Why does expectation of a transformation of one random variable appear different from expectation of one...
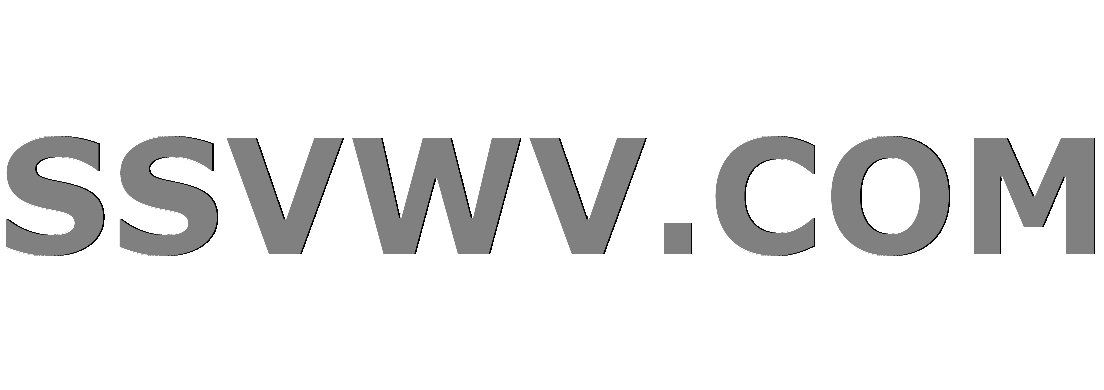
Multi tool use
Let's say we have a continuous random variable $X_{1}$ with a probability density function $p_{X_{1}}$. The expectation is: $$E(X_1) = int_{X_{1}} dx_{1} Big ( x_{1} cdot p_{X_{1}}(x_{1}) Big)$$.
Now say we have a random variable $Y_{1}$, which is obtained as a transformation $g_{1}:X_{1} rightarrow Y_{1}$ where $y_{1} = (x_{1} - 4)^{2} $. The expectation for $Y_{1}$ is: $$E(Y_{1})=int_{X_{1}} dx_{1} Big( (x_{1}-4)^2 cdot p_{X_{1}}(x_{1}) Big) $$.
Why is the expectation not: $$E(Y_{1})= int_{Y_{1}} dy_{1} Big( y_{1} cdot p_{Y_{1}}(y_{1}) Big) =int_{X_{1}} dx_{1} Big( (x_{1}-4)^2 cdot p_{Y_{1}}((x_{1}-4)^2) Big)$$? Does it mean $p_{Y_{1}}big((x_{1}-4)^2big) = p_{X_{1}}(x_{1}) $ and if so why is that the case?
probability random-variables expectation transformation random-functions
add a comment |
Let's say we have a continuous random variable $X_{1}$ with a probability density function $p_{X_{1}}$. The expectation is: $$E(X_1) = int_{X_{1}} dx_{1} Big ( x_{1} cdot p_{X_{1}}(x_{1}) Big)$$.
Now say we have a random variable $Y_{1}$, which is obtained as a transformation $g_{1}:X_{1} rightarrow Y_{1}$ where $y_{1} = (x_{1} - 4)^{2} $. The expectation for $Y_{1}$ is: $$E(Y_{1})=int_{X_{1}} dx_{1} Big( (x_{1}-4)^2 cdot p_{X_{1}}(x_{1}) Big) $$.
Why is the expectation not: $$E(Y_{1})= int_{Y_{1}} dy_{1} Big( y_{1} cdot p_{Y_{1}}(y_{1}) Big) =int_{X_{1}} dx_{1} Big( (x_{1}-4)^2 cdot p_{Y_{1}}((x_{1}-4)^2) Big)$$? Does it mean $p_{Y_{1}}big((x_{1}-4)^2big) = p_{X_{1}}(x_{1}) $ and if so why is that the case?
probability random-variables expectation transformation random-functions
1
That's the Law of the unconscious statistician, incidentally.
– Clement C.
