Differentiating a simple, single-variable equation involving a vector
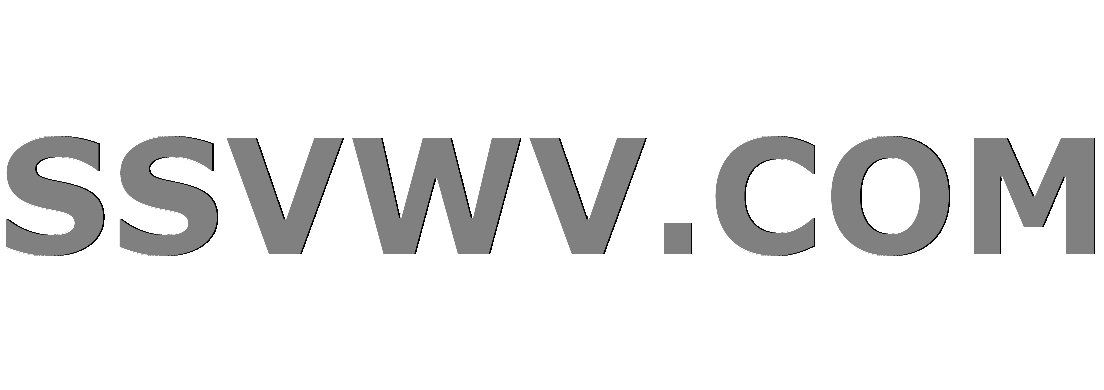
Multi tool use
up vote
2
down vote
favorite
Please forgive how simple this is, but I can't seem to find any explanations for how to differentiate single-variable equations of the following form:
$f(boldsymbol{x}) = 5boldsymbol{x}$, where $boldsymbol{x}$ is a $n$-dimensional column-vector of scalar values; i.e., $boldsymbol{x} = langle a_1, a_2, a_3, ... a_n rangle$ for $a_i in mathbb{R}$).
Also, if vector-valued functions are those that map a vector to a scalar (i.e. $mathbb{R}^n to mathbb{R}$), what are functions like this called?
calculus vectors vector-analysis
add a comment |
up vote
2
down vote
favorite
Please forgive how simple this is, but I can't seem to find any explanations for how to differentiate single-variable equations of the following form:
$f(boldsymbol{x}) = 5boldsymbol{x}$, where $boldsymbol{x}$ is a $n$-dimensional column-vector of scalar values; i.e., $boldsymbol{x} = langle a_1, a_2, a_3, ... a_n rangle$ for $a_i in mathbb{R}$).
Also, if vector-valued functions are those that map a vector to a scalar (i.e. $mathbb{R}^n to mathbb{R}$), what are functions like this called?
calculus vectors vector-analysis
With respect to what do you want to derive it? Usually if we "derive" vectors, we mean taking the divergence
– Wesley Strik
Nov 23 at 21:07
Vector-valued functions can also output a vector, as in your example. An example of a vector valued function that maps to the reals, take: $f(vec{v})=v_1 ^2 -v_2$
– Wesley Strik
Nov 23 at 21:18
Vector-valued functions would not have an output of a scalar. This is the point of the term "vector-valued". Sure, to be technical, real numbers are also one-dimensional vectors, but this observation is at odds with the intent of the terminology "vector-valued". A function $f: mathbb{R}^n rightarrow mathbb{R}^m$ should be called an $m$-vector valued function of $n$-variables, or something similar.
– James S. Cook
Nov 24 at 1:12
add a comment |
up vote
2
down vote
favorite
up vote
2
down vote
favorite
Please forgive how simple this is, but I can't seem to find any explanations for how to differentiate single-variable equations of the following form:
$f(boldsymbol{x}) = 5boldsymbol{x}$, where $boldsymbol{x}$ is a $n$-dimensional column-vector of scalar values; i.e., $boldsymbol{x} = langle a_1, a_2, a_3, ... a_n rangle$ for $a_i in mathbb{R}$).
Also, if vector-valued functions are those that map a vector to a scalar (i.e. $mathbb{R}^n to mathbb{R}$), what are functions like this called?
calculus vectors vector-analysis
Please forgive how simple this is, but I can't seem to find any explanations for how to differentiate single-variable equations of the following form:
$f(boldsymbol{x}) = 5boldsymbol{x}$, where $boldsymbol{x}$ is a $n$-dimensional column-vector of scalar values; i.e., $boldsymbol{x} = langle a_1, a_2, a_3, ... a_n rangle$ for $a_i in mathbb{R}$).
Also, if vector-valued functions are those that map a vector to a scalar (i.e. $mathbb{R}^n to mathbb{R}$), what are functions like this called?
calculus vectors vector-analysis
calculus vectors vector-analysis
edited Nov 23 at 21:58


Wesley Strik
1,510422
1,510422
asked Nov 23 at 20:59
lthompson
1099
1099
With respect to what do you want to derive it? Usually if we "derive" vectors, we mean taking the divergence
– Wesley Strik
Nov 23 at 21:07
Vector-valued functions can also output a vector, as in your example. An example of a vector valued function that maps to the reals, take: $f(vec{v})=v_1 ^2 -v_2$
– Wesley Strik
Nov 23 at 21:18
Vector-valued functions would not have an output of a scalar. This is the point of the term "vector-valued". Sure, to be technical, real numbers are also one-dimensional vectors, but this observation is at odds with the intent of the terminology "vector-valued". A function $f: mathbb{R}^n rightarrow mathbb{R}^m$ should be called an $m$-vector valued function of $n$-variables, or something similar.
