Finding a conditional probabilty by conditioning on geometric rv [duplicate]
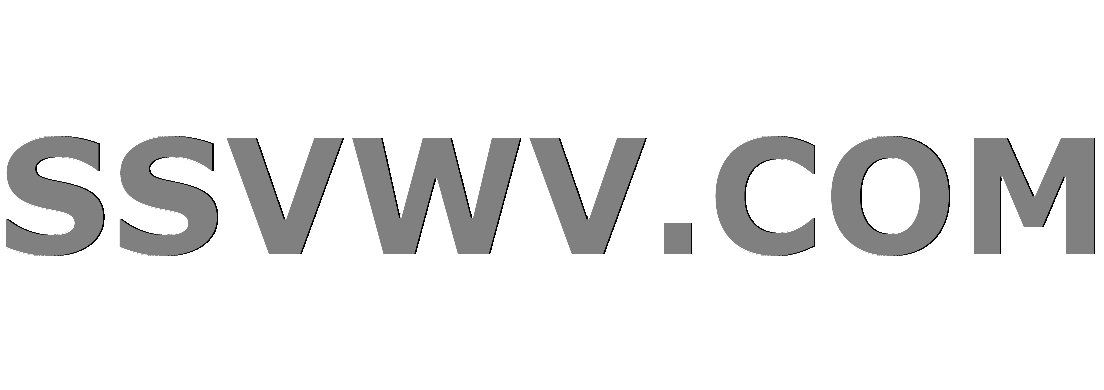
Multi tool use
$begingroup$
This question already has an answer here:
Trying to derive a result on conditional probability
1 answer
Let $X_1,...$ be indepedent rv with common distribution $F(x)$ and $N$ geometric random variable with parameter $p$. $N$ is indepedent from all the $X_i$ . let $M = max(X_1,X_2,...,X_N)$. Find $P(M leq x mid N > 1 ) $
solution sketch
We have
$$ P(M leq x mid N > 1 ) = frac{P( X_1 leq x, X_2 leq x, ..., X_N leq x, N > 1 ) }{P(N > 1) }$$
$$ = frac{P(X_1 leq x )P( X_2 leq x, X_3 leq x, ..., X_N leq x, N > 1 ) }{P(N > 1) } $$
$$ = P(X_1 leq x ) P( max( X_2,X_3,...,X_N leq x mid N > 1 ) $$
$$ = P(X_1 leq x ) P( max( X_1,X_2,...,X_{N-1} leq x mid N > 1 ) $$
$$ = F(x) P( M leq x ) $$
My question is: Why can we pull out $P(X_1 leq x)$?? I mean by this reasoning, we can also do the following
$$ P(M leq x mid N > 1 ) = frac{ P(X_1 leq x) P(X_2 leq x) ... P(X_N leq x ) P(N > 1) }{P(N>1) } = F^n(x) $$
Also, in the equality before last one, why is it that
$$ P( max(X_2,...,X_N) leq x mid N > 1) = P( max(X_1,...,X_{N-1} ) leq x mid N > 1 )$$ Why can we do this ?
probability
$endgroup$
marked as duplicate by Did
StackExchange.ready(function() {
if (StackExchange.options.isMobile) return;
$('.dupe-hammer-message-hover:not(.hover-bound)').each(function() {
var $hover = $(this).addClass('hover-bound'),
$msg = $hover.siblings('.dupe-hammer-message');
$hover.hover(
function() {
$hover.showInfoMessage('', {
messageElement: $msg.clone().show(),
transient: false,
position: { my: 'bottom left', at: 'top center', offsetTop: -7 },
dismissable: false,
relativeToBody: true
});
},
function() {
StackExchange.helpers.removeMessages();
}
);
});
});
Dec 4 '18 at 22:42
This question has been asked before and already has an answer. If those answers do not fully address your question, please ask a new question.