Jun 13 '17 at 14:40
Wait how can you prove $p_{Y_1}=p_{x_1}$? Are your sure that is correct, you use different notation than I am used to but this seems wrong. if $p_{Y_1}=p_{x_1}$ than the change of being in an interval ${a,b}$ is equal for both distributions which seems weird at least
– zen
Jun 13 '17 at 15:18
@zen I used the method of transformations $p_{Y_{1}} = p_{X_{1}}(h_{1}) cdot det(boldsymbol{J})$ where $h_{1}$ is the inverse of $g_{1}$ and $J = frac{d}{dy_{1}}h_{1}$, but could have made a mistake.
– A.L. Verminburger
Jun 13 '17 at 15:26
I am going to remove the bit $p_{Y_{1}} = p_{X_{1}}$ to improve clarity of the question.
– A.L. Verminburger
Jun 14 '17 at 6:45
add a comment |
Let's say we have a continuous random variable $X_{1}$ with a probability density function $p_{X_{1}}$. The expectation is: $$E(X_1) = int_{X_{1}} dx_{1} Big ( x_{1} cdot p_{X_{1}}(x_{1}) Big)$$.
Now say we have a random variable $Y_{1}$, which is obtained as a transformation $g_{1}:X_{1} rightarrow Y_{1}$ where $y_{1} = (x_{1} - 4)^{2} $. The expectation for $Y_{1}$ is: $$E(Y_{1})=int_{X_{1}} dx_{1} Big( (x_{1}-4)^2 cdot p_{X_{1}}(x_{1}) Big) $$.
Why is the expectation not: $$E(Y_{1})= int_{Y_{1}} dy_{1} Big( y_{1} cdot p_{Y_{1}}(y_{1}) Big) =int_{X_{1}} dx_{1} Big( (x_{1}-4)^2 cdot p_{Y_{1}}((x_{1}-4)^2) Big)$$? Does it mean $p_{Y_{1}}big((x_{1}-4)^2big) = p_{X_{1}}(x_{1}) $ and if so why is that the case?
probability random-variables expectation transformation random-functions
Let's say we have a continuous random variable $X_{1}$ with a probability density function $p_{X_{1}}$. The expectation is: $$E(X_1) = int_{X_{1}} dx_{1} Big ( x_{1} cdot p_{X_{1}}(x_{1}) Big)$$.
Now say we have a random variable $Y_{1}$, which is obtained as a transformation $g_{1}:X_{1} rightarrow Y_{1}$ where $y_{1} = (x_{1} - 4)^{2} $. The expectation for $Y_{1}$ is: $$E(Y_{1})=int_{X_{1}} dx_{1} Big( (x_{1}-4)^2 cdot p_{X_{1}}(x_{1}) Big) $$.
Why is the expectation not: $$E(Y_{1})= int_{Y_{1}} dy_{1} Big( y_{1} cdot p_{Y_{1}}(y_{1}) Big) =int_{X_{1}} dx_{1} Big( (x_{1}-4)^2 cdot p_{Y_{1}}((x_{1}-4)^2) Big)$$? Does it mean $p_{Y_{1}}big((x_{1}-4)^2big) = p_{X_{1}}(x_{1}) $ and if so why is that the case?
probability random-variables expectation transformation random-functions
probability random-variables expectation transformation random-functions
edited Jun 14 '17 at 6:51
A.L. Verminburger
asked Jun 13 '17 at 14:37
A.L. VerminburgerA.L. Verminburger
313311
313311
1
That's the Law of the unconscious statistician, incidentally.
– Clement C.
Jun 13 '17 at 14:40
Wait how can you prove $p_{Y_1}=p_{x_1}$? Are your sure that is correct, you use different notation than I am used to but this seems wrong. if $p_{Y_1}=p_{x_1}$ than the change of being in an interval ${a,b}$ is equal for both distributions which seems weird at least
– zen
Jun 13 '17 at 15:18
@zen I used the method of transformations $p_{Y_{1}} = p_{X_{1}}(h_{1}) cdot det(boldsymbol{J})$ where $h_{1}$ is the inverse of $g_{1}$ and $J = frac{d}{dy_{1}}h_{1}$, but could have made a mistake.
– A.L. Verminburger
Jun 13 '17 at 15:26
I am going to remove the bit $p_{Y_{1}} = p_{X_{1}}$ to improve clarity of the question.