– James S. Cook
Nov 24 at 1:12
add a comment |
With respect to what do you want to derive it? Usually if we "derive" vectors, we mean taking the divergence
– Wesley Strik
Nov 23 at 21:07
Vector-valued functions can also output a vector, as in your example. An example of a vector valued function that maps to the reals, take: $f(vec{v})=v_1 ^2 -v_2$
– Wesley Strik
Nov 23 at 21:18
Vector-valued functions would not have an output of a scalar. This is the point of the term "vector-valued". Sure, to be technical, real numbers are also one-dimensional vectors, but this observation is at odds with the intent of the terminology "vector-valued". A function $f: mathbb{R}^n rightarrow mathbb{R}^m$ should be called an $m$-vector valued function of $n$-variables, or something similar.
– James S. Cook
Nov 24 at 1:12
With respect to what do you want to derive it? Usually if we "derive" vectors, we mean taking the divergence
– Wesley Strik
Nov 23 at 21:07
With respect to what do you want to derive it? Usually if we "derive" vectors, we mean taking the divergence
– Wesley Strik
Nov 23 at 21:07
Vector-valued functions can also output a vector, as in your example. An example of a vector valued function that maps to the reals, take: $f(vec{v})=v_1 ^2 -v_2$
– Wesley Strik
Nov 23 at 21:18
Vector-valued functions can also output a vector, as in your example. An example of a vector valued function that maps to the reals, take: $f(vec{v})=v_1 ^2 -v_2$
– Wesley Strik
Nov 23 at 21:18
Vector-valued functions would not have an output of a scalar. This is the point of the term "vector-valued". Sure, to be technical, real numbers are also one-dimensional vectors, but this observation is at odds with the intent of the terminology "vector-valued". A function $f: mathbb{R}^n rightarrow mathbb{R}^m$ should be called an $m$-vector valued function of $n$-variables, or something similar.
– James S. Cook
Nov 24 at 1:12
Vector-valued functions would not have an output of a scalar. This is the point of the term "vector-valued". Sure, to be technical, real numbers are also one-dimensional vectors, but this observation is at odds with the intent of the terminology "vector-valued". A function $f: mathbb{R}^n rightarrow mathbb{R}^m$ should be called an $m$-vector valued function of $n$-variables, or something similar.
– James S. Cook
Nov 24 at 1:12
add a comment |
2 Answers
2
active
oldest
votes
up vote
1
down vote
The differential of a mapping $f: mathbb{R}^n rightarrow mathbb{R}^n$ at a point $p$, if it exists, is a linear transformation $df_p: mathbb{R}^n rightarrow mathbb{R}^n$ which best approximates the change in $f$ near $p$. In particular, the differential $df_p$ is implicitly defined by the Frechet quotient:
$$ lim_{h rightarrow 0} frac{f(p+h)-f(p)-df_p(h)}{| h |} = 0$$
For small $h$, $f(p+h) simeq f(p) + df_p(h)$. The relation of the differential and the partial derivatives more commonly taught in introductory calculus is given by the definition $frac{partial f}{partial x_i}(p) = df_p(e_i)$ where $(e_i)_j = delta_{ij}$ or equivalently $e_i cdot e_j = delta_{ij}$. Here I use $e_1,e_2,dots , e_n$ to denote the standard basis for $mathbb{R}^n$. Incidentally, this definition of partial derivatives equally well applies to a basis for some abstract finite dimensional normed linear space. That said, $| h | = sqrt{ h cdot h}$ is the length of $h$. Notice, we cannot divide by $h$ since generally division by a vector is not defined. Getting back to the main story,
$$ J_f(p) = [df_p] = [df_p(e_1)|df_p(e_2)| cdots | df_p(e_n)] = left[ frac{partial f}{partial x_1}(p)bigg{|}frac{partial f}{partial x_2}(p)bigg{|}cdots bigg{|}frac{partial f}{partial x_n}(p) right] $$
is the Jacobian matrix of $f$ at $p$. The relation between $df_p$ and $J_f(p)$ is given by matrix multiplication:
$$ df_p(h) = J_f(p)h $$
We can view the Jacobian as a stack of gradient vectors, one for each component function of $f = (f_1,f_2, dots , f_n)$; $nabla f_j = [partial_1 f_j, dots , partial_n f_j]^T$ and
$$ J_f = left[ begin{array}{c} (nabla f_1)^T \ (nabla f_2)^T \ vdots \ (nabla f_n)^T end{array}right] $$
Thus,
$$ df_p(h) = left[ begin{array}{c} (nabla f_1)^T \ (nabla f_1)^T \ vdots \ (nabla f_n)^T end{array}right]left[ begin{array}{c} h_1 \ h_2 \ vdots \ h_n end{array}right] = left[ begin{array}{c} (nabla f_1) cdot h \ (nabla f_2)cdot h \ vdots \ (nabla f_n) cdot hend{array}right]. $$
In fact, the derivative (differential) of $f$ involves many gradients at once working in concert as above. You see, the larger confusion here is the tendency for students to assume the derivative of a function on $mathbb{R}^n$ should be another function on $mathbb{R}^n$. It's not. The first derivative is naturally identified with the pointwise assignment of a linear map at each such point as the Frechet quotient exists. Then, it turns out the higher derivatives of a function on $mathbb{R}^n$ can be identified with the pointwise assignment of a completely symmetric $k$-linear mapping. These things are explained rather nicely in Volume 2 of Zorich's Mathematical Analysis. However, this material is standard in any higher course in multivariate analysis.