add a comment |
$begingroup$
This question already has an answer here:
Trying to derive a result on conditional probability
1 answer
Let $X_1,...$ be indepedent rv with common distribution $F(x)$ and $N$ geometric random variable with parameter $p$. $N$ is indepedent from all the $X_i$ . let $M = max(X_1,X_2,...,X_N)$. Find $P(M leq x mid N > 1 ) $
solution sketch
We have
$$ P(M leq x mid N > 1 ) = frac{P( X_1 leq x, X_2 leq x, ..., X_N leq x, N > 1 ) }{P(N > 1) }$$
$$ = frac{P(X_1 leq x )P( X_2 leq x, X_3 leq x, ..., X_N leq x, N > 1 ) }{P(N > 1) } $$
$$ = P(X_1 leq x ) P( max( X_2,X_3,...,X_N leq x mid N > 1 ) $$
$$ = P(X_1 leq x ) P( max( X_1,X_2,...,X_{N-1} leq x mid N > 1 ) $$
$$ = F(x) P( M leq x ) $$
My question is: Why can we pull out $P(X_1 leq x)$?? I mean by this reasoning, we can also do the following
$$ P(M leq x mid N > 1 ) = frac{ P(X_1 leq x) P(X_2 leq x) ... P(X_N leq x ) P(N > 1) }{P(N>1) } = F^n(x) $$
Also, in the equality before last one, why is it that
$$ P( max(X_2,...,X_N) leq x mid N > 1) = P( max(X_1,...,X_{N-1} ) leq x mid N > 1 )$$ Why can we do this ?
probability
$endgroup$
marked as duplicate by Did
StackExchange.ready(function() {
if (StackExchange.options.isMobile) return;
$('.dupe-hammer-message-hover:not(.hover-bound)').each(function() {
var $hover = $(this).addClass('hover-bound'),
$msg = $hover.siblings('.dupe-hammer-message');
$hover.hover(
function() {
$hover.showInfoMessage('', {
messageElement: $msg.clone().show(),
transient: false,
position: { my: 'bottom left', at: 'top center', offsetTop: -7 },
dismissable: false,
relativeToBody: true
});
},
function() {
StackExchange.helpers.removeMessages();
}
);
});
});
Dec 4 '18 at 22:42
This question has been asked before and already has an answer. If those answers do not fully address your question, please ask a new question.
$begingroup$
In your proof, how do you get that $P(max(X_1, X_2,...,X_{N-1} leq x | N>1) = P(M leq x)$? $P(M leq x)$ is for the variables $X_1,...,X_n$ all being $leq x$, whereas you only have from $X_1,...,X_{N-1}$ here.
$endgroup$
– bob
Dec 4 '18 at 15:34
$begingroup$
This is the part tat I dont understand, why is that? someone gave me this answer but I dont fully see why
$endgroup$
– Neymar
Dec 4 '18 at 15:36
add a comment |
$begingroup$
This question already has an answer here:
Trying to derive a result on conditional probability
1 answer
Let $X_1,...$ be indepedent rv with common distribution $F(x)$ and $N$ geometric random variable with parameter $p$. $N$ is indepedent from all the $X_i$ . let $M = max(X_1,X_2,...,X_N)$. Find $P(M leq x mid N > 1 ) $
solution sketch
We have
$$ P(M leq x mid N > 1 ) = frac{P( X_1 leq x, X_2 leq x, ..., X_N leq x, N > 1 ) }{P(N > 1) }$$
$$ = frac{P(X_1 leq x )P( X_2 leq x, X_3 leq x, ..., X_N leq x, N > 1 ) }{P(N > 1) } $$
$$ = P(X_1 leq x ) P( max( X_2,X_3,...,X_N leq x mid N > 1 ) $$
$$ = P(X_1 leq x ) P( max( X_1,X_2,...,X_{N-1} leq x mid N > 1 ) $$
$$ = F(x) P( M leq x ) $$
My question is: Why can we pull out $P(X_1 leq x)$?? I mean by this reasoning, we can also do the following
$$ P(M leq x mid N > 1 ) = frac{ P(X_1 leq x) P(X_2 leq x) ... P(X_N leq x ) P(N > 1) }{P(N>1) } = F^n(x) $$
Also, in the equality before last one, why is it that
$$ P( max(X_2,...,X_N) leq x mid N > 1) = P( max(X_1,...,X_{N-1} ) leq x mid N > 1 )$$ Why can we do this ?