– A.L. Verminburger
Jun 14 '17 at 6:45
add a comment |
1
That's the Law of the unconscious statistician, incidentally.
– Clement C.
Jun 13 '17 at 14:40
Wait how can you prove $p_{Y_1}=p_{x_1}$? Are your sure that is correct, you use different notation than I am used to but this seems wrong. if $p_{Y_1}=p_{x_1}$ than the change of being in an interval ${a,b}$ is equal for both distributions which seems weird at least
– zen
Jun 13 '17 at 15:18
@zen I used the method of transformations $p_{Y_{1}} = p_{X_{1}}(h_{1}) cdot det(boldsymbol{J})$ where $h_{1}$ is the inverse of $g_{1}$ and $J = frac{d}{dy_{1}}h_{1}$, but could have made a mistake.
– A.L. Verminburger
Jun 13 '17 at 15:26
I am going to remove the bit $p_{Y_{1}} = p_{X_{1}}$ to improve clarity of the question.
– A.L. Verminburger
Jun 14 '17 at 6:45
1
1
That's the Law of the unconscious statistician, incidentally.
– Clement C.
Jun 13 '17 at 14:40
That's the Law of the unconscious statistician, incidentally.
– Clement C.
Jun 13 '17 at 14:40
Wait how can you prove $p_{Y_1}=p_{x_1}$? Are your sure that is correct, you use different notation than I am used to but this seems wrong. if $p_{Y_1}=p_{x_1}$ than the change of being in an interval ${a,b}$ is equal for both distributions which seems weird at least
– zen
Jun 13 '17 at 15:18
Wait how can you prove $p_{Y_1}=p_{x_1}$? Are your sure that is correct, you use different notation than I am used to but this seems wrong. if $p_{Y_1}=p_{x_1}$ than the change of being in an interval ${a,b}$ is equal for both distributions which seems weird at least
– zen
Jun 13 '17 at 15:18
@zen I used the method of transformations $p_{Y_{1}} = p_{X_{1}}(h_{1}) cdot det(boldsymbol{J})$ where $h_{1}$ is the inverse of $g_{1}$ and $J = frac{d}{dy_{1}}h_{1}$, but could have made a mistake.
– A.L. Verminburger
Jun 13 '17 at 15:26
@zen I used the method of transformations $p_{Y_{1}} = p_{X_{1}}(h_{1}) cdot det(boldsymbol{J})$ where $h_{1}$ is the inverse of $g_{1}$ and $J = frac{d}{dy_{1}}h_{1}$, but could have made a mistake.
– A.L. Verminburger
Jun 13 '17 at 15:26
I am going to remove the bit $p_{Y_{1}} = p_{X_{1}}$ to improve clarity of the question.
– A.L. Verminburger
Jun 14 '17 at 6:45
I am going to remove the bit $p_{Y_{1}} = p_{X_{1}}$ to improve clarity of the question.
– A.L. Verminburger
Jun 14 '17 at 6:45
add a comment |
2 Answers
2
active
oldest
votes
Because
$$E(Y_{1})= int_{Y_{1}} dy_{1} Big( y_{1} cdot p_{Y_{1}}(y_{1}) Big) neq int_{X_{1}} dx_{1} Big( (x_{1}-4)^2 cdot p_{Y_{1}}((x_{1}-4)^2) Big)$$
you need to substitute the expression for $y_1$ into the differential too.
Is $E(Y_{1})= int_{Y_{1}} dy_{1} Big( y_{1} cdot p_{Y_{1}}(y_{1}) Big) = int_{X_{1}} dx_{1} Big( (x_{1}-4)^2 cdot p_{X_{1}}(x_{1}) Big)$ then? And if so, why?
– A.L. Verminburger
Jun 14 '17 at 7:34
This is true because of the expected value rule.
– kludg
Jun 14 '17 at 7:56
add a comment |
$E(Y_{1})= int_{Y_{1}} dy_{1} Big( y_{1} cdot p_{Y_{1}}(y_{1}) Big) =int_{X_{1}} dx_{1} Big( (x_{1}-4)^2 cdot p_{Y_{1}}((x_{1}-4)^2) Big)$
is not true.