Getting back to your actual function $f(x) = 5x$, this function is linear so the best linear approximation to the function is essentially the function itself. We can calculate $J_f(p) = 5I_n$ where $I_n$ is the $n times n$ identity matrix. Or, if you prefer, $df_p(h) = 5I_nh = 5h$ for each $p in mathbb{R}^n$.
add a comment |
up vote
-1
down vote
Actually, a multivariate derivation is called gradient. For a function $f(x_1,x_2,cdots ,x_n):Bbb R^nto Bbb R$ we define a gradient vector as following:
A gradient vector contains $n$ components namely $g_i$ , $i=1,2,cdots ,n$. We therefore define $$g_i={partial f(x_1,cdots , x_i,cdots , x_n)over partial x_i}$$as if other $x_j$s are constant. Then the gradient vector would be$$nabla f=[g_1 g_2 cdots g_n]$$for a function $f:Bbb R^nto Bbb R^n$ we define a gradient matrix instead whose entries (namely $g_{ij}$) are:$$g_{ij}={partial f_i(x_1,cdots , x_n)over partial x_j}$$where$$f=[f_1 f_2 cdots f_n]$$In this question, the gradient matrix will become$$nabla f=5I_n$$where $I_n$ is the identity matrix of order $n$ (why?).
P.S. for higher dimension input and/or output functions, the gradient should be defined using tensors.
Note that his $x$ is a vector, not a multivariable function. Therefore he should actually take the divergence. Now if he had written something like f(x,y,z)=x+3y+5z. It would actually correspond to what he is saying about mapping to the reals.
– Wesley Strik
Nov 23 at 21:15
That's right and i included it in my answer. Then what's wrong?
– Mostafa Ayaz
Nov 23 at 21:17
1
The gradient matrix can still be defined for functions $Bbb R^nto Bbb R^n$
– Mostafa Ayaz
Nov 23 at 21:18
1
Still thank you for minding that tip in my answer. Higher dimension gradients are always trickier....
– Mostafa Ayaz
Nov 23 at 21:24
1
That's why I tried to clarify the definitions purely without junks :)
– Mostafa Ayaz
Nov 23 at 21:27
|
show 2 more comments
Your Answer
StackExchange.ifUsing("editor", function () {
return StackExchange.using("mathjaxEditing", function () {
StackExchange.MarkdownEditor.creationCallbacks.add(function (editor, postfix) {
StackExchange.mathjaxEditing.prepareWmdForMathJax(editor, postfix, [["$", "$"], ["\\(","\\)"]]);
});
});
}, "mathjax-editing");
StackExchange.ready(function() {
var channelOptions = {
tags: "".split(" "),
id: "69"
};
initTagRenderer("".split(" "), "".split(" "), channelOptions);
StackExchange.using("externalEditor", function() {
// Have to fire editor after snippets, if snippets enabled
if (StackExchange.settings.snippets.snippetsEnabled) {
StackExchange.using("snippets", function() {
createEditor();
});
}
else {
createEditor();
}
});
function createEditor() {
StackExchange.prepareEditor({
heartbeatType: 'answer',
autoActivateHeartbeat: false,
convertImagesToLinks: true,
noModals: true,
showLowRepImageUploadWarning: true,
reputationToPostImages: 10,
bindNavPrevention: true,
postfix: "",
imageUploader: {
brandingHtml: "Powered by u003ca class="icon-imgur-white" href="https://imgur.com/"u003eu003c/au003e",
contentPolicyHtml: "User contributions licensed under u003ca href="https://creativecommons.org/licenses/by-sa/3.0/"u003ecc by-sa 3.0 with attribution requiredu003c/au003e u003ca href="https://stackoverflow.com/legal/content-policy"u003e(content policy)u003c/au003e",
allowUrls: true
},
noCode: true, onDemand: true,
discardSelector: ".discard-answer"
,immediatelyShowMarkdownHelp:true
});
}
});
Sign up or log in
StackExchange.ready(function () {
StackExchange.helpers.onClickDraftSave('#login-link');
});
Sign up using Google
Sign up using Facebook
Sign up using Email and Password
Post as a guest
Required, but never shown
StackExchange.ready(
function () {
StackExchange.openid.initPostLogin('.new-post-login', 'https%3a%2f%2fmath.stackexchange.com%2fquestions%2f3010828%2fdifferentiating-a-simple-single-variable-equation-involving-a-vector%23new-answer', 'question_page');
}
);
Post as a guest
Required, but never shown
2 Answers
2
active
oldest
votes
2 Answers
2
active
oldest
votes
active
oldest
votes
active
oldest
votes
up vote
1
down vote
The differential of a mapping $f: mathbb{R}^n rightarrow mathbb{R}^n$ at a point $p$, if it exists, is a linear transformation $df_p: mathbb{R}^n rightarrow mathbb{R}^n$ which best approximates the change in $f$ near $p$. In particular, the differential $df_p$ is implicitly defined by the Frechet quotient:
$$ lim_{h rightarrow 0} frac{f(p+h)-f(p)-df_p(h)}{| h |} = 0$$
For small $h$, $f(p+h) simeq f(p) + df_p(h)$. The relation of the differential and the partial derivatives more commonly taught in introductory calculus is given by the definition $frac{partial f}{partial x_i}(p) = df_p(e_i)$ where $(e_i)_j = delta_{ij}$ or equivalently $e_i cdot e_j = delta_{ij}$. Here I use $e_1,e_2,dots , e_n$ to denote the standard basis for $mathbb{R}^n$. Incidentally, this definition of partial derivatives equally well applies to a basis for some abstract finite dimensional normed linear space. That said, $| h | = sqrt{ h cdot h}$ is the length of $h$. Notice, we cannot divide by $h$ since generally division by a vector is not defined. Getting back to the main story,