probability
$endgroup$
This question already has an answer here:
Trying to derive a result on conditional probability
1 answer
Let $X_1,...$ be indepedent rv with common distribution $F(x)$ and $N$ geometric random variable with parameter $p$. $N$ is indepedent from all the $X_i$ . let $M = max(X_1,X_2,...,X_N)$. Find $P(M leq x mid N > 1 ) $
solution sketch
We have
$$ P(M leq x mid N > 1 ) = frac{P( X_1 leq x, X_2 leq x, ..., X_N leq x, N > 1 ) }{P(N > 1) }$$
$$ = frac{P(X_1 leq x )P( X_2 leq x, X_3 leq x, ..., X_N leq x, N > 1 ) }{P(N > 1) } $$
$$ = P(X_1 leq x ) P( max( X_2,X_3,...,X_N leq x mid N > 1 ) $$
$$ = P(X_1 leq x ) P( max( X_1,X_2,...,X_{N-1} leq x mid N > 1 ) $$
$$ = F(x) P( M leq x ) $$
My question is: Why can we pull out $P(X_1 leq x)$?? I mean by this reasoning, we can also do the following
$$ P(M leq x mid N > 1 ) = frac{ P(X_1 leq x) P(X_2 leq x) ... P(X_N leq x ) P(N > 1) }{P(N>1) } = F^n(x) $$
Also, in the equality before last one, why is it that
$$ P( max(X_2,...,X_N) leq x mid N > 1) = P( max(X_1,...,X_{N-1} ) leq x mid N > 1 )$$ Why can we do this ?
This question already has an answer here:
Trying to derive a result on conditional probability
1 answer
probability
probability
asked Dec 4 '18 at 15:25
NeymarNeymar
355114
355114
marked as duplicate by Did
StackExchange.ready(function() {
if (StackExchange.options.isMobile) return;
$('.dupe-hammer-message-hover:not(.hover-bound)').each(function() {
var $hover = $(this).addClass('hover-bound'),
$msg = $hover.siblings('.dupe-hammer-message');
$hover.hover(
function() {
$hover.showInfoMessage('', {
messageElement: $msg.clone().show(),
transient: false,
position: { my: 'bottom left', at: 'top center', offsetTop: -7 },
dismissable: false,
relativeToBody: true
});
},
function() {
StackExchange.helpers.removeMessages();
}
);
});
});
Dec 4 '18 at 22:42
This question has been asked before and already has an answer. If those answers do not fully address your question, please ask a new question.
marked as duplicate by Did
StackExchange.ready(function() {
if (StackExchange.options.isMobile) return;
$('.dupe-hammer-message-hover:not(.hover-bound)').each(function() {
var $hover = $(this).addClass('hover-bound'),
$msg = $hover.siblings('.dupe-hammer-message');
$hover.hover(
function() {
$hover.showInfoMessage('', {
messageElement: $msg.clone().show(),
transient: false,
position: { my: 'bottom left', at: 'top center', offsetTop: -7 },
dismissable: false,
relativeToBody: true
});
},
function() {
StackExchange.helpers.removeMessages();
}
);
});
});
Dec 4 '18 at 22:42
This question has been asked before and already has an answer. If those answers do not fully address your question, please ask a new question.
$begingroup$
In your proof, how do you get that $P(max(X_1, X_2,...,X_{N-1} leq x | N>1) = P(M leq x)$? $P(M leq x)$ is for the variables $X_1,...,X_n$ all being $leq x$, whereas you only have from $X_1,...,X_{N-1}$ here.
$endgroup$
– bob
Dec 4 '18 at 15:34
$begingroup$
This is the part tat I dont understand, why is that? someone gave me this answer but I dont fully see why
$endgroup$
– Neymar
Dec 4 '18 at 15:36
add a comment |
$begingroup$
In your proof, how do you get that $P(max(X_1, X_2,...,X_{N-1} leq x | N>1) = P(M leq x)$? $P(M leq x)$ is for the variables $X_1,...,X_n$ all being $leq x$, whereas you only have from $X_1,...,X_{N-1}$ here.
$endgroup$
– bob
Dec 4 '18 at 15:34
$begingroup$
This is the part tat I dont understand, why is that? someone gave me this answer but I dont fully see why
$endgroup$
– Neymar
Dec 4 '18 at 15:36
$begingroup$
In your proof, how do you get that $P(max(X_1, X_2,...,X_{N-1} leq x | N>1) = P(M leq x)$? $P(M leq x)$ is for the variables $X_1,...,X_n$ all being $leq x$, whereas you only have from $X_1,...,X_{N-1}$ here.