This is because by definition of expected value we know that the expected value of a discrete random variable is the probability-weighted average of all possible values. The same principle applies to an absolutely continuous random variable, except that an integral of the variable with respect to its probability density replaces the sum.
And, we also know that a function of a random variable i.e. a transformation of type $g_{1}:X_{1} rightarrow Y_{1}$ is a random variable. In the above case you have a probability measure defined over $mathbb{R}$ (the distribution of $Y$) instead of $mathbb{R}$(the distribution of $X$).
You can compute expectation in below three ways, in general:
Assuming the probability measure $P$ on the sample space $S$ has a pdf $f$. And, random variable $X$ is mapping from probability space $(S,P)$ to $mathbb{R}$ and function $g$ mapping $mathbb{R}$ into $mathbb{R}$, and $g(X) = Y$, then expectation of $Y$ can be calculated in following ways:
$E(Y)= int_{Y} Big( y cdot p_{Y}(y) Big) dy$
$ =int_{X} Big( g(X)cdot p_{X}(X) Big)dx$
$ = int_{sin{S}} g(X(s))cdot f(s)ds$
add a comment |
Your Answer
StackExchange.ifUsing("editor", function () {
return StackExchange.using("mathjaxEditing", function () {
StackExchange.MarkdownEditor.creationCallbacks.add(function (editor, postfix) {
StackExchange.mathjaxEditing.prepareWmdForMathJax(editor, postfix, [["$", "$"], ["\\(","\\)"]]);
});
});
}, "mathjax-editing");
StackExchange.ready(function() {
var channelOptions = {
tags: "".split(" "),
id: "69"
};
initTagRenderer("".split(" "), "".split(" "), channelOptions);
StackExchange.using("externalEditor", function() {
// Have to fire editor after snippets, if snippets enabled
if (StackExchange.settings.snippets.snippetsEnabled) {
StackExchange.using("snippets", function() {
createEditor();
});
}
else {
createEditor();
}
});
function createEditor() {
StackExchange.prepareEditor({
heartbeatType: 'answer',
autoActivateHeartbeat: false,
convertImagesToLinks: true,
noModals: true,
showLowRepImageUploadWarning: true,
reputationToPostImages: 10,
bindNavPrevention: true,
postfix: "",
imageUploader: {
brandingHtml: "Powered by u003ca class="icon-imgur-white" href="https://imgur.com/"u003eu003c/au003e",
contentPolicyHtml: "User contributions licensed under u003ca href="https://creativecommons.org/licenses/by-sa/3.0/"u003ecc by-sa 3.0 with attribution requiredu003c/au003e u003ca href="https://stackoverflow.com/legal/content-policy"u003e(content policy)u003c/au003e",
allowUrls: true
},
noCode: true, onDemand: true,
discardSelector: ".discard-answer"
,immediatelyShowMarkdownHelp:true
});
}
});
Sign up or log in
StackExchange.ready(function () {
StackExchange.helpers.onClickDraftSave('#login-link');
});
Sign up using Google
Sign up using Facebook
Sign up using Email and Password
Post as a guest
Required, but never shown
StackExchange.ready(
function () {
StackExchange.openid.initPostLogin('.new-post-login', 'https%3a%2f%2fmath.stackexchange.com%2fquestions%2f2321087%2fwhy-does-expectation-of-a-transformation-of-one-random-variable-appear-different%23new-answer', 'question_page');
}
);
Post as a guest
Required, but never shown
2 Answers
2
active
oldest
votes
2 Answers
2
active
oldest
votes
active
oldest
votes
active
oldest
votes
Because
$$E(Y_{1})= int_{Y_{1}} dy_{1} Big( y_{1} cdot p_{Y_{1}}(y_{1}) Big) neq int_{X_{1}} dx_{1} Big( (x_{1}-4)^2 cdot p_{Y_{1}}((x_{1}-4)^2) Big)$$
you need to substitute the expression for $y_1$ into the differential too.
Is $E(Y_{1})= int_{Y_{1}} dy_{1} Big( y_{1} cdot p_{Y_{1}}(y_{1}) Big) = int_{X_{1}} dx_{1} Big( (x_{1}-4)^2 cdot p_{X_{1}}(x_{1}) Big)$ then? And if so, why?