$$ J_f(p) = [df_p] = [df_p(e_1)|df_p(e_2)| cdots | df_p(e_n)] = left[ frac{partial f}{partial x_1}(p)bigg{|}frac{partial f}{partial x_2}(p)bigg{|}cdots bigg{|}frac{partial f}{partial x_n}(p) right] $$
is the Jacobian matrix of $f$ at $p$. The relation between $df_p$ and $J_f(p)$ is given by matrix multiplication:
$$ df_p(h) = J_f(p)h $$
We can view the Jacobian as a stack of gradient vectors, one for each component function of $f = (f_1,f_2, dots , f_n)$; $nabla f_j = [partial_1 f_j, dots , partial_n f_j]^T$ and
$$ J_f = left[ begin{array}{c} (nabla f_1)^T \ (nabla f_2)^T \ vdots \ (nabla f_n)^T end{array}right] $$
Thus,
$$ df_p(h) = left[ begin{array}{c} (nabla f_1)^T \ (nabla f_1)^T \ vdots \ (nabla f_n)^T end{array}right]left[ begin{array}{c} h_1 \ h_2 \ vdots \ h_n end{array}right] = left[ begin{array}{c} (nabla f_1) cdot h \ (nabla f_2)cdot h \ vdots \ (nabla f_n) cdot hend{array}right]. $$
In fact, the derivative (differential) of $f$ involves many gradients at once working in concert as above. You see, the larger confusion here is the tendency for students to assume the derivative of a function on $mathbb{R}^n$ should be another function on $mathbb{R}^n$. It's not. The first derivative is naturally identified with the pointwise assignment of a linear map at each such point as the Frechet quotient exists. Then, it turns out the higher derivatives of a function on $mathbb{R}^n$ can be identified with the pointwise assignment of a completely symmetric $k$-linear mapping. These things are explained rather nicely in Volume 2 of Zorich's Mathematical Analysis. However, this material is standard in any higher course in multivariate analysis.
Getting back to your actual function $f(x) = 5x$, this function is linear so the best linear approximation to the function is essentially the function itself. We can calculate $J_f(p) = 5I_n$ where $I_n$ is the $n times n$ identity matrix. Or, if you prefer, $df_p(h) = 5I_nh = 5h$ for each $p in mathbb{R}^n$.
add a comment |
up vote
1
down vote
The differential of a mapping $f: mathbb{R}^n rightarrow mathbb{R}^n$ at a point $p$, if it exists, is a linear transformation $df_p: mathbb{R}^n rightarrow mathbb{R}^n$ which best approximates the change in $f$ near $p$. In particular, the differential $df_p$ is implicitly defined by the Frechet quotient:
$$ lim_{h rightarrow 0} frac{f(p+h)-f(p)-df_p(h)}{| h |} = 0$$
For small $h$, $f(p+h) simeq f(p) + df_p(h)$. The relation of the differential and the partial derivatives more commonly taught in introductory calculus is given by the definition $frac{partial f}{partial x_i}(p) = df_p(e_i)$ where $(e_i)_j = delta_{ij}$ or equivalently $e_i cdot e_j = delta_{ij}$. Here I use $e_1,e_2,dots , e_n$ to denote the standard basis for $mathbb{R}^n$. Incidentally, this definition of partial derivatives equally well applies to a basis for some abstract finite dimensional normed linear space. That said, $| h | = sqrt{ h cdot h}$ is the length of $h$. Notice, we cannot divide by $h$ since generally division by a vector is not defined. Getting back to the main story,
$$ J_f(p) = [df_p] = [df_p(e_1)|df_p(e_2)| cdots | df_p(e_n)] = left[ frac{partial f}{partial x_1}(p)bigg{|}frac{partial f}{partial x_2}(p)bigg{|}cdots bigg{|}frac{partial f}{partial x_n}(p) right] $$
is the Jacobian matrix of $f$ at $p$. The relation between $df_p$ and $J_f(p)$ is given by matrix multiplication:
$$ df_p(h) = J_f(p)h $$
We can view the Jacobian as a stack of gradient vectors, one for each component function of $f = (f_1,f_2, dots , f_n)$; $nabla f_j = [partial_1 f_j, dots , partial_n f_j]^T$ and
$$ J_f = left[ begin{array}{c} (nabla f_1)^T \ (nabla f_2)^T \ vdots \ (nabla f_n)^T end{array}right] $$
Thus,
$$ df_p(h) = left[ begin{array}{c} (nabla f_1)^T \ (nabla f_1)^T \ vdots \ (nabla f_n)^T end{array}right]left[ begin{array}{c} h_1 \ h_2 \ vdots \ h_n end{array}right] = left[ begin{array}{c} (nabla f_1) cdot h \ (nabla f_2)cdot h \ vdots \ (nabla f_n) cdot hend{array}right]. $$
In fact, the derivative (differential) of $f$ involves many gradients at once working in concert as above. You see, the larger confusion here is the tendency for students to assume the derivative of a function on $mathbb{R}^n$ should be another function on $mathbb{R}^n$. It's not. The first derivative is naturally identified with the pointwise assignment of a linear map at each such point as the Frechet quotient exists. Then, it turns out the higher derivatives of a function on $mathbb{R}^n$ can be identified with the pointwise assignment of a completely symmetric $k$-linear mapping. These things are explained rather nicely in Volume 2 of Zorich's Mathematical Analysis. However, this material is standard in any higher course in multivariate analysis.