$endgroup$
– bob
Dec 4 '18 at 15:34
$begingroup$
In your proof, how do you get that $P(max(X_1, X_2,...,X_{N-1} leq x | N>1) = P(M leq x)$? $P(M leq x)$ is for the variables $X_1,...,X_n$ all being $leq x$, whereas you only have from $X_1,...,X_{N-1}$ here.
$endgroup$
– bob
Dec 4 '18 at 15:34
$begingroup$
This is the part tat I dont understand, why is that? someone gave me this answer but I dont fully see why
$endgroup$
– Neymar
Dec 4 '18 at 15:36
$begingroup$
This is the part tat I dont understand, why is that? someone gave me this answer but I dont fully see why
$endgroup$
– Neymar
Dec 4 '18 at 15:36
add a comment |
1 Answer
1
active
oldest
votes
$begingroup$
This can be done directly using the law of total probability. Note that
$$ begin{align} P(Mleq x) & = P(Mleq x|N = 1)P(N=1) + P(Mleq x | N > 1)P(N>1) \ & = F(x)p + (1-p)P(Mleq x| N > 1)end{align}$$
We can calculate $P(Mleq x)$ as follows using a geometric sum:
$$ begin{align} P(Mleq x) & = sum_{ngeq 1} P(Mleq x|N=n)P(N=n)\ &= sum_{ngeq 1} F(x)^n (1-p)^{n-1} p \ & = frac{F(x)p}{1 - F(x)(1-p)} end{align} $$
where $P(Mleq x | N=n) = F(x)^n$ since the $X_i$ are independent. Some quick rearranging gives $$ P(Mleq x|N>1) = frac{F(x)^2p}{1-F(x)(1-p)} = F(x)P(Mleq x)$$
For your method : we can pull out the $P(X_1 leq x)$ since $X_1$ is independent from $X_i$ for $i > 1$ and from $N$. We can't pull out all of the $X_i$ because how many there are depends on $N$. In the equality before last, we are just relabelling $X_i$ to $X_{i-1}$ which we are allowed to do as the $X_i$ are i.i.d. However, I'm not a hundred percent sure how to justify the last equality.
$endgroup$
add a comment |
1 Answer
1
active
oldest
votes
1 Answer
1
active
oldest
votes
active
oldest
votes
active
oldest
votes
$begingroup$
This can be done directly using the law of total probability. Note that
$$ begin{align} P(Mleq x) & = P(Mleq x|N = 1)P(N=1) + P(Mleq x | N > 1)P(N>1) \ & = F(x)p + (1-p)P(Mleq x| N > 1)end{align}$$
We can calculate $P(Mleq x)$ as follows using a geometric sum:
$$ begin{align} P(Mleq x) & = sum_{ngeq 1} P(Mleq x|N=n)P(N=n)\ &= sum_{ngeq 1} F(x)^n (1-p)^{n-1} p \ & = frac{F(x)p}{1 - F(x)(1-p)} end{align} $$
where $P(Mleq x | N=n) = F(x)^n$ since the $X_i$ are independent. Some quick rearranging gives $$ P(Mleq x|N>1) = frac{F(x)^2p}{1-F(x)(1-p)} = F(x)P(Mleq x)$$
For your method : we can pull out the $P(X_1 leq x)$ since $X_1$ is independent from $X_i$ for $i > 1$ and from $N$. We can't pull out all of the $X_i$ because how many there are depends on $N$. In the equality before last, we are just relabelling $X_i$ to $X_{i-1}$ which we are allowed to do as the $X_i$ are i.i.d. However, I'm not a hundred percent sure how to justify the last equality.