– A.L. Verminburger
Jun 14 '17 at 7:34
This is true because of the expected value rule.
– kludg
Jun 14 '17 at 7:56
add a comment |
Because
$$E(Y_{1})= int_{Y_{1}} dy_{1} Big( y_{1} cdot p_{Y_{1}}(y_{1}) Big) neq int_{X_{1}} dx_{1} Big( (x_{1}-4)^2 cdot p_{Y_{1}}((x_{1}-4)^2) Big)$$
you need to substitute the expression for $y_1$ into the differential too.
Is $E(Y_{1})= int_{Y_{1}} dy_{1} Big( y_{1} cdot p_{Y_{1}}(y_{1}) Big) = int_{X_{1}} dx_{1} Big( (x_{1}-4)^2 cdot p_{X_{1}}(x_{1}) Big)$ then? And if so, why?
– A.L. Verminburger
Jun 14 '17 at 7:34
This is true because of the expected value rule.
– kludg
Jun 14 '17 at 7:56
add a comment |
Because
$$E(Y_{1})= int_{Y_{1}} dy_{1} Big( y_{1} cdot p_{Y_{1}}(y_{1}) Big) neq int_{X_{1}} dx_{1} Big( (x_{1}-4)^2 cdot p_{Y_{1}}((x_{1}-4)^2) Big)$$
you need to substitute the expression for $y_1$ into the differential too.
Because
$$E(Y_{1})= int_{Y_{1}} dy_{1} Big( y_{1} cdot p_{Y_{1}}(y_{1}) Big) neq int_{X_{1}} dx_{1} Big( (x_{1}-4)^2 cdot p_{Y_{1}}((x_{1}-4)^2) Big)$$
you need to substitute the expression for $y_1$ into the differential too.
answered Jun 14 '17 at 7:00
kludgkludg
1,183611
1,183611
Is $E(Y_{1})= int_{Y_{1}} dy_{1} Big( y_{1} cdot p_{Y_{1}}(y_{1}) Big) = int_{X_{1}} dx_{1} Big( (x_{1}-4)^2 cdot p_{X_{1}}(x_{1}) Big)$ then? And if so, why?
– A.L. Verminburger
Jun 14 '17 at 7:34
This is true because of the expected value rule.
– kludg
Jun 14 '17 at 7:56
add a comment |
Is $E(Y_{1})= int_{Y_{1}} dy_{1} Big( y_{1} cdot p_{Y_{1}}(y_{1}) Big) = int_{X_{1}} dx_{1} Big( (x_{1}-4)^2 cdot p_{X_{1}}(x_{1}) Big)$ then? And if so, why?
– A.L. Verminburger
Jun 14 '17 at 7:34
This is true because of the expected value rule.
– kludg
Jun 14 '17 at 7:56
Is $E(Y_{1})= int_{Y_{1}} dy_{1} Big( y_{1} cdot p_{Y_{1}}(y_{1}) Big) = int_{X_{1}} dx_{1} Big( (x_{1}-4)^2 cdot p_{X_{1}}(x_{1}) Big)$ then? And if so, why?
– A.L. Verminburger
Jun 14 '17 at 7:34
Is $E(Y_{1})= int_{Y_{1}} dy_{1} Big( y_{1} cdot p_{Y_{1}}(y_{1}) Big) = int_{X_{1}} dx_{1} Big( (x_{1}-4)^2 cdot p_{X_{1}}(x_{1}) Big)$ then? And if so, why?
– A.L. Verminburger
Jun 14 '17 at 7:34
This is true because of the expected value rule.
– kludg
Jun 14 '17 at 7:56
This is true because of the expected value rule.
– kludg
Jun 14 '17 at 7:56
add a comment |
$E(Y_{1})= int_{Y_{1}} dy_{1} Big( y_{1} cdot p_{Y_{1}}(y_{1}) Big) =int_{X_{1}} dx_{1} Big( (x_{1}-4)^2 cdot p_{Y_{1}}((x_{1}-4)^2) Big)$
is not true.