Getting back to your actual function $f(x) = 5x$, this function is linear so the best linear approximation to the function is essentially the function itself. We can calculate $J_f(p) = 5I_n$ where $I_n$ is the $n times n$ identity matrix. Or, if you prefer, $df_p(h) = 5I_nh = 5h$ for each $p in mathbb{R}^n$.
add a comment |
up vote
1
down vote
up vote
1
down vote
The differential of a mapping $f: mathbb{R}^n rightarrow mathbb{R}^n$ at a point $p$, if it exists, is a linear transformation $df_p: mathbb{R}^n rightarrow mathbb{R}^n$ which best approximates the change in $f$ near $p$. In particular, the differential $df_p$ is implicitly defined by the Frechet quotient:
$$ lim_{h rightarrow 0} frac{f(p+h)-f(p)-df_p(h)}{| h |} = 0$$
For small $h$, $f(p+h) simeq f(p) + df_p(h)$. The relation of the differential and the partial derivatives more commonly taught in introductory calculus is given by the definition $frac{partial f}{partial x_i}(p) = df_p(e_i)$ where $(e_i)_j = delta_{ij}$ or equivalently $e_i cdot e_j = delta_{ij}$. Here I use $e_1,e_2,dots , e_n$ to denote the standard basis for $mathbb{R}^n$. Incidentally, this definition of partial derivatives equally well applies to a basis for some abstract finite dimensional normed linear space. That said, $| h | = sqrt{ h cdot h}$ is the length of $h$. Notice, we cannot divide by $h$ since generally division by a vector is not defined. Getting back to the main story,
$$ J_f(p) = [df_p] = [df_p(e_1)|df_p(e_2)| cdots | df_p(e_n)] = left[ frac{partial f}{partial x_1}(p)bigg{|}frac{partial f}{partial x_2}(p)bigg{|}cdots bigg{|}frac{partial f}{partial x_n}(p) right] $$
is the Jacobian matrix of $f$ at $p$. The relation between $df_p$ and $J_f(p)$ is given by matrix multiplication:
$$ df_p(h) = J_f(p)h $$
We can view the Jacobian as a stack of gradient vectors, one for each component function of $f = (f_1,f_2, dots , f_n)$; $nabla f_j = [partial_1 f_j, dots , partial_n f_j]^T$ and
$$ J_f = left[ begin{array}{c} (nabla f_1)^T \ (nabla f_2)^T \ vdots \ (nabla f_n)^T end{array}right] $$
Thus,
$$ df_p(h) = left[ begin{array}{c} (nabla f_1)^T \ (nabla f_1)^T \ vdots \ (nabla f_n)^T end{array}right]left[ begin{array}{c} h_1 \ h_2 \ vdots \ h_n end{array}right] = left[ begin{array}{c} (nabla f_1) cdot h \ (nabla f_2)cdot h \ vdots \ (nabla f_n) cdot hend{array}right]. $$
In fact, the derivative (differential) of $f$ involves many gradients at once working in concert as above. You see, the larger confusion here is the tendency for students to assume the derivative of a function on $mathbb{R}^n$ should be another function on $mathbb{R}^n$. It's not. The first derivative is naturally identified with the pointwise assignment of a linear map at each such point as the Frechet quotient exists. Then, it turns out the higher derivatives of a function on $mathbb{R}^n$ can be identified with the pointwise assignment of a completely symmetric $k$-linear mapping. These things are explained rather nicely in Volume 2 of Zorich's Mathematical Analysis. However, this material is standard in any higher course in multivariate analysis.
Getting back to your actual function $f(x) = 5x$, this function is linear so the best linear approximation to the function is essentially the function itself. We can calculate $J_f(p) = 5I_n$ where $I_n$ is the $n times n$ identity matrix. Or, if you prefer, $df_p(h) = 5I_nh = 5h$ for each $p in mathbb{R}^n$.