$endgroup$
add a comment |
$begingroup$
This can be done directly using the law of total probability. Note that
$$ begin{align} P(Mleq x) & = P(Mleq x|N = 1)P(N=1) + P(Mleq x | N > 1)P(N>1) \ & = F(x)p + (1-p)P(Mleq x| N > 1)end{align}$$
We can calculate $P(Mleq x)$ as follows using a geometric sum:
$$ begin{align} P(Mleq x) & = sum_{ngeq 1} P(Mleq x|N=n)P(N=n)\ &= sum_{ngeq 1} F(x)^n (1-p)^{n-1} p \ & = frac{F(x)p}{1 - F(x)(1-p)} end{align} $$
where $P(Mleq x | N=n) = F(x)^n$ since the $X_i$ are independent. Some quick rearranging gives $$ P(Mleq x|N>1) = frac{F(x)^2p}{1-F(x)(1-p)} = F(x)P(Mleq x)$$
For your method : we can pull out the $P(X_1 leq x)$ since $X_1$ is independent from $X_i$ for $i > 1$ and from $N$. We can't pull out all of the $X_i$ because how many there are depends on $N$. In the equality before last, we are just relabelling $X_i$ to $X_{i-1}$ which we are allowed to do as the $X_i$ are i.i.d. However, I'm not a hundred percent sure how to justify the last equality.
$endgroup$
add a comment |
$begingroup$
This can be done directly using the law of total probability. Note that
$$ begin{align} P(Mleq x) & = P(Mleq x|N = 1)P(N=1) + P(Mleq x | N > 1)P(N>1) \ & = F(x)p + (1-p)P(Mleq x| N > 1)end{align}$$
We can calculate $P(Mleq x)$ as follows using a geometric sum:
$$ begin{align} P(Mleq x) & = sum_{ngeq 1} P(Mleq x|N=n)P(N=n)\ &= sum_{ngeq 1} F(x)^n (1-p)^{n-1} p \ & = frac{F(x)p}{1 - F(x)(1-p)} end{align} $$
where $P(Mleq x | N=n) = F(x)^n$ since the $X_i$ are independent. Some quick rearranging gives $$ P(Mleq x|N>1) = frac{F(x)^2p}{1-F(x)(1-p)} = F(x)P(Mleq x)$$
For your method : we can pull out the $P(X_1 leq x)$ since $X_1$ is independent from $X_i$ for $i > 1$ and from $N$. We can't pull out all of the $X_i$ because how many there are depends on $N$. In the equality before last, we are just relabelling $X_i$ to $X_{i-1}$ which we are allowed to do as the $X_i$ are i.i.d. However, I'm not a hundred percent sure how to justify the last equality.
$endgroup$
This can be done directly using the law of total probability. Note that
$$ begin{align} P(Mleq x) & = P(Mleq x|N = 1)P(N=1) + P(Mleq x | N > 1)P(N>1) \ & = F(x)p + (1-p)P(Mleq x| N > 1)end{align}$$
We can calculate $P(Mleq x)$ as follows using a geometric sum:
$$ begin{align} P(Mleq x) & = sum_{ngeq 1} P(Mleq x|N=n)P(N=n)\ &= sum_{ngeq 1} F(x)^n (1-p)^{n-1} p \ & = frac{F(x)p}{1 - F(x)(1-p)} end{align} $$
where $P(Mleq x | N=n) = F(x)^n$ since the $X_i$ are independent. Some quick rearranging gives $$ P(Mleq x|N>1) = frac{F(x)^2p}{1-F(x)(1-p)} = F(x)P(Mleq x)$$
For your method : we can pull out the $P(X_1 leq x)$ since $X_1$ is independent from $X_i$ for $i > 1$ and from $N$. We can't pull out all of the $X_i$ because how many there are depends on $N$. In the equality before last, we are just relabelling $X_i$ to $X_{i-1}$ which we are allowed to do as the $X_i$ are i.i.d. However, I'm not a hundred percent sure how to justify the last equality.
answered Dec 4 '18 at 16:31
ODFODF
1,431510
1,431510
add a comment |
add a comment |
E8m LA,5mxXh K30q7 AHDGdDHoIAuJmhZ3L mTB2SaNVxf57,Z5PlvF1U8k3 Gpe9PT5eeh
$begingroup$
In your proof, how do you get that $P(max(X_1, X_2,...,X_{N-1} leq x | N>1) = P(M leq x)$? $P(M leq x)$ is for the variables $X_1,...,X_n$ all being $leq x$, whereas you only have from $X_1,...,X_{N-1}$ here.
$endgroup$
– bob
Dec 4 '18 at 15:34
$begingroup$
This is the part tat I dont understand, why is that? someone gave me this answer but I dont fully see why
$endgroup$
– Neymar
Dec 4 '18 at 15:36