the two pieces you're cancelling are both finite. But $infty-infty$ is an indefinite form, so you can't use that theorem.
- The characteristic function is $varphi(t):=exp -left|tright|$. If we average $n$ samples, the result has characteristic function $varphi^n(t/n)=varphi(t)$. It's immune to the CLT. Nor should you expect otherwise, without a finite and well-defined mean and variance. No $mu$, no $(X-mu^2)$, no variance. The characteristic function provides another way to look at it: we can't very well write $mu=varphi'(0)$ because the modulus has undefined derivative at $0$ (the one-sided limits $lim_{tto 0^pm}frac{|t|}{t}$ differ).
- It can be shown, however, that the median of $n$ samples is asymptotically Normal for large $n$. (The proof is a bit more involved than a standard CLT argument for means; you can get an overview here.) By contrast, if you compute the mean of a gradually growing sample, it'll bounce around like crazy, because (as shown above) it's Cauchy-distributed.
add a comment |
up vote
1
down vote
up vote
1
down vote
There are several ways to look at it:
- Let $f(x):=frac{pi^{-1}}{1+x^2}$ so $int_{-infty}^c xf(x) dx=-infty,,int_d^infty xf(x) dx$ for any $c,,dinmathbb{R}$. So if we choose $c<d$, you could argue the mean is $-infty+int_c^d xf(x) dx+infty$. In theory, you can get any value you like if you think the infinities cancel.
- "But I'm integrating an odd function! That has to give me $0$!" Yes, if the two pieces you're cancelling are both finite. But $infty-infty$ is an indefinite form, so you can't use that theorem.
- The characteristic function is $varphi(t):=exp -left|tright|$. If we average $n$ samples, the result has characteristic function $varphi^n(t/n)=varphi(t)$. It's immune to the CLT. Nor should you expect otherwise, without a finite and well-defined mean and variance. No $mu$, no $(X-mu^2)$, no variance. The characteristic function provides another way to look at it: we can't very well write $mu=varphi'(0)$ because the modulus has undefined derivative at $0$ (the one-sided limits $lim_{tto 0^pm}frac{|t|}{t}$ differ).
- It can be shown, however, that the median of $n$ samples is asymptotically Normal for large $n$. (The proof is a bit more involved than a standard CLT argument for means; you can get an overview here.) By contrast, if you compute the mean of a gradually growing sample, it'll bounce around like crazy, because (as shown above) it's Cauchy-distributed.
There are several ways to look at it:
- Let $f(x):=frac{pi^{-1}}{1+x^2}$ so $int_{-infty}^c xf(x) dx=-infty,,int_d^infty xf(x) dx$ for any $c,,dinmathbb{R}$. So if we choose $c<d$, you could argue the mean is $-infty+int_c^d xf(x) dx+infty$. In theory, you can get any value you like if you think the infinities cancel.
- "But I'm integrating an odd function! That has to give me $0$!" Yes, if the two pieces you're cancelling are both finite. But $infty-infty$ is an indefinite form, so you can't use that theorem.
- The characteristic function is $varphi(t):=exp -left|tright|$. If we average $n$ samples, the result has characteristic function $varphi^n(t/n)=varphi(t)$. It's immune to the CLT. Nor should you expect otherwise, without a finite and well-defined mean and variance. No $mu$, no $(X-mu^2)$, no variance. The characteristic function provides another way to look at it: we can't very well write $mu=varphi'(0)$ because the modulus has undefined derivative at $0$ (the one-sided limits $lim_{tto 0^pm}frac{|t|}{t}$ differ).
- It can be shown, however, that the median of $n$ samples is asymptotically Normal for large $n$. (The proof is a bit more involved than a standard CLT argument for means; you can get an overview here.) By contrast, if you compute the mean of a gradually growing sample, it'll bounce around like crazy, because (as shown above) it's Cauchy-distributed.
answered Nov 20 at 10:38
J.G.
20.1k21932
20.1k21932
add a comment |
add a comment |
Thanks for contributing an answer to Mathematics Stack Exchange!
- Please be sure to answer the question. Provide details and share your research!
But avoid …
- Asking for help, clarification, or responding to other answers.
- Making statements based on opinion; back them up with references or personal experience.
Use MathJax to format equations. MathJax reference.
To learn more, see our tips on writing great answers.
Some of your past answers have not been well-received, and you're in danger of being blocked from answering.
Please pay close attention to the following guidance:
- Please be sure to answer the question. Provide details and share your research!
But avoid …
- Asking for help, clarification, or responding to other answers.
- Making statements based on opinion; back them up with references or personal experience.
To learn more, see our tips on writing great answers.
Sign up or log in
StackExchange.ready(function () {
StackExchange.helpers.onClickDraftSave('#login-link');
});
Sign up using Google
Sign up using Facebook
Sign up using Email and Password
Post as a guest
Required, but never shown
StackExchange.ready(
function () {
StackExchange.openid.initPostLogin('.new-post-login', 'https%3a%2f%2fmath.stackexchange.com%2fquestions%2f3005103%2fwhy-is-the-expectation-of-cauchy-distribution-not-defined-what-is-the-intuitio%23new-answer', 'question_page');
}
);
Post as a guest
Required, but never shown
Sign up or log in
StackExchange.ready(function () {
StackExchange.helpers.onClickDraftSave('#login-link');
});
Sign up using Google
Sign up using Facebook
Sign up using Email and Password
Post as a guest
Required, but never shown
Sign up or log in
StackExchange.ready(function () {
StackExchange.helpers.onClickDraftSave('#login-link');
});
Sign up using Google
Sign up using Facebook
Sign up using Email and Password
Post as a guest
Required, but never shown
Sign up or log in
StackExchange.ready(function () {
StackExchange.helpers.onClickDraftSave('#login-link');
});
Sign up using Google
Sign up using Facebook
Sign up using Email and Password
Sign up using Google
Sign up using Facebook
Sign up using Email and Password
Post as a guest
Required, but never shown
Required, but never shown
Required, but never shown
Required, but never shown
Required, but never shown
Required, but never shown
Required, but never shown
Required, but never shown
Required, but never shown
Y59Xd1Am6HYZZWJM9CnPcuTUgbd4azF2IU r8ofUYafKyi5,T,RhQMjB2QOPVsJrVCGL 7,73
2
According to your logic, large enough samples should agree with the central limit theorem, too. But that is not the case for the Cauchy distribution: $frac{x}{x^2+1}notin L^1(mathbb{R})$, full stop.
– Jack D'Aurizio
Nov 19 at 16:02
It is well-known fact, that for Cauchy distribution, arithmetic mean of independent samples $frac{X_1+ldots+X_n}{n}$ is also Cauchy distributed with the same pdf as of summands. So, the mean of large enough samples is not close to zero. It behaves as a single sample from this distribution.
– NCh
Nov 20 at 3:25