The differential of a mapping $f: mathbb{R}^n rightarrow mathbb{R}^n$ at a point $p$, if it exists, is a linear transformation $df_p: mathbb{R}^n rightarrow mathbb{R}^n$ which best approximates the change in $f$ near $p$. In particular, the differential $df_p$ is implicitly defined by the Frechet quotient:
$$ lim_{h rightarrow 0} frac{f(p+h)-f(p)-df_p(h)}{| h |} = 0$$
For small $h$, $f(p+h) simeq f(p) + df_p(h)$. The relation of the differential and the partial derivatives more commonly taught in introductory calculus is given by the definition $frac{partial f}{partial x_i}(p) = df_p(e_i)$ where $(e_i)_j = delta_{ij}$ or equivalently $e_i cdot e_j = delta_{ij}$. Here I use $e_1,e_2,dots , e_n$ to denote the standard basis for $mathbb{R}^n$. Incidentally, this definition of partial derivatives equally well applies to a basis for some abstract finite dimensional normed linear space. That said, $| h | = sqrt{ h cdot h}$ is the length of $h$. Notice, we cannot divide by $h$ since generally division by a vector is not defined. Getting back to the main story,
$$ J_f(p) = [df_p] = [df_p(e_1)|df_p(e_2)| cdots | df_p(e_n)] = left[ frac{partial f}{partial x_1}(p)bigg{|}frac{partial f}{partial x_2}(p)bigg{|}cdots bigg{|}frac{partial f}{partial x_n}(p) right] $$
is the Jacobian matrix of $f$ at $p$. The relation between $df_p$ and $J_f(p)$ is given by matrix multiplication:
$$ df_p(h) = J_f(p)h $$
We can view the Jacobian as a stack of gradient vectors, one for each component function of $f = (f_1,f_2, dots , f_n)$; $nabla f_j = [partial_1 f_j, dots , partial_n f_j]^T$ and
$$ J_f = left[ begin{array}{c} (nabla f_1)^T \ (nabla f_2)^T \ vdots \ (nabla f_n)^T end{array}right] $$
Thus,
$$ df_p(h) = left[ begin{array}{c} (nabla f_1)^T \ (nabla f_1)^T \ vdots \ (nabla f_n)^T end{array}right]left[ begin{array}{c} h_1 \ h_2 \ vdots \ h_n end{array}right] = left[ begin{array}{c} (nabla f_1) cdot h \ (nabla f_2)cdot h \ vdots \ (nabla f_n) cdot hend{array}right]. $$
In fact, the derivative (differential) of $f$ involves many gradients at once working in concert as above. You see, the larger confusion here is the tendency for students to assume the derivative of a function on $mathbb{R}^n$ should be another function on $mathbb{R}^n$. It's not. The first derivative is naturally identified with the pointwise assignment of a linear map at each such point as the Frechet quotient exists. Then, it turns out the higher derivatives of a function on $mathbb{R}^n$ can be identified with the pointwise assignment of a completely symmetric $k$-linear mapping. These things are explained rather nicely in Volume 2 of Zorich's Mathematical Analysis. However, this material is standard in any higher course in multivariate analysis.
Getting back to your actual function $f(x) = 5x$, this function is linear so the best linear approximation to the function is essentially the function itself. We can calculate $J_f(p) = 5I_n$ where $I_n$ is the $n times n$ identity matrix. Or, if you prefer, $df_p(h) = 5I_nh = 5h$ for each $p in mathbb{R}^n$.
answered Nov 24 at 1:08


James S. Cook
13k22870
13k22870
add a comment |
add a comment |
up vote
-1
down vote
Actually, a multivariate derivation is called gradient. For a function $f(x_1,x_2,cdots ,x_n):Bbb R^nto Bbb R$ we define a gradient vector as following:
A gradient vector contains $n$ components namely $g_i$ , $i=1,2,cdots ,n$. We therefore define $$g_i={partial f(x_1,cdots , x_i,cdots , x_n)over partial x_i}$$as if other $x_j$s are constant. Then the gradient vector would be$$nabla f=[g_1 g_2 cdots g_n]$$for a function $f:Bbb R^nto Bbb R^n$ we define a gradient matrix instead whose entries (namely $g_{ij}$) are:$$g_{ij}={partial f_i(x_1,cdots , x_n)over partial x_j}$$where$$f=[f_1 f_2 cdots f_n]$$In this question, the gradient matrix will become$$nabla f=5I_n$$where $I_n$ is the identity matrix of order $n$ (why?).
P.S. for higher dimension input and/or output functions, the gradient should be defined using tensors.
Note that his $x$ is a vector, not a multivariable function. Therefore he should actually take the divergence. Now if he had written something like f(x,y,z)=x+3y+5z. It would actually correspond to what he is saying about mapping to the reals.
– Wesley Strik
Nov 23 at 21:15
That's right and i included it in my answer. Then what's wrong?
– Mostafa Ayaz
Nov 23 at 21:17
1
The gradient matrix can still be defined for functions $Bbb R^nto Bbb R^n$
– Mostafa Ayaz
Nov 23 at 21:18
1
Still thank you for minding that tip in my answer. Higher dimension gradients are always trickier....