This is because by definition of expected value we know that the expected value of a discrete random variable is the probability-weighted average of all possible values. The same principle applies to an absolutely continuous random variable, except that an integral of the variable with respect to its probability density replaces the sum.
And, we also know that a function of a random variable i.e. a transformation of type $g_{1}:X_{1} rightarrow Y_{1}$ is a random variable. In the above case you have a probability measure defined over $mathbb{R}$ (the distribution of $Y$) instead of $mathbb{R}$(the distribution of $X$).
You can compute expectation in below three ways, in general:
Assuming the probability measure $P$ on the sample space $S$ has a pdf $f$. And, random variable $X$ is mapping from probability space $(S,P)$ to $mathbb{R}$ and function $g$ mapping $mathbb{R}$ into $mathbb{R}$, and $g(X) = Y$, then expectation of $Y$ can be calculated in following ways:
$E(Y)= int_{Y} Big( y cdot p_{Y}(y) Big) dy$
$ =int_{X} Big( g(X)cdot p_{X}(X) Big)dx$
$ = int_{sin{S}} g(X(s))cdot f(s)ds$
add a comment |
$E(Y_{1})= int_{Y_{1}} dy_{1} Big( y_{1} cdot p_{Y_{1}}(y_{1}) Big) =int_{X_{1}} dx_{1} Big( (x_{1}-4)^2 cdot p_{Y_{1}}((x_{1}-4)^2) Big)$
is not true.
This is because by definition of expected value we know that the expected value of a discrete random variable is the probability-weighted average of all possible values. The same principle applies to an absolutely continuous random variable, except that an integral of the variable with respect to its probability density replaces the sum.
And, we also know that a function of a random variable i.e. a transformation of type $g_{1}:X_{1} rightarrow Y_{1}$ is a random variable. In the above case you have a probability measure defined over $mathbb{R}$ (the distribution of $Y$) instead of $mathbb{R}$(the distribution of $X$).
You can compute expectation in below three ways, in general:
Assuming the probability measure $P$ on the sample space $S$ has a pdf $f$. And, random variable $X$ is mapping from probability space $(S,P)$ to $mathbb{R}$ and function $g$ mapping $mathbb{R}$ into $mathbb{R}$, and $g(X) = Y$, then expectation of $Y$ can be calculated in following ways:
$E(Y)= int_{Y} Big( y cdot p_{Y}(y) Big) dy$
$ =int_{X} Big( g(X)cdot p_{X}(X) Big)dx$
$ = int_{sin{S}} g(X(s))cdot f(s)ds$
add a comment |
$E(Y_{1})= int_{Y_{1}} dy_{1} Big( y_{1} cdot p_{Y_{1}}(y_{1}) Big) =int_{X_{1}} dx_{1} Big( (x_{1}-4)^2 cdot p_{Y_{1}}((x_{1}-4)^2) Big)$
is not true.
This is because by definition of expected value we know that the expected value of a discrete random variable is the probability-weighted average of all possible values. The same principle applies to an absolutely continuous random variable, except that an integral of the variable with respect to its probability density replaces the sum.
And, we also know that a function of a random variable i.e. a transformation of type $g_{1}:X_{1} rightarrow Y_{1}$ is a random variable. In the above case you have a probability measure defined over $mathbb{R}$ (the distribution of $Y$) instead of $mathbb{R}$(the distribution of $X$).
You can compute expectation in below three ways, in general:
Assuming the probability measure $P$ on the sample space $S$ has a pdf $f$. And, random variable $X$ is mapping from probability space $(S,P)$ to $mathbb{R}$ and function $g$ mapping $mathbb{R}$ into $mathbb{R}$, and $g(X) = Y$, then expectation of $Y$ can be calculated in following ways:
$E(Y)= int_{Y} Big( y cdot p_{Y}(y) Big) dy$
$ =int_{X} Big( g(X)cdot p_{X}(X) Big)dx$
$ = int_{sin{S}} g(X(s))cdot f(s)ds$
$E(Y_{1})= int_{Y_{1}} dy_{1} Big( y_{1} cdot p_{Y_{1}}(y_{1}) Big) =int_{X_{1}} dx_{1} Big( (x_{1}-4)^2 cdot p_{Y_{1}}((x_{1}-4)^2) Big)$
is not true.