– Mostafa Ayaz
Nov 23 at 21:24
1
That's why I tried to clarify the definitions purely without junks :)
– Mostafa Ayaz
Nov 23 at 21:27
|
show 2 more comments
up vote
-1
down vote
Actually, a multivariate derivation is called gradient. For a function $f(x_1,x_2,cdots ,x_n):Bbb R^nto Bbb R$ we define a gradient vector as following:
A gradient vector contains $n$ components namely $g_i$ , $i=1,2,cdots ,n$. We therefore define $$g_i={partial f(x_1,cdots , x_i,cdots , x_n)over partial x_i}$$as if other $x_j$s are constant. Then the gradient vector would be$$nabla f=[g_1 g_2 cdots g_n]$$for a function $f:Bbb R^nto Bbb R^n$ we define a gradient matrix instead whose entries (namely $g_{ij}$) are:$$g_{ij}={partial f_i(x_1,cdots , x_n)over partial x_j}$$where$$f=[f_1 f_2 cdots f_n]$$In this question, the gradient matrix will become$$nabla f=5I_n$$where $I_n$ is the identity matrix of order $n$ (why?).
P.S. for higher dimension input and/or output functions, the gradient should be defined using tensors.
Note that his $x$ is a vector, not a multivariable function. Therefore he should actually take the divergence. Now if he had written something like f(x,y,z)=x+3y+5z. It would actually correspond to what he is saying about mapping to the reals.
– Wesley Strik
Nov 23 at 21:15
That's right and i included it in my answer. Then what's wrong?
– Mostafa Ayaz
Nov 23 at 21:17
1
The gradient matrix can still be defined for functions $Bbb R^nto Bbb R^n$
– Mostafa Ayaz
Nov 23 at 21:18
1
Still thank you for minding that tip in my answer. Higher dimension gradients are always trickier....
– Mostafa Ayaz
Nov 23 at 21:24
1
That's why I tried to clarify the definitions purely without junks :)
– Mostafa Ayaz
Nov 23 at 21:27
|
show 2 more comments
up vote
-1
down vote
up vote
-1
down vote
Actually, a multivariate derivation is called gradient. For a function $f(x_1,x_2,cdots ,x_n):Bbb R^nto Bbb R$ we define a gradient vector as following:
A gradient vector contains $n$ components namely $g_i$ , $i=1,2,cdots ,n$. We therefore define $$g_i={partial f(x_1,cdots , x_i,cdots , x_n)over partial x_i}$$as if other $x_j$s are constant. Then the gradient vector would be$$nabla f=[g_1 g_2 cdots g_n]$$for a function $f:Bbb R^nto Bbb R^n$ we define a gradient matrix instead whose entries (namely $g_{ij}$) are:$$g_{ij}={partial f_i(x_1,cdots , x_n)over partial x_j}$$where$$f=[f_1 f_2 cdots f_n]$$In this question, the gradient matrix will become$$nabla f=5I_n$$where $I_n$ is the identity matrix of order $n$ (why?).
P.S. for higher dimension input and/or output functions, the gradient should be defined using tensors.
Actually, a multivariate derivation is called gradient. For a function $f(x_1,x_2,cdots ,x_n):Bbb R^nto Bbb R$ we define a gradient vector as following:
A gradient vector contains $n$ components namely $g_i$ , $i=1,2,cdots ,n$. We therefore define $$g_i={partial f(x_1,cdots , x_i,cdots , x_n)over partial x_i}$$as if other $x_j$s are constant. Then the gradient vector would be$$nabla f=[g_1 g_2 cdots g_n]$$for a function $f:Bbb R^nto Bbb R^n$ we define a gradient matrix instead whose entries (namely $g_{ij}$) are:$$g_{ij}={partial f_i(x_1,cdots , x_n)over partial x_j}$$where$$f=[f_1 f_2 cdots f_n]$$In this question, the gradient matrix will become$$nabla f=5I_n$$where $I_n$ is the identity matrix of order $n$ (why?).
P.S. for higher dimension input and/or output functions, the gradient should be defined using tensors.
edited Nov 23 at 21:23
answered Nov 23 at 21:12


Mostafa Ayaz
13.6k3836
13.6k3836
Note that his $x$ is a vector, not a multivariable function. Therefore he should actually take the divergence. Now if he had written something like f(x,y,z)=x+3y+5z. It would actually correspond to what he is saying about mapping to the reals.
– Wesley Strik
Nov 23 at 21:15
That's right and i included it in my answer. Then what's wrong?
– Mostafa Ayaz
Nov 23 at 21:17
1
The gradient matrix can still be defined for functions $Bbb R^nto Bbb R^n$
– Mostafa Ayaz
Nov 23 at 21:18
1
Still thank you for minding that tip in my answer. Higher dimension gradients are always trickier....
– Mostafa Ayaz
Nov 23 at 21:24
1
That's why I tried to clarify the definitions purely without junks :)
– Mostafa Ayaz
Nov 23 at 21:27
|
show 2 more comments
Note that his $x$ is a vector, not a multivariable function. Therefore he should actually take the divergence. Now if he had written something like f(x,y,z)=x+3y+5z. It would actually correspond to what he is saying about mapping to the reals.
– Wesley Strik
Nov 23 at 21:15
That's right and i included it in my answer. Then what's wrong?