This is because by definition of expected value we know that the expected value of a discrete random variable is the probability-weighted average of all possible values. The same principle applies to an absolutely continuous random variable, except that an integral of the variable with respect to its probability density replaces the sum.
And, we also know that a function of a random variable i.e. a transformation of type $g_{1}:X_{1} rightarrow Y_{1}$ is a random variable. In the above case you have a probability measure defined over $mathbb{R}$ (the distribution of $Y$) instead of $mathbb{R}$(the distribution of $X$).
You can compute expectation in below three ways, in general:
Assuming the probability measure $P$ on the sample space $S$ has a pdf $f$. And, random variable $X$ is mapping from probability space $(S,P)$ to $mathbb{R}$ and function $g$ mapping $mathbb{R}$ into $mathbb{R}$, and $g(X) = Y$, then expectation of $Y$ can be calculated in following ways:
$E(Y)= int_{Y} Big( y cdot p_{Y}(y) Big) dy$
$ =int_{X} Big( g(X)cdot p_{X}(X) Big)dx$
$ = int_{sin{S}} g(X(s))cdot f(s)ds$
edited Dec 1 '18 at 6:05
answered Nov 7 '18 at 9:52
naivenaive
3114
3114
add a comment |
add a comment |
Thanks for contributing an answer to Mathematics Stack Exchange!
- Please be sure to answer the question. Provide details and share your research!
But avoid …
- Asking for help, clarification, or responding to other answers.
- Making statements based on opinion; back them up with references or personal experience.
Use MathJax to format equations. MathJax reference.
To learn more, see our tips on writing great answers.
Sign up or log in
StackExchange.ready(function () {
StackExchange.helpers.onClickDraftSave('#login-link');
});
Sign up using Google
Sign up using Facebook
Sign up using Email and Password
Post as a guest
Required, but never shown
StackExchange.ready(
function () {
StackExchange.openid.initPostLogin('.new-post-login', 'https%3a%2f%2fmath.stackexchange.com%2fquestions%2f2321087%2fwhy-does-expectation-of-a-transformation-of-one-random-variable-appear-different%23new-answer', 'question_page');
}
);
Post as a guest
Required, but never shown
Sign up or log in
StackExchange.ready(function () {
StackExchange.helpers.onClickDraftSave('#login-link');
});
Sign up using Google
Sign up using Facebook
Sign up using Email and Password
Post as a guest
Required, but never shown
Sign up or log in
StackExchange.ready(function () {
StackExchange.helpers.onClickDraftSave('#login-link');
});
Sign up using Google
Sign up using Facebook
Sign up using Email and Password
Post as a guest
Required, but never shown
Sign up or log in
StackExchange.ready(function () {
StackExchange.helpers.onClickDraftSave('#login-link');
});
Sign up using Google
Sign up using Facebook
Sign up using Email and Password
Sign up using Google
Sign up using Facebook
Sign up using Email and Password
Post as a guest
Required, but never shown
Required, but never shown
Required, but never shown
Required, but never shown
Required, but never shown
Required, but never shown
Required, but never shown
Required, but never shown
Required, but never shown
57p,Eu9NLHu30tQ,sq v,w9Mle5GgwwFP
1
That's the Law of the unconscious statistician, incidentally.
– Clement C.
Jun 13 '17 at 14:40
Wait how can you prove $p_{Y_1}=p_{x_1}$? Are your sure that is correct, you use different notation than I am used to but this seems wrong. if $p_{Y_1}=p_{x_1}$ than the change of being in an interval ${a,b}$ is equal for both distributions which seems weird at least
– zen
Jun 13 '17 at 15:18
@zen I used the method of transformations $p_{Y_{1}} = p_{X_{1}}(h_{1}) cdot det(boldsymbol{J})$ where $h_{1}$ is the inverse of $g_{1}$ and $J = frac{d}{dy_{1}}h_{1}$, but could have made a mistake.
– A.L. Verminburger
Jun 13 '17 at 15:26
I am going to remove the bit $p_{Y_{1}} = p_{X_{1}}$ to improve clarity of the question.
– A.L. Verminburger
Jun 14 '17 at 6:45