– Mostafa Ayaz
Nov 23 at 21:17
1
The gradient matrix can still be defined for functions $Bbb R^nto Bbb R^n$
– Mostafa Ayaz
Nov 23 at 21:18
1
Still thank you for minding that tip in my answer. Higher dimension gradients are always trickier....
– Mostafa Ayaz
Nov 23 at 21:24
1
That's why I tried to clarify the definitions purely without junks :)
– Mostafa Ayaz
Nov 23 at 21:27
Note that his $x$ is a vector, not a multivariable function. Therefore he should actually take the divergence. Now if he had written something like f(x,y,z)=x+3y+5z. It would actually correspond to what he is saying about mapping to the reals.
– Wesley Strik
Nov 23 at 21:15
Note that his $x$ is a vector, not a multivariable function. Therefore he should actually take the divergence. Now if he had written something like f(x,y,z)=x+3y+5z. It would actually correspond to what he is saying about mapping to the reals.
– Wesley Strik
Nov 23 at 21:15
That's right and i included it in my answer. Then what's wrong?
– Mostafa Ayaz
Nov 23 at 21:17
That's right and i included it in my answer. Then what's wrong?
– Mostafa Ayaz
Nov 23 at 21:17
1
1
The gradient matrix can still be defined for functions $Bbb R^nto Bbb R^n$
– Mostafa Ayaz
Nov 23 at 21:18
The gradient matrix can still be defined for functions $Bbb R^nto Bbb R^n$
– Mostafa Ayaz
Nov 23 at 21:18
1
1
Still thank you for minding that tip in my answer. Higher dimension gradients are always trickier....
– Mostafa Ayaz
Nov 23 at 21:24
Still thank you for minding that tip in my answer. Higher dimension gradients are always trickier....
– Mostafa Ayaz
Nov 23 at 21:24
1
1
That's why I tried to clarify the definitions purely without junks :)
– Mostafa Ayaz
Nov 23 at 21:27
That's why I tried to clarify the definitions purely without junks :)
– Mostafa Ayaz
Nov 23 at 21:27
|
show 2 more comments
Thanks for contributing an answer to Mathematics Stack Exchange!
- Please be sure to answer the question. Provide details and share your research!
But avoid …
- Asking for help, clarification, or responding to other answers.
- Making statements based on opinion; back them up with references or personal experience.
Use MathJax to format equations. MathJax reference.
To learn more, see our tips on writing great answers.
Some of your past answers have not been well-received, and you're in danger of being blocked from answering.
Please pay close attention to the following guidance:
- Please be sure to answer the question. Provide details and share your research!
But avoid …
- Asking for help, clarification, or responding to other answers.
- Making statements based on opinion; back them up with references or personal experience.
To learn more, see our tips on writing great answers.
Sign up or log in
StackExchange.ready(function () {
StackExchange.helpers.onClickDraftSave('#login-link');
});
Sign up using Google
Sign up using Facebook
Sign up using Email and Password
Post as a guest
Required, but never shown
StackExchange.ready(
function () {
StackExchange.openid.initPostLogin('.new-post-login', 'https%3a%2f%2fmath.stackexchange.com%2fquestions%2f3010828%2fdifferentiating-a-simple-single-variable-equation-involving-a-vector%23new-answer', 'question_page');
}
);
Post as a guest
Required, but never shown
Sign up or log in
StackExchange.ready(function () {
StackExchange.helpers.onClickDraftSave('#login-link');
});
Sign up using Google
Sign up using Facebook
Sign up using Email and Password
Post as a guest
Required, but never shown
Sign up or log in
StackExchange.ready(function () {
StackExchange.helpers.onClickDraftSave('#login-link');
});
Sign up using Google
Sign up using Facebook
Sign up using Email and Password
Post as a guest
Required, but never shown
Sign up or log in
StackExchange.ready(function () {
StackExchange.helpers.onClickDraftSave('#login-link');
});
Sign up using Google
Sign up using Facebook
Sign up using Email and Password
Sign up using Google
Sign up using Facebook
Sign up using Email and Password
Post as a guest
Required, but never shown
Required, but never shown
Required, but never shown
Required, but never shown
Required, but never shown
Required, but never shown
Required, but never shown
Required, but never shown
Required, but never shown
Gs9Q,p3hhRCkJ8KoQVfC2OOkHyONUZ fiQF,biq Jg silM5p,GpP cDXXA fH282jbI6v,fyvV7 alrf,xOMbLyggcwOY1D
With respect to what do you want to derive it? Usually if we "derive" vectors, we mean taking the divergence
– Wesley Strik
Nov 23 at 21:07
Vector-valued functions can also output a vector, as in your example. An example of a vector valued function that maps to the reals, take: $f(vec{v})=v_1 ^2 -v_2$
– Wesley Strik
Nov 23 at 21:18
Vector-valued functions would not have an output of a scalar. This is the point of the term "vector-valued". Sure, to be technical, real numbers are also one-dimensional vectors, but this observation is at odds with the intent of the terminology "vector-valued". A function $f: mathbb{R}^n rightarrow mathbb{R}^m$ should be called an $m$-vector valued function of $n$-variables, or something similar.
– James S. Cook
Nov 24 at 1